Squeezed Edge YOLO: Onboard Object Detection on Edge Devices
CoRR(2023)
摘要
Demand for efficient onboard object detection is increasing due to its key role in autonomous navigation. However, deploying object detection models such as YOLO on resource constrained edge devices is challenging due to the high computational requirements of such models. In this paper, an compressed object detection model named Squeezed Edge YOLO is examined. This model is compressed and optimized to kilobytes of parameters in order to fit onboard such edge devices. To evaluate Squeezed Edge YOLO, two use cases - human and shape detection - are used to show the model accuracy and performance. Moreover, the model is deployed onboard a GAP8 processor with 8 RISC-V cores and an NVIDIA Jetson Nano with 4GB of memory. Experimental results show Squeezed Edge YOLO model size is optimized by a factor of 8x which leads to 76% improvements in energy efficiency and 3.3x faster throughout.
更多查看译文
AI 理解论文
溯源树
样例
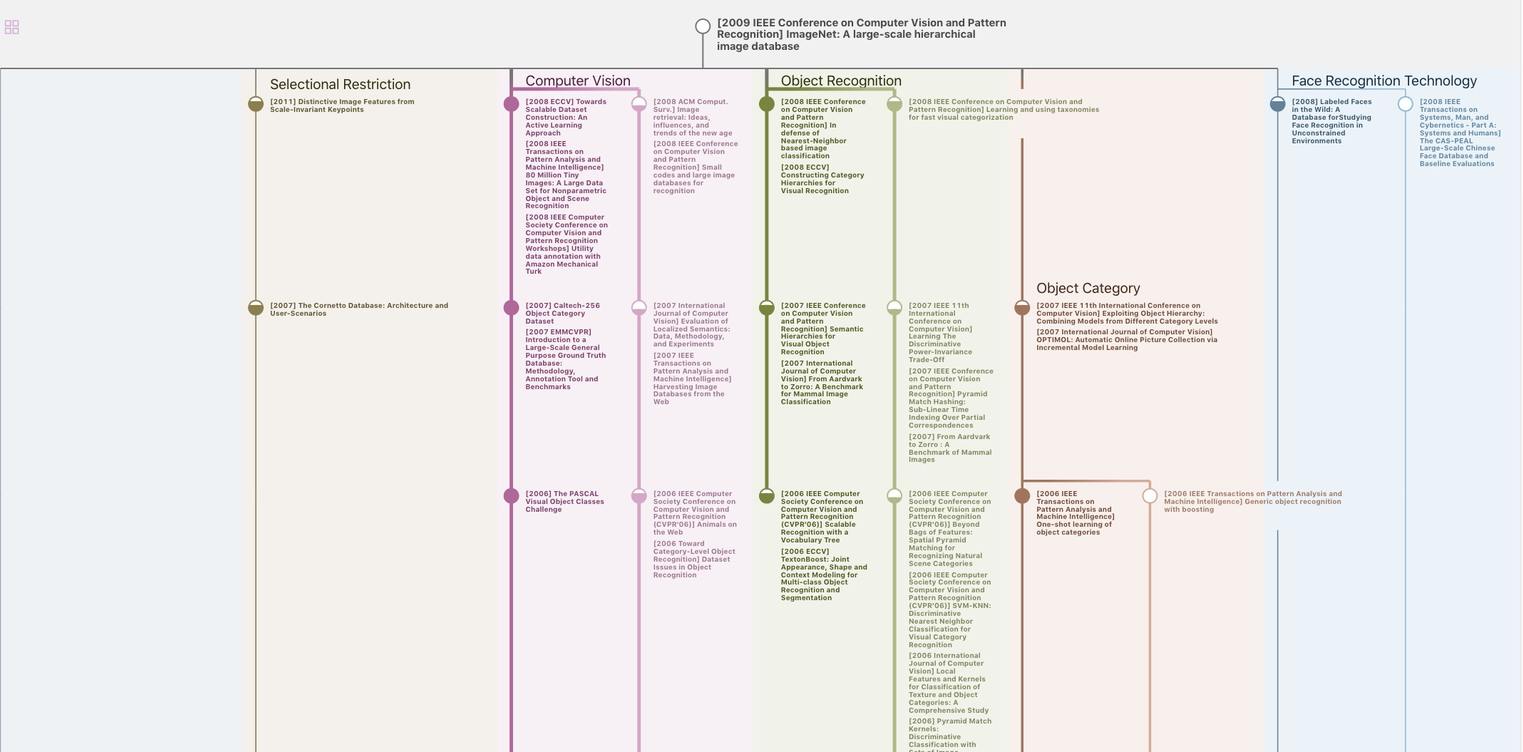
生成溯源树,研究论文发展脉络
Chat Paper
正在生成论文摘要