Debiased graph contrastive learning based on positive and unlabeled learning
International Journal of Machine Learning and Cybernetics(2023)
摘要
Graph contrastive learning (GCL) is one of the mainstream techniques for unsupervised graph representation learning, which reduces the distance between positive pairs and increases the distance between negative pairs in the embedding space to obtain discriminative representation. However, most existing GCL methods mainly focus on graph augmentation for positive samples, while the effect of negative samples is less explored, and they regard all samples except anchors as negative samples, which may push away latent positive samples that belong to the same class as the anchor, thus introducing false negative samples. To this end, this paper proposes a novel framework called Debiased Graph Contrastive Learning Based on Positive and Unlabeled Learning (DGCL-PU). Firstly, in this framework, we cluster the nodes by using the K-means algorithm and then treat the samples that are the same as the anchor as positive samples and the others as unlabelled samples. In this way, PU learning can be applied to assign scores for samples to determine the propensity of becoming negative samples relative to the anchor point. Secondly, we integrate the similarity between samples and the negative propensity score of samples, thereby obtaining reasonable weights for negative samples. Finally, the weighted graph contrastive loss is designed to obtain more discriminative feature representations and alleviate the bias of false negative samples. Moreover, DGCL-PU is a general framework, and it can be embedded into most existing GCL methods to improve their performance. Experiments on multiple benchmark datasets demonstrate that our method achieves state-of-the-art performance on multiple downstream tasks, including graph node classification, node clustering, and link prediction.
更多查看译文
关键词
Graph contrastive learning,Positive and unlabeled learning,False negative samples,Graph representation learning
AI 理解论文
溯源树
样例
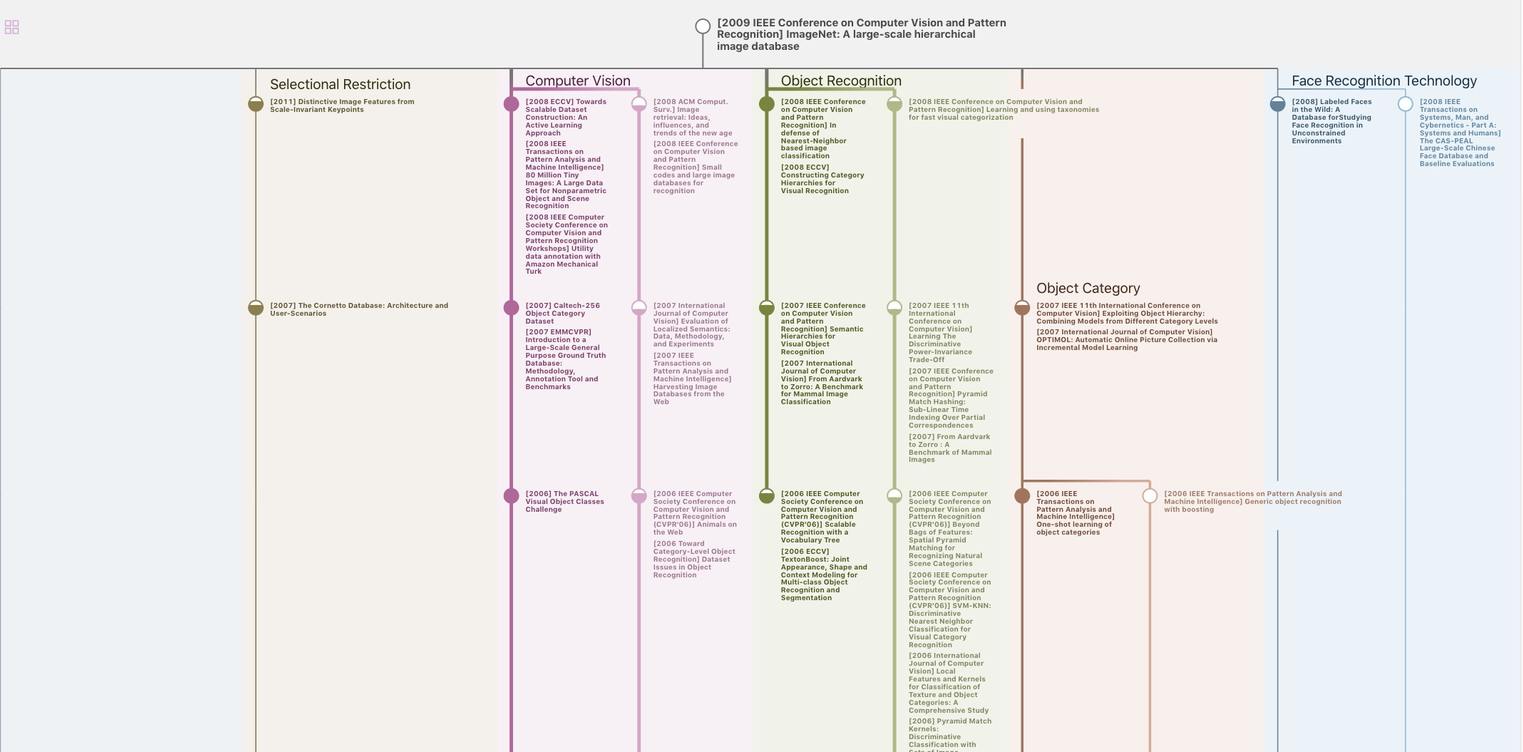
生成溯源树,研究论文发展脉络
Chat Paper
正在生成论文摘要