Prediction of Adolescent Depression Relapse Events Using Fusion of Actigraphy and Ecological Momentary Assessment Features
2023 IEEE 5th International Conference on Cybernetics, Cognition and Machine Learning Applications (ICCCMLA)(2023)
摘要
The objective of this study is to explore the application of machine learning (ML) for the prediction of relapse events in adolescents suffering from Major Depressive Disorder using actigraphy and ecological momentary assessment (EMA) data. The data were collected from 114 adolescents aged between 12 and 21 who were participating in a depression research study at the Centre for Addiction and Mental Health. They made up to 8 visits where a psychiatrist would assess their level of depression by conducting a Children's Depression Rating Scale (CDRS) survey. Between visits, participants wore the GeneActiv wearable device to provide actigraphy data and also answered survey questions to provide EMA data. Any subject that experienced one visit with a CDRS score of less than 40 followed by another visit with a score of greater than 40 was labelled as undergoing a relapse event. Various ML methods including support vector machines, random forests, and neural networks in combination with fusion methods were used to predict relapse events. After training the above methods on 67% of the participants and testing them on the remaining ones, it was determined that feature fusion techniques applied to actigraphy and EMA metrics produced the highest area under the receiver operating characteristic curve of 0.72. Another novel technique combining raw actigraphy counts with the aforementioned metrics yielded areas of up to 0.65. Overall, the results show that there is much promise that can be held in the integration of digital phenotypes for the prediction of relapse events in adolescents.
更多查看译文
关键词
machine learning,neural networks,actigraphy,ecological momentary assessment,fusion methods,adolescent depression,relapse
AI 理解论文
溯源树
样例
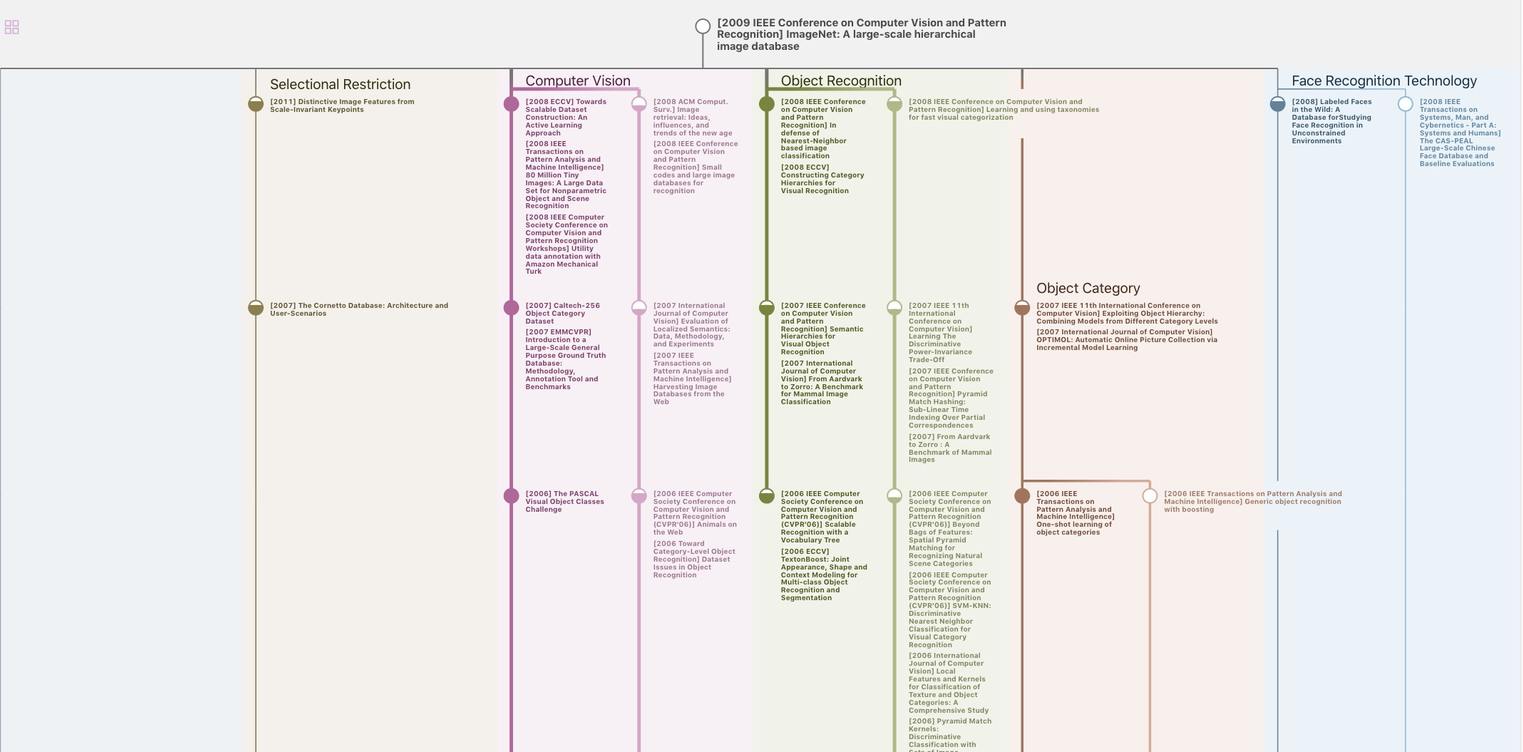
生成溯源树,研究论文发展脉络
Chat Paper
正在生成论文摘要