Sensitivity analysis of distributed photovoltaic system capacity estimation based on artificial neural network
Sustainable Energy, Grids and Networks(2024)
摘要
Residential solar photovoltaic (PV) system installations are expected to continue increasing due to their growing cost competitiveness and supportive government policies. However, excessive installations of unknown behind-the-meter solar panels present a challenge for accurate load prediction and reliable operations of power networks. To address such growing concerns of distribution network operators (DNOs), this research proposes a novel model for distributed PV system capacity estimations. Innovative extracted features from 24-hour substation net load curves were fed into a deep neural network to estimate the PV capacity linked to the substation feeder. A comprehensive study into the sensitivity of the model’s accuracy to specific temporal scales of data collection, number of households served by a substation, and proportion of PV-equipped properties was conducted. This study revealed that a model developed to be used exclusively in summer achieved a 18.1% decrease in estimation root mean squared error (RMSE) compared to an all-year model, whilst using only a third of the training data amount. Similarly, compared to an all-year model, RMSE decreased by 26.9% when only data from Mondays to Thursdays were used to train and test the model. Also, for the all-year model, the most accurate estimations occur when 20% to 80% of households have PV systems installed and estimation percentage error tend to remain constant at around 10% when more than 20% of households have PV systems installed. A machine learning-ready dataset of substations with known PV capacity and experiment results are both useful to inform DNOs on the potential of the proposed method in reducing grid operation costs.
更多查看译文
关键词
Data analytics,Feature extraction,Feeder load data,Machine learning,Solar photovoltaic,Capacity estimation
AI 理解论文
溯源树
样例
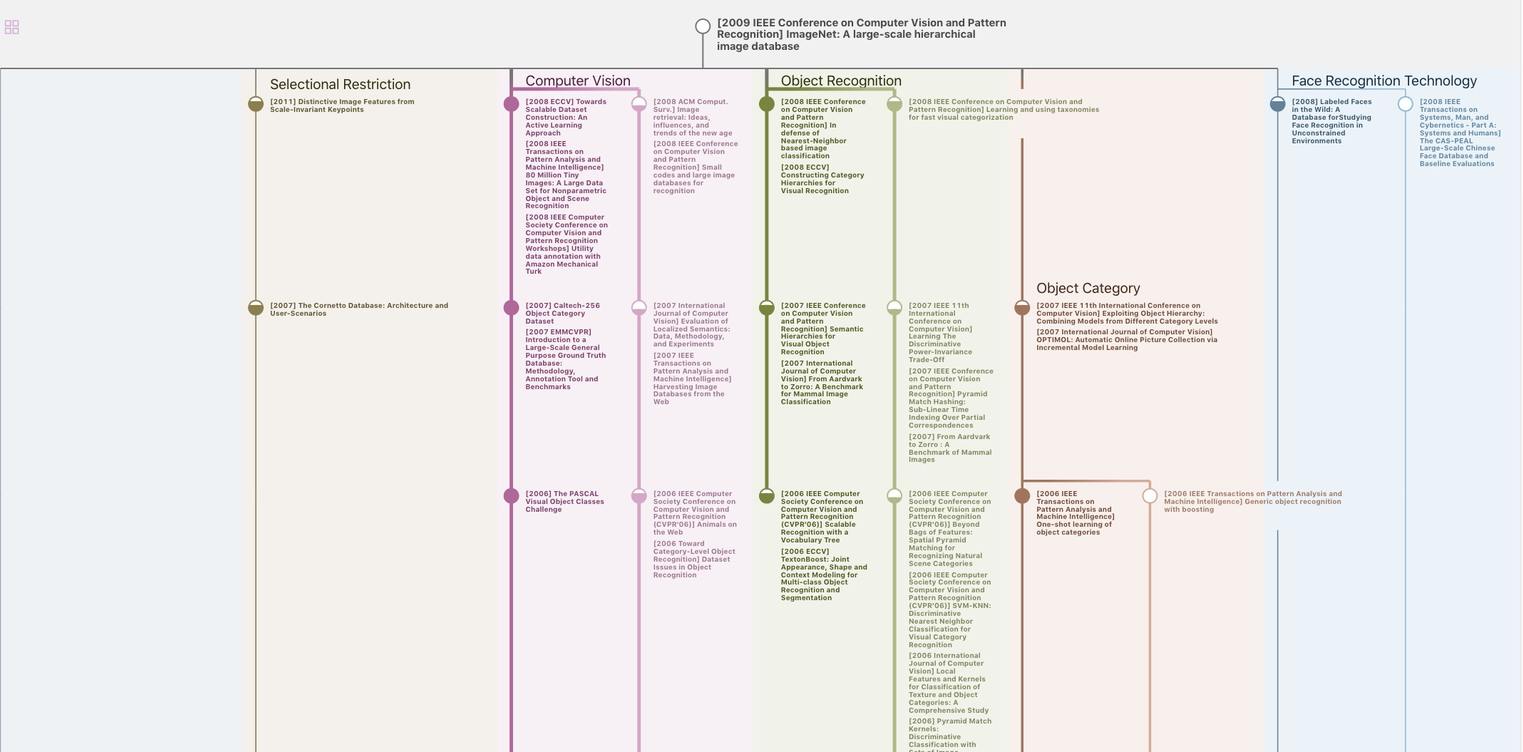
生成溯源树,研究论文发展脉络
Chat Paper
正在生成论文摘要