ORB-Net: End-to-end Planning Using Feature-based Imitation Learning for Autonomous Drone Racing
ISR Europe 2023; 56th International Symposium on Robotics(2023)
摘要
Deep learning-based methods have enhanced the performance of many robot applications thanks to their superior ability to robustly extract rich high-dimensional features. However, it comes with a high computational cost that often increases the latency of the overall system. On the other hand, traditional feature extraction methods, such as oriented FAST and rotated BRIEF (ORB), can be computed efficiently and remains the backbone of some critical robot algorithms, e.g. ORBSLAM for robot state estimation. In this work, the usefulness of the aforementioned features for a robot navigation task is investigated. The features are experimentally incorporated with a deep-learning method, called ORB-Net, which allows an agile aerial robot to learn a motion policy to complete a racing track in an autonomous drone racing context. The experimental studies demonstrate that it can be beneficial to reuse these computed features for end-to-end motion planning for the agile quadrotor, as the proposed method using combined input of ORB feature position and RGB images outperforms the baseline methods which use only RGB images.
更多查看译文
AI 理解论文
溯源树
样例
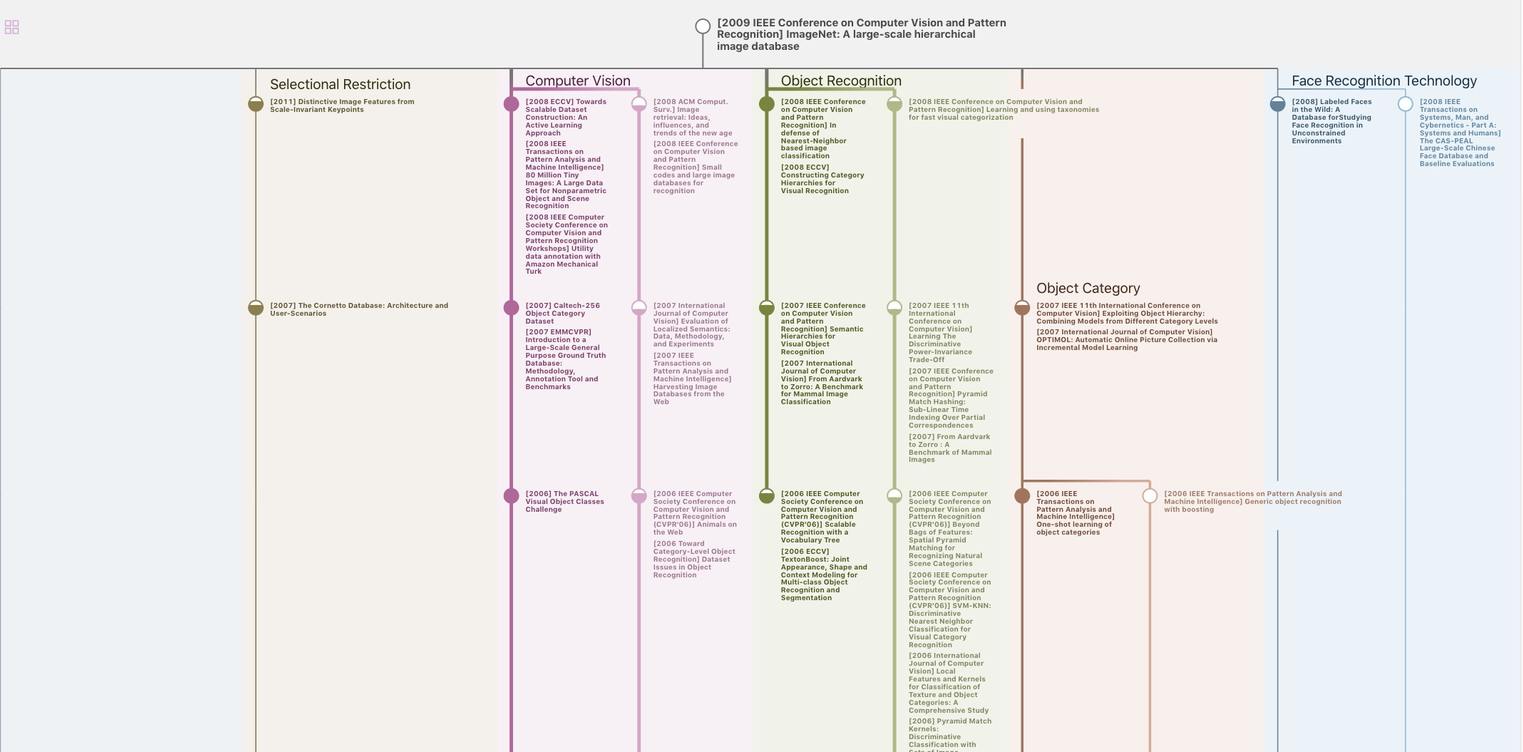
生成溯源树,研究论文发展脉络
Chat Paper
正在生成论文摘要