Statistical significance maps based on machine learning. On the use of data-dependent upper bounds
2023 8th International Conference on Information and Network Technologies (ICINT)(2023)
摘要
The analysis of neuroimaging data has become a standard in the clinical practice and is frequently used to corroborate the diagnosis and monitor the evolution of neurological disorders. Over the last decades, the analysis of these data has evolved from visual inspection to computer analysis, with machine learning based approaches being particularly common in recent years. These models are particularly useful for classifying data but their ability to extract regions of interest from the comparison of two populations has not yet been adequately explored.In this paper, we demonstrate a tool to build significance maps from medical brain imaging. These maps contain the regions of interest for the comparison of two given groups and are built using the accuracy rate of a statistical classifier trained to separate these groups. In contrast to previous works, the significance level of each region is calculated from corrected accuracy rates, which are computed by considering the upper bound for the classification error (worst case). This makes the presented approach very conservative and reinforces the importance of the reported regions. In addition, we propose a methodology to calculate data-adapted upper bounds based on the Bayesian theory.
更多查看译文
关键词
machine learning,statistical significance,neuroimaging,Matlab toolbox
AI 理解论文
溯源树
样例
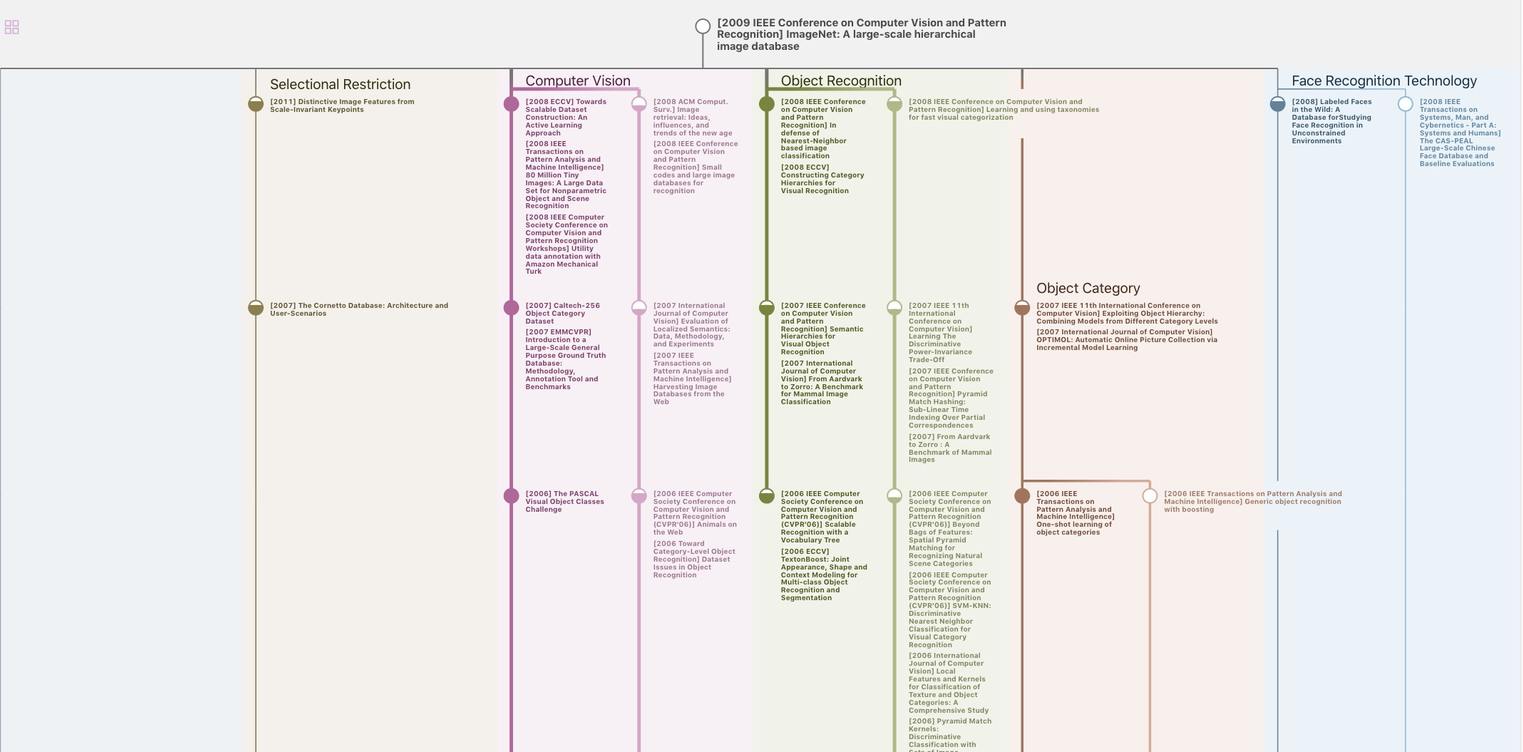
生成溯源树,研究论文发展脉络
Chat Paper
正在生成论文摘要