A novel diffusion recommendation algorithm based on multi-scale cnn and residual lstm
arxiv(2023)
摘要
Sequential recommendation aims to infer user preferences from historical interaction sequences and predict the next item that users may be interested in the future. The current mainstream design approach is to represent items as fixed vectors, capturing the underlying relationships between items and user preferences based on the order of interactions. However, relying on a single fixed-item embedding may weaken the modeling capability of the system, and the global dynamics and local saliency exhibited by user preferences need to be distinguished. To address these issues, this paper proposes a novel diffusion recommendation algorithm based on multi-scale cnn and residual lstm (AREAL). We introduce diffusion models into the recommend system, representing items as probability distributions instead of fixed vectors. This approach enables adaptive reflection of multiple aspects of the items and generates item distributions in a denoising manner. We use multi-scale cnn and residual lstm methods to extract the local and global dependency features of user history interactions, and use attention mechanism to distinguish weights as the guide features of reverse diffusion recovery. The effectiveness of the proposed method is validated through experiments conducted on two real-world datasets. Specifically, AREAL obtains improvements over the best baselines by 2.63% and 4.25% in terms of HR@20 and 5.05% and 3.94% in terms of NDCG@20 on all datasets.
更多查看译文
AI 理解论文
溯源树
样例
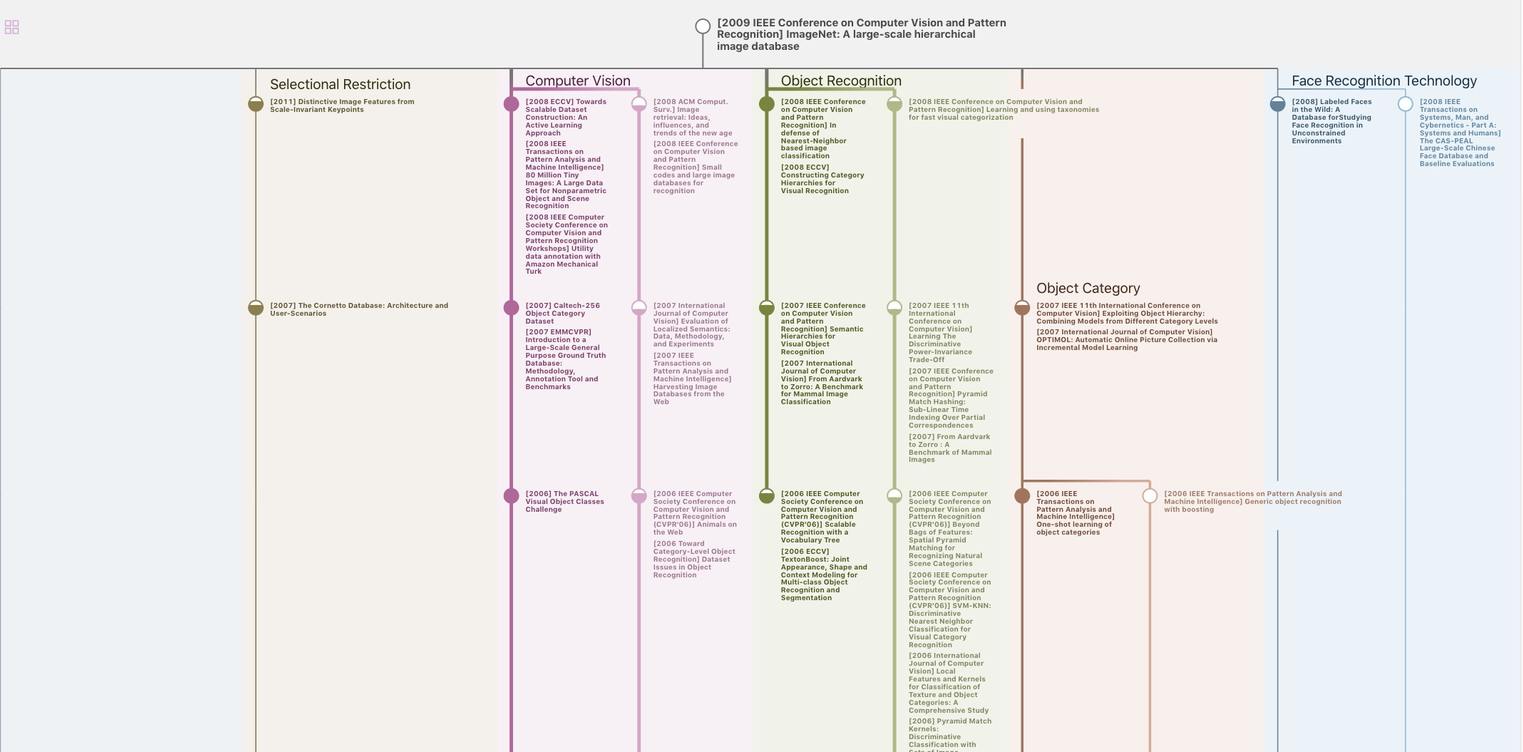
生成溯源树,研究论文发展脉络
Chat Paper
正在生成论文摘要