SoC-Tuner: an Importance-guided Exploration Framework for DNN-targeting SoC Design
Asia and South Pacific Design Automation Conference(2024)
摘要
Designing a system-on-chip (SoC) for deep neural network (DNN) acceleration requires balancing multiple metrics such as latency, power, and area. However, most existing methods ignore the interactions among different SoC components and rely on inaccurate and error-prone evaluation tools, leading to inferior SoC design. In this paper, we present SoC-Tuner, a DNN-targeting exploration framework to find the Pareto optimal set of SoC configurations efficiently. Our framework constructs a thorough SoC design space of all components and divides the exploration into three phases. We propose an importance-based analysis to prune the design space, a sampling algorithm to select the most representative initialization points, and an information-guided multi-objective optimization method to balance multiple design metrics of SoC design. We validate our framework with the actual very-large-scale-integration (VLSI) flow on various DNN benchmarks and show that it outperforms previous methods. To the best of our knowledge, this is the first work to construct an exploration framework of SoCs for DNN acceleration.
更多查看译文
关键词
System-on-Chip Designs,Deep Neural Network,Multi-objective Optimization,Design Space,Multi-objective Optimization Method,Pareto Optimal Set,Important Parameter,Transformer,Alternative Models,Analytical Tools,Design Parameters,Multi-core,Information Gain,Space Exploration,Learning Settings,Original Space,Support Vector Regression,Deep Neural Network Model,Design Points,Design Setting,Design Space Exploration,Average Vector,L2 Cache,Chip Area,Huge Space,Gaussian Process Model
AI 理解论文
溯源树
样例
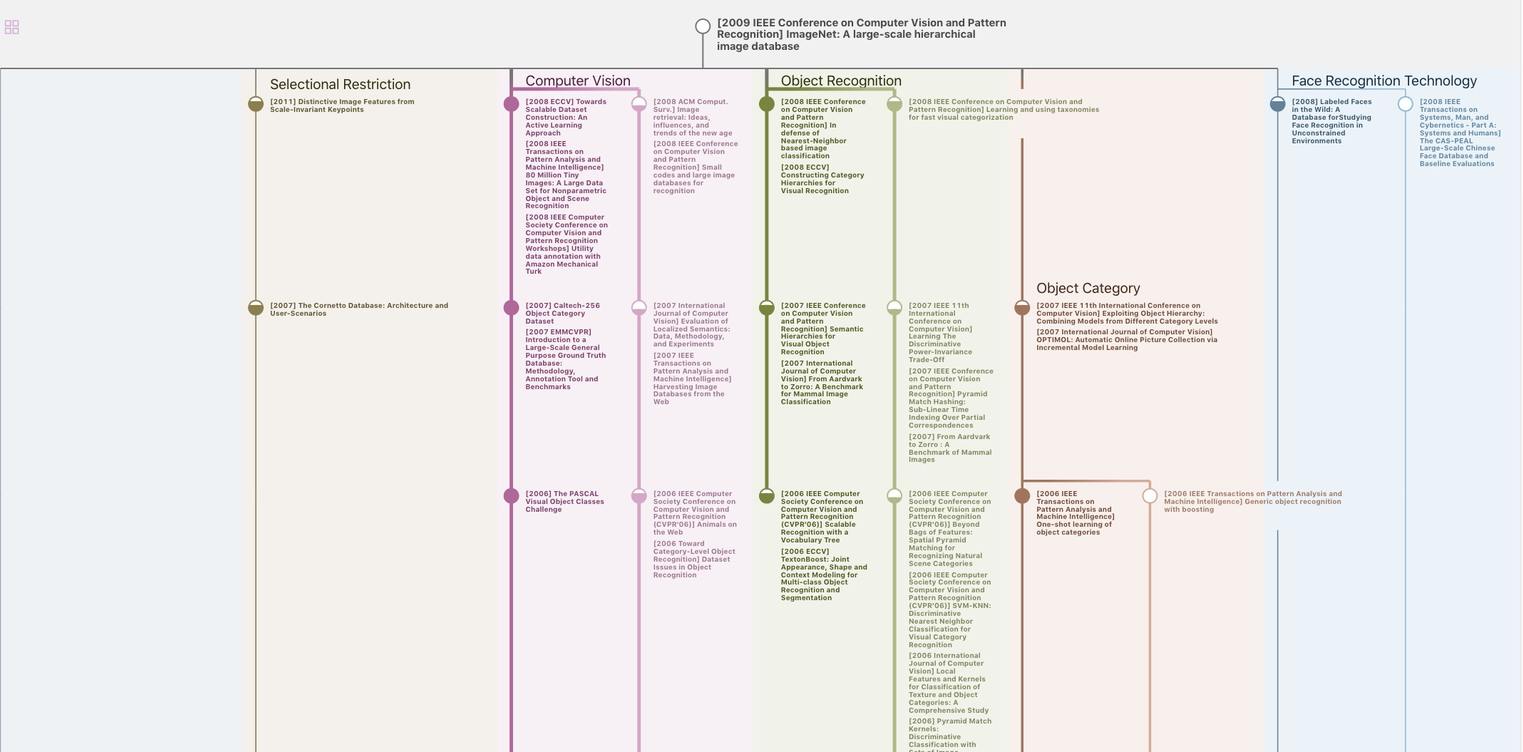
生成溯源树,研究论文发展脉络
Chat Paper
正在生成论文摘要