Seeing both sides: context-aware heterogeneous graph matching networks for extracting-related arguments
Neural Computing and Applications(2024)
摘要
Our research focuses on extracting exchanged views from dialogical documents through argument pair extraction (APE). The objective of this process is to facilitate comprehension of complex argumentative discourse by finding the related arguments. The APE comprises two stages: argument mining and argument matching. Researchers typically employ sequence labeling models for mining arguments and text matching models to calculate the relationships between them, thereby generating argument pairs. However, these approaches fail to capture long-distance contextual information and struggle to fully comprehend the complex structure of arguments. In our work, we propose the context-aware heterogeneous graph matching (HGMN) model for the APE task. First, we design a graph schema specifically tailored to argumentative texts, along with a heterogeneous graph attention network that effectively captures context information and structural information of arguments. Moreover, the text matching between arguments is converted into a graph matching paradigm and a multi-granularity graph matching model is proposed to handle the intricate relationships between arguments at various levels of granularity. In this way, the semantics of argument are modeled structurally and thus capture the complicated correlations between arguments. Extensive experiments are conducted to evaluate the HGMN model, including comparisons with existing methods and the GPT series of large language models (LLM). The results demonstrate that HGMN outperforms the state-of-the-art method.
更多查看译文
关键词
Natural language process,Argument mining,Argument pair extraction,Graph matching networks,Heterogeneous graph neural networks
AI 理解论文
溯源树
样例
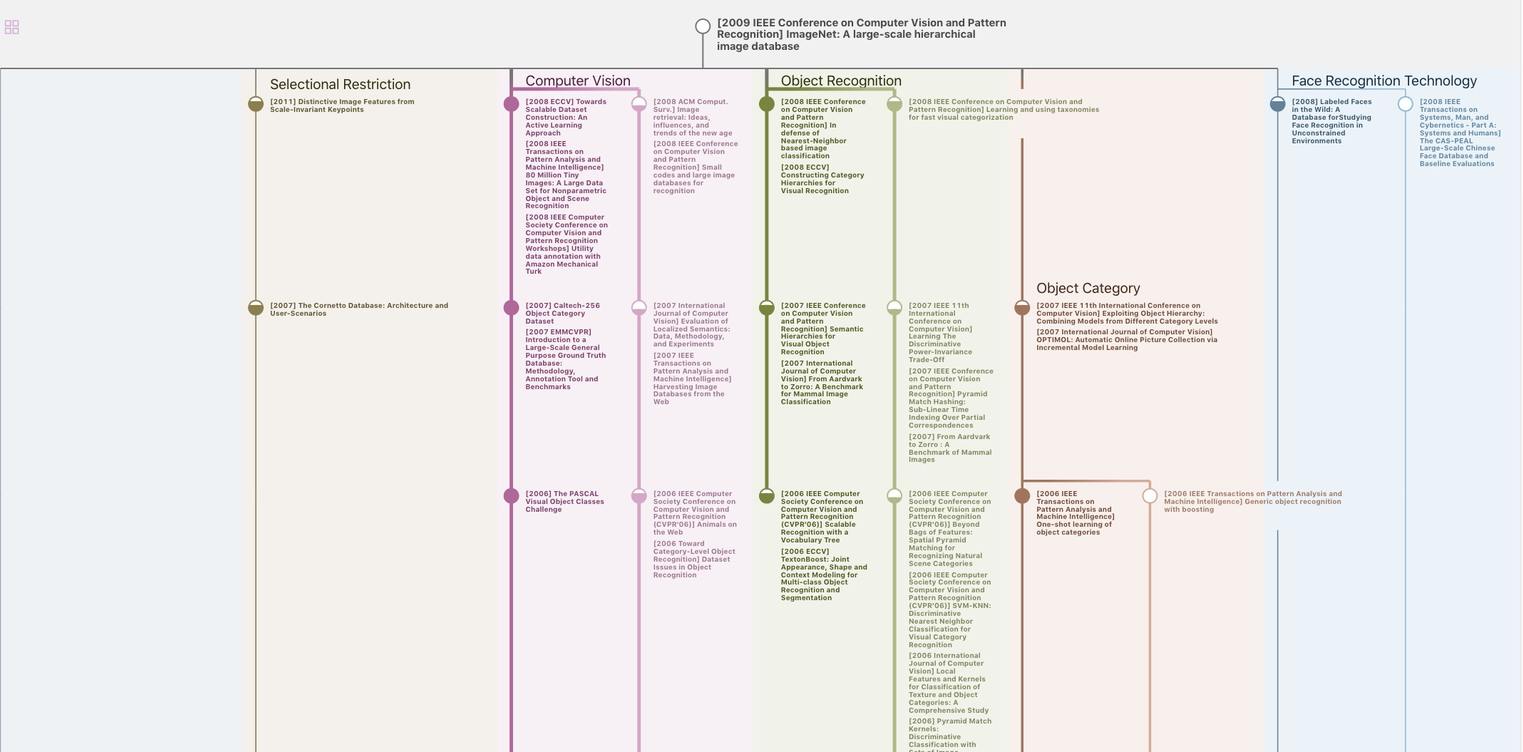
生成溯源树,研究论文发展脉络
Chat Paper
正在生成论文摘要