Explainability Analysis of Weather Variables in Short- Term Load Forecasting.
2023 14th International Conference on Information, Intelligence, Systems & Applications (IISA)(2023)
摘要
Short-term load forecasting tasks utilize a plethora of weather sequences in order to study meaningful features that describe complex relationships between environmental parameters and the variable of load as well as the occurrence of impactful events, reinforcing the predictive power of estimators. It is evident that the inclusion of those additional features increases the input dimensions and decreases the overall explain ability of forecasting models since not all of the extracted features are equally compatible with the assumptions of the regressors, resulting in models where the justification of predictive potency, the connection between the variance of the target variable and the variance of predictors as well as the explanation of the computational flow are made increasingly difficult due to ambiguity. Therefore, this research work studies the representativeness of weather variables and examines the explain ability of interpretable short-term load forecasting structures that utilize environmental sequences towards the selection of important feature subsets that maximize the explained variance of load time series as well as the seasonal, trend and residual load components during training. This project considers linear regression and prominent tree-based estimators coupled with model-dependent feature importance frameworks utilizing game theoretic, permutative and impurity-based approaches for the derivation of optimal feature sets and feature set combinations in terms of explained variance scores. Additionally, two structural approaches of explainable feature set generation from the joint feature importance scores through the utilization of hierarchical and maj ority inclusion are compared and the main observations from each stage of this analysis are discussed.
更多查看译文
关键词
explainability,load forecasting,intelligent data analytics,machine learning,feature engineering,knowledge extraction
AI 理解论文
溯源树
样例
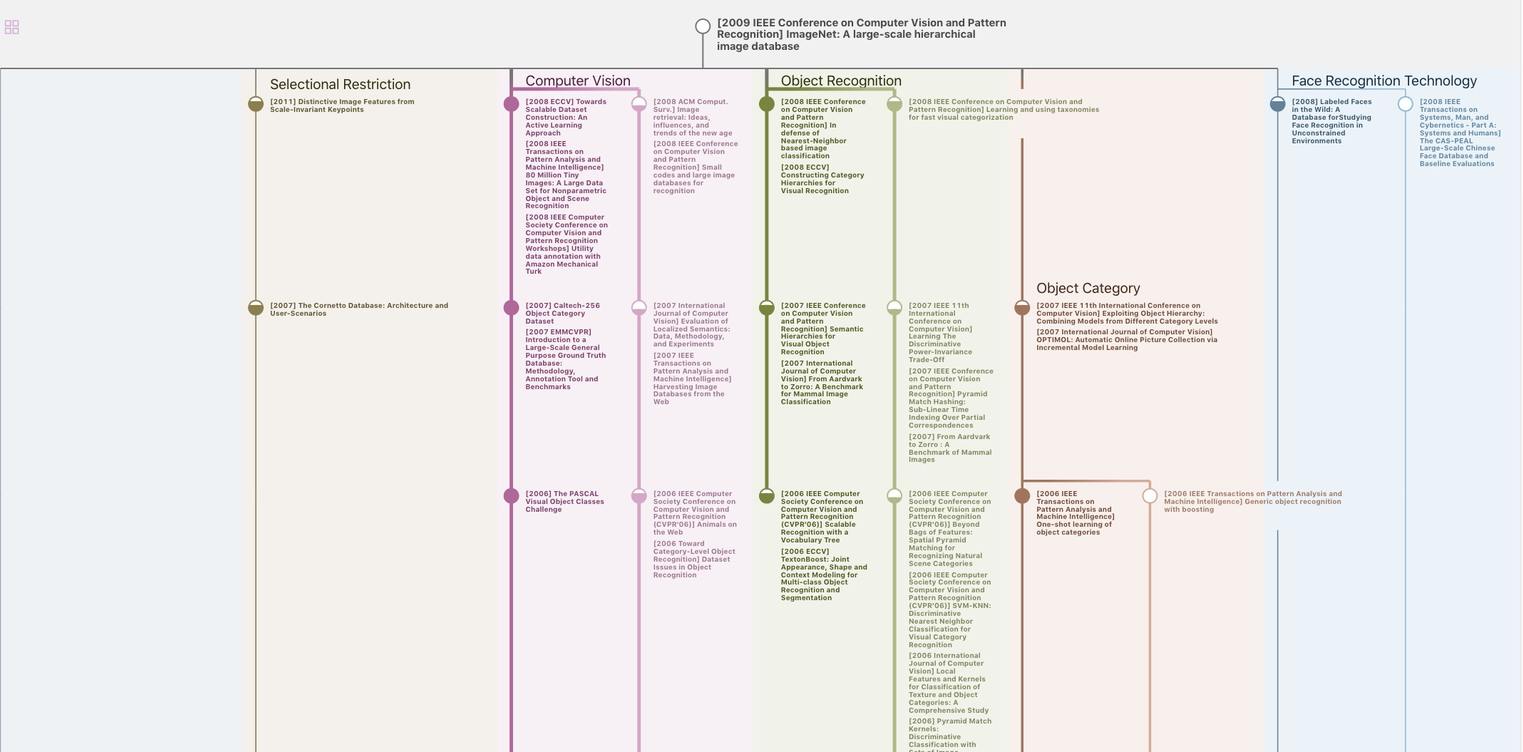
生成溯源树,研究论文发展脉络
Chat Paper
正在生成论文摘要