Motif Masking-based Self-Supervised Learning For Molecule Graph Representation Learning.
2023 IEEE International Conference on e-Business Engineering (ICEBE)(2023)
摘要
Molecule graph representation is an emergent technique for chemistry analysis. Integrating it with online drug sales can deeply understand the properties of drugs and realize personalized recommendations based on molecular information, which is of great significance in the intelligent analysis system and the improvement of user experience. The latest research works apply the mask-based model to represent the molecule graph and achieve great success in molecule graph representation. However, Existing mask-based molecular graph representation methods can only randomly mask single nodes, and cannot mask key functional groups in the molecular graph as a whole. This causes the mutual information of the key features of the molecule to be lost, limiting the further improvement of the performance of the molecular graph representation. To cope with this challenge, we propose a novel molecular graph representation method that uses motif vocabulary to mask critical functional groups to complement the defining mutual information of the molecular graph. First, the functional groups are discovered by motif vocabulary. Then, the discovered functional groups are masked randomly from the original graph. After, our method effectively learns a molecular graph encoder, which is enhanced by more discriminative node-node and graph-graph cross information. At last, massive experiments show that the graph representation information output by our method can be better used for downstream molecular graph classification tasks. The implementation is publicly available at https://gitee.com/wu-yasu/mmssl.git
更多查看译文
关键词
Deep Learning,Graph Representation Learning,Molecule Graph Analysis,E-Analysis
AI 理解论文
溯源树
样例
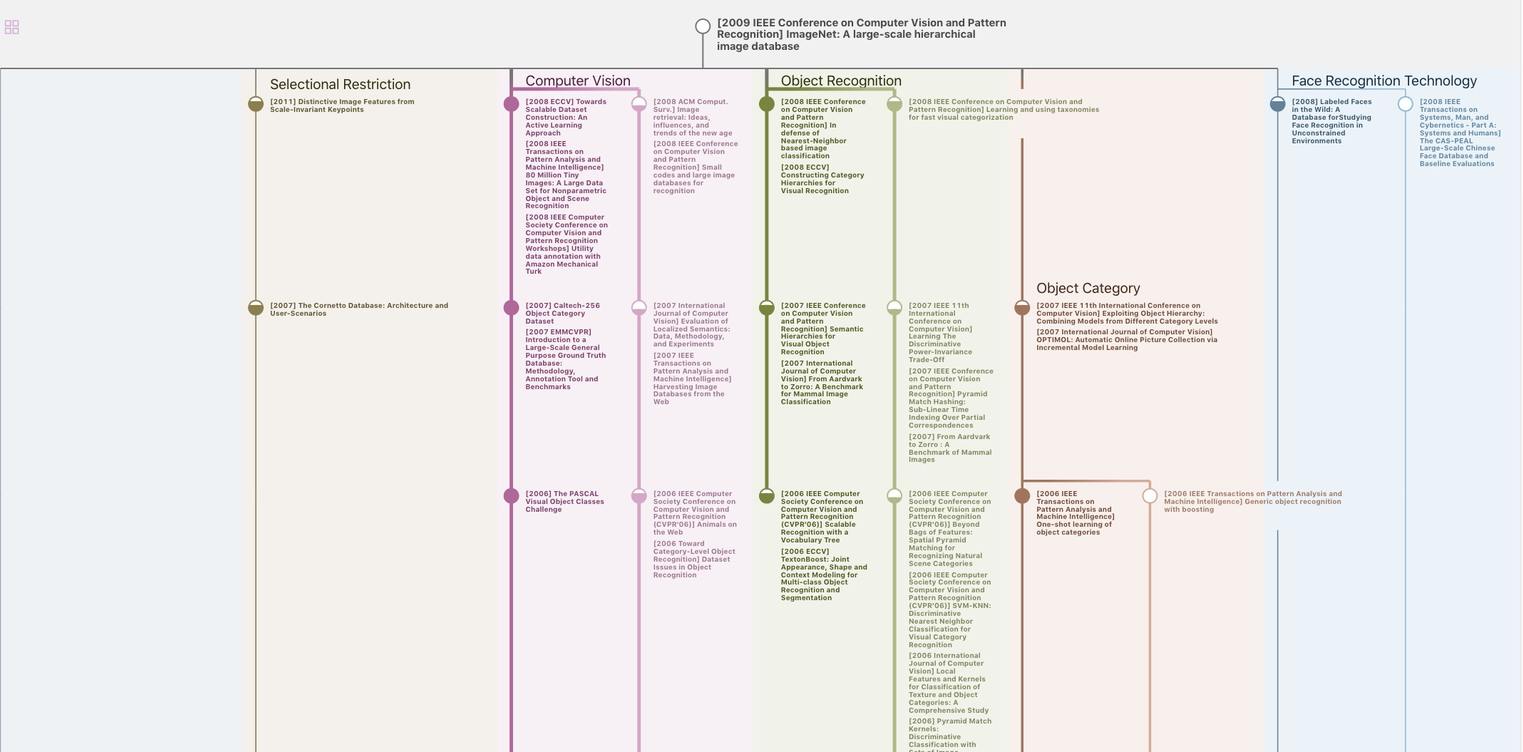
生成溯源树,研究论文发展脉络
Chat Paper
正在生成论文摘要