MELO: Enhancing Model Editing with Neuron-Indexed Dynamic LoRA
AAAI 2024(2024)
摘要
Large language models (LLMs) have shown great success in various Natural Language Processing (NLP) tasks, whist they still need updates after deployment to fix errors or keep pace with the changing knowledge in the world. Researchers formulate such problem as Model Editing and have developed various editors focusing on different axes of editing properties. However, current editors can hardly support all properties and rely on heavy computational resources. In this paper, we propose a plug-in Model Editing method based on neuron-indexed dynamic LoRA (MELO), which alters the behavior of language models by dynamically activating certain LoRA blocks according to the index built in an inner vector database. Our method satisfies various editing properties with high efficiency and can be easily integrated into multiple LLM backbones. Experimental results show that our proposed MELO achieves state-of-the-art editing performance on three sequential editing tasks (document classification, question answering and hallucination correction), while requires the least trainable parameters and computational cost.
更多查看译文
关键词
NLP: Learning & Optimization for NLP,NLP: (Large) Language Models,NLP: Safety and Robustness
AI 理解论文
溯源树
样例
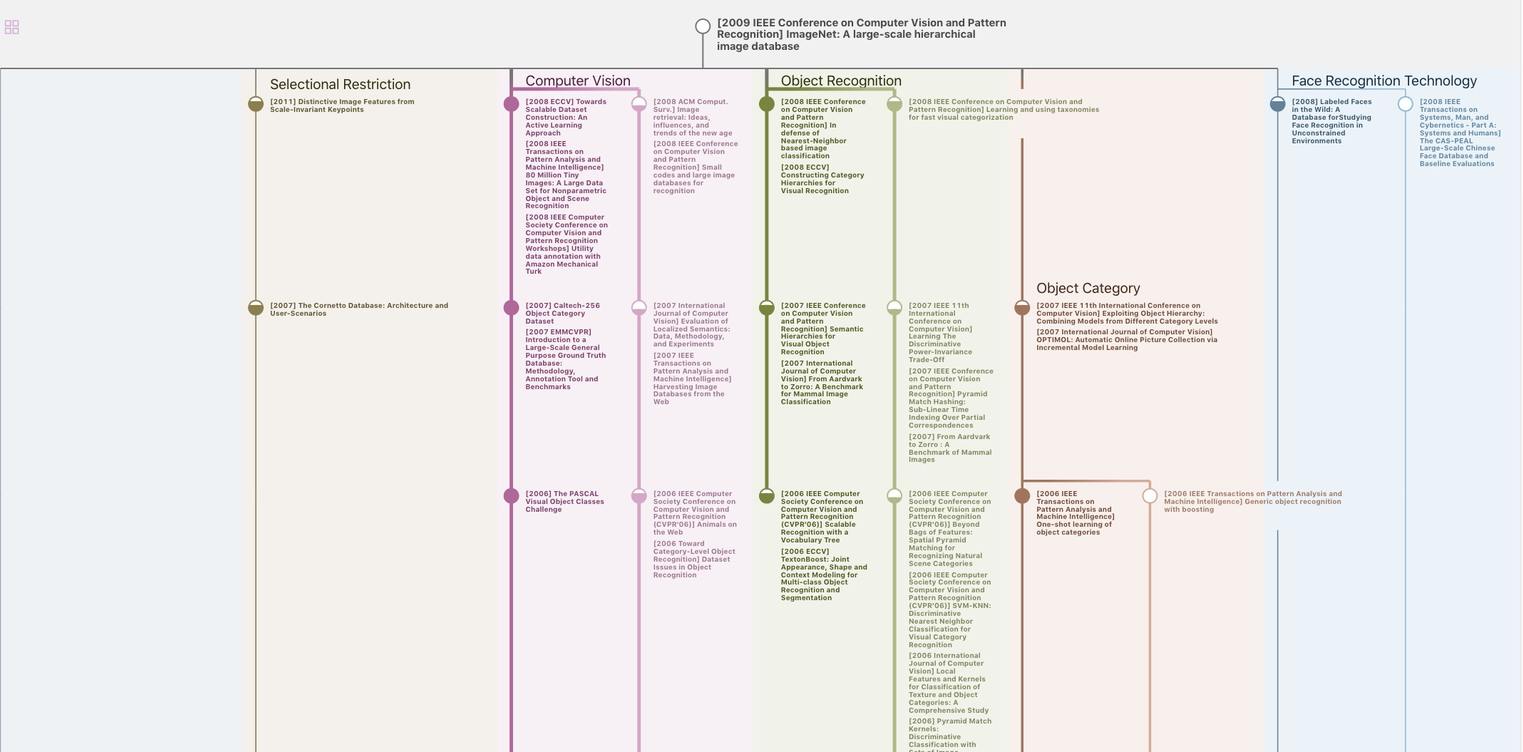
生成溯源树,研究论文发展脉络
Chat Paper
正在生成论文摘要