Learning Domain-Independent Heuristics for Grounded and Lifted Planning
AAAI 2024(2024)
摘要
We present three novel graph representations of planning tasks suitable for learning domain-independent heuristics using Graph Neural Networks (GNNs) to guide search. In particular, to mitigate the issues caused by large grounded GNNs we present the first method for learning domain-independent heuristics with only the lifted representation of a planning task. We also provide a theoretical analysis of the expressiveness of our models, showing that some are more powerful than STRIPS-HGN, the only other existing model for learning domain-independent heuristics. Our experiments show that our heuristics generalise to much larger problems than those in the training set, vastly surpassing STRIPS-HGN heuristics.
更多查看译文
关键词
PRS: Learning for Planning and Scheduling,PRS: Model-Based Reasoning,PRS: Other Foundations of Planning, Routing & Scheduling,PRS: Planning/Scheduling and Learning,SO: Learning to Search
AI 理解论文
溯源树
样例
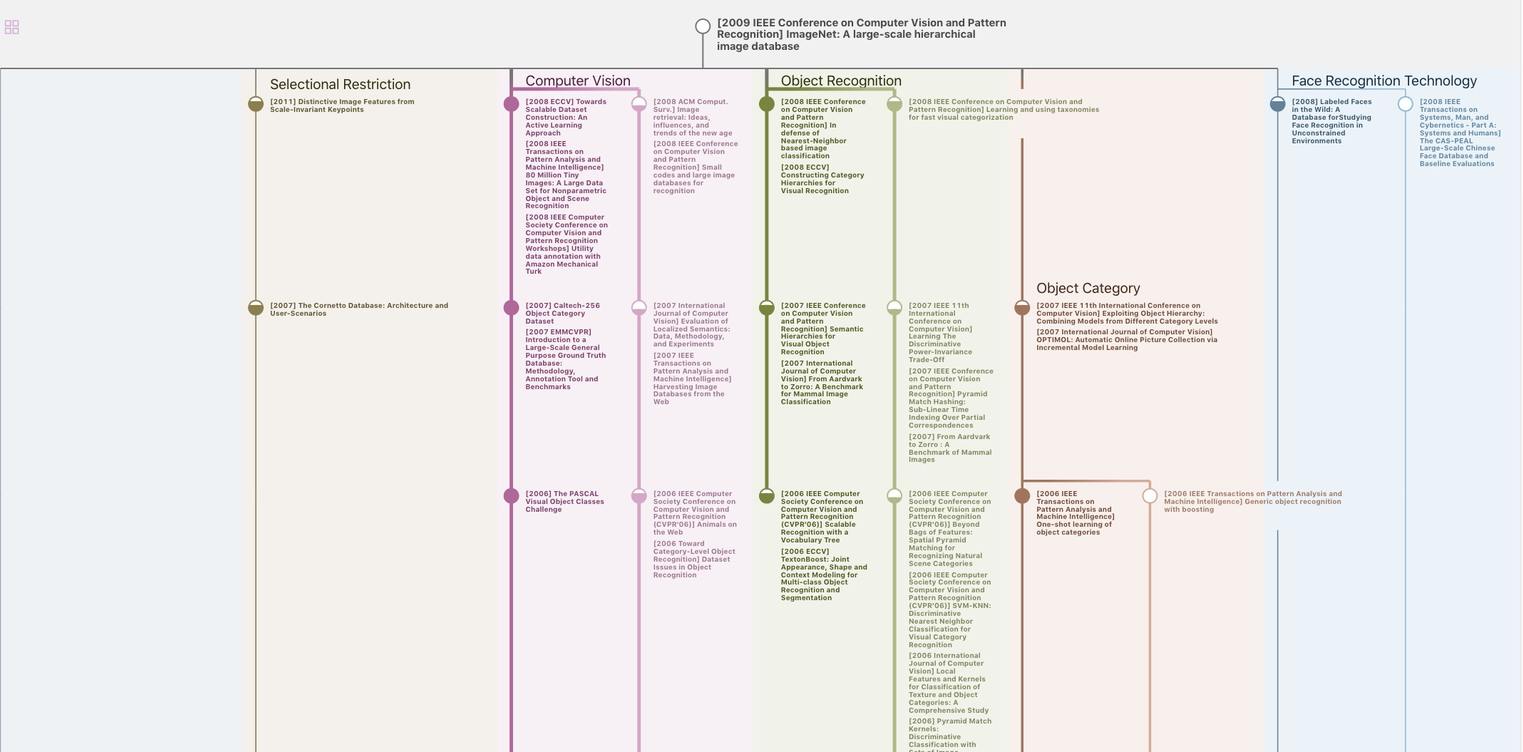
生成溯源树,研究论文发展脉络
Chat Paper
正在生成论文摘要