A Dual-Way Enhanced Framework from Text Matching Point of View for Multimodal Entity Linking
AAAI 2024(2024)
摘要
Multimodal Entity Linking (MEL) aims at linking ambiguous mentions with multimodal information to entity in Knowledge Graph (KG) such as Wikipedia, which plays a key role in many applications. However, existing methods suffer from shortcomings, including modality impurity such as noise in raw image and ambiguous textual entity representation, which puts obstacles to MEL. We formulate multimodal entity linking as a neural text matching problem where each multimodal information (text and image) is treated as a query, and the model learns the mapping from each query to the relevant entity from candidate entities. This paper introduces a dual-way enhanced (DWE) framework for MEL: (1) our model refines queries with multimodal data and addresses semantic gaps using cross-modal enhancers between text and image information. Besides, DWE innovatively leverages fine-grained image attributes, including facial characteristic and scene feature, to enhance and refine visual features. (2)By using Wikipedia descriptions, DWE enriches entity semantics and obtains more comprehensive textual representation, which reduces between textual representation and the entities in KG. Extensive experiments on three public benchmarks demonstrate that our method achieves state-of-the-art (SOTA) performance, indicating the superiority of our model. The code is released on https://github.com/season1blue/DWE.
更多查看译文
关键词
NLP: Language Grounding & Multi-modal NLP,ML: Multi-instance/Multi-view Learning
AI 理解论文
溯源树
样例
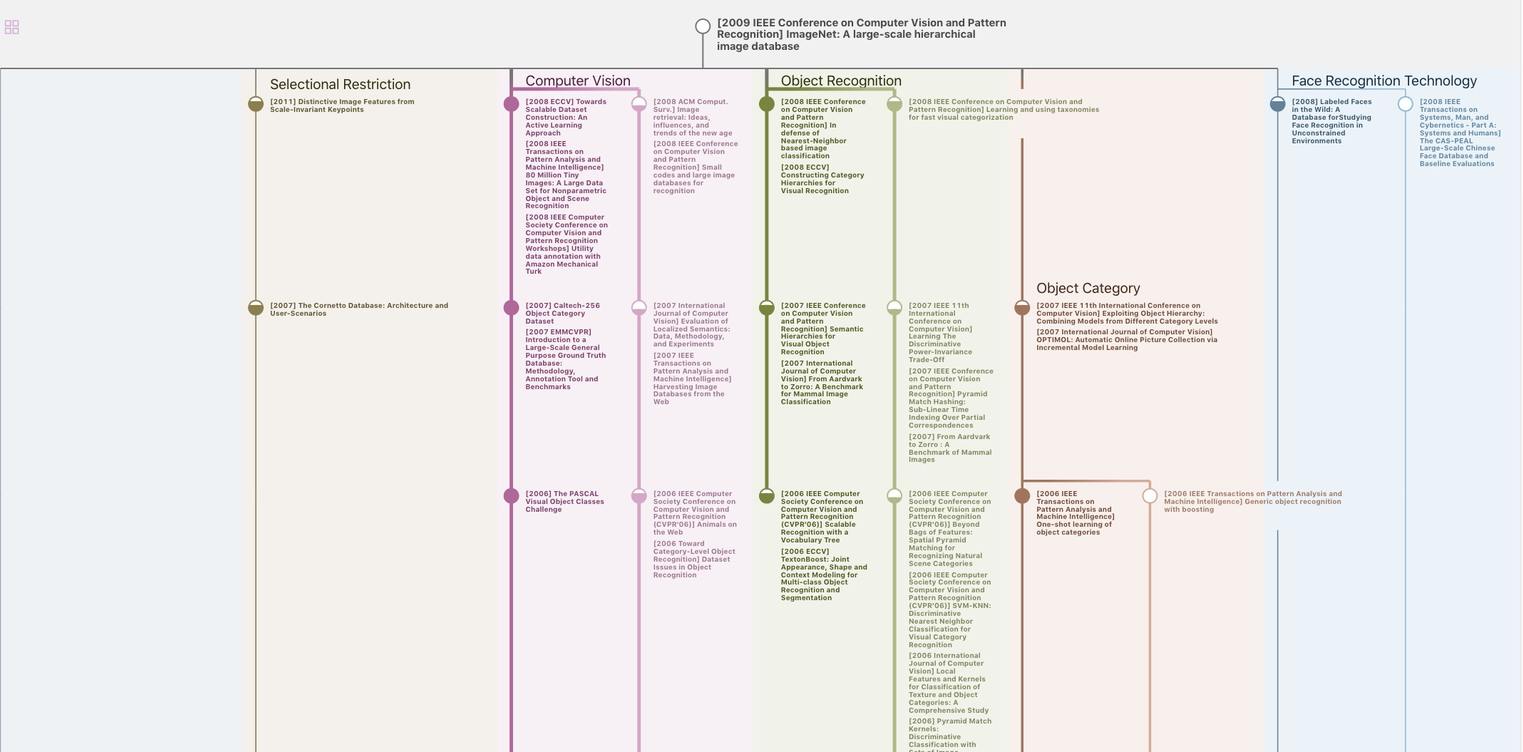
生成溯源树,研究论文发展脉络
Chat Paper
正在生成论文摘要