Anomalous Behavior Detection for Cloud Infrastructures via Transformer-represented Prior Knowledge.
2023 IEEE 8th International Conference on Smart Cloud (SmartCloud)(2023)
摘要
With the rapid progression and increasing industrial relevance of cloud computing, the stability of cloud systems has become increasingly critical as they are handling a vast number of requests. To ensure this stability, the operational status of cloud servers, indicated by key performance indicators such as CPU load, network bandwidth, memory allocation, and disk 1/0 operations, must be continuously monitored. Any changes in these metrics could signify potential system malfunctions like network intrusion or hardware faults due to user activities. However, given the cyclical nature of the utilization metrics, which often exhibit, minute, hour, or daily patterns, merely setting thresholds may not effectively detect these anomalous behaviors. To address this, our research capitalizes on the advantages of the Transformer model, incorporating cyclical prior knowledge to extract these periodic patterns from the metrics, thereby enhancing the detection efficacy. Experimental results show that our proposed approach outperforms the Transformer model, improving the anomalous detection accuracy by 1.7 %, with the comprehensive evaluation metric (Fl score) surpassing 0.88.
更多查看译文
关键词
Cloud computing,Utilization metrics,Anomalous detection,Transformer,Prior Knowledge
AI 理解论文
溯源树
样例
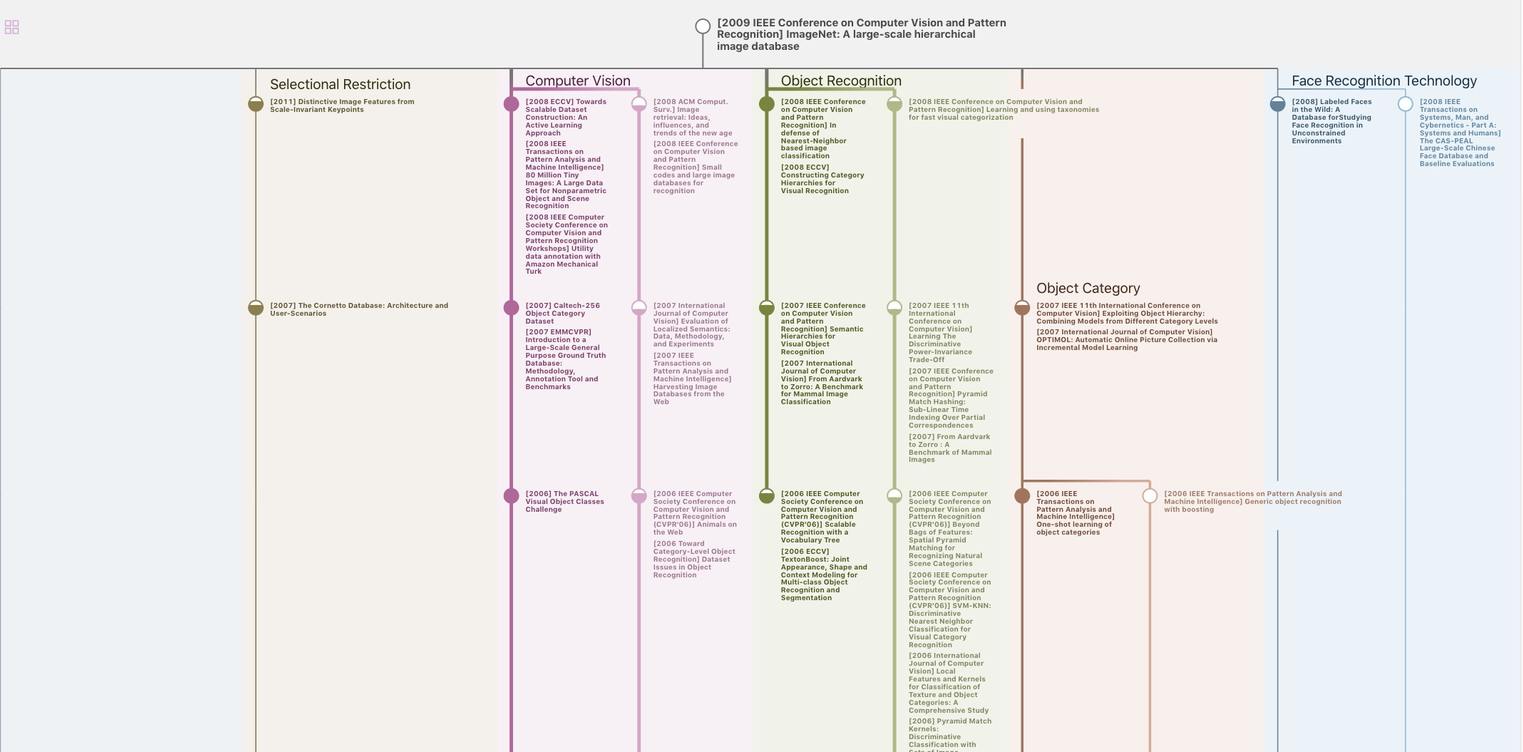
生成溯源树,研究论文发展脉络
Chat Paper
正在生成论文摘要