New Classes of the Greedy-Applicable Arm Feature Distributions in the Sparse Linear Bandit Problem
AAAI 2024(2024)
摘要
We consider the sparse contextual bandit problem where arm feature affects reward through the inner product of sparse parameters. Recent studies have developed sparsity-agnostic algorithms based on the greedy arm selection policy. However, the analysis of these algorithms requires strong assumptions on the arm feature distribution to ensure that the greedily selected samples are sufficiently diverse; One of the most common assumptions, relaxed symmetry, imposes approximate origin-symmetry on the distribution, which cannot allow distributions that has origin-asymmetric support. In this paper, we show that the greedy algorithm is applicable to a wider range of the arm feature distributions from two aspects. Firstly, we show that a mixture distribution that has a greedy-applicable component is also greedy-applicable. Second, we propose new distribution classes, related to Gaussian mixture, discrete, and radial distribution, for which the sample diversity is guaranteed. The proposed classes can describe distributions with origin-asymmetric support and, in conjunction with the first claim, provide theoretical guarantees of the greedy policy for a very wide range of the arm feature distributions.
更多查看译文
关键词
ML: Online Learning & Bandits,ML: Learning Theory
AI 理解论文
溯源树
样例
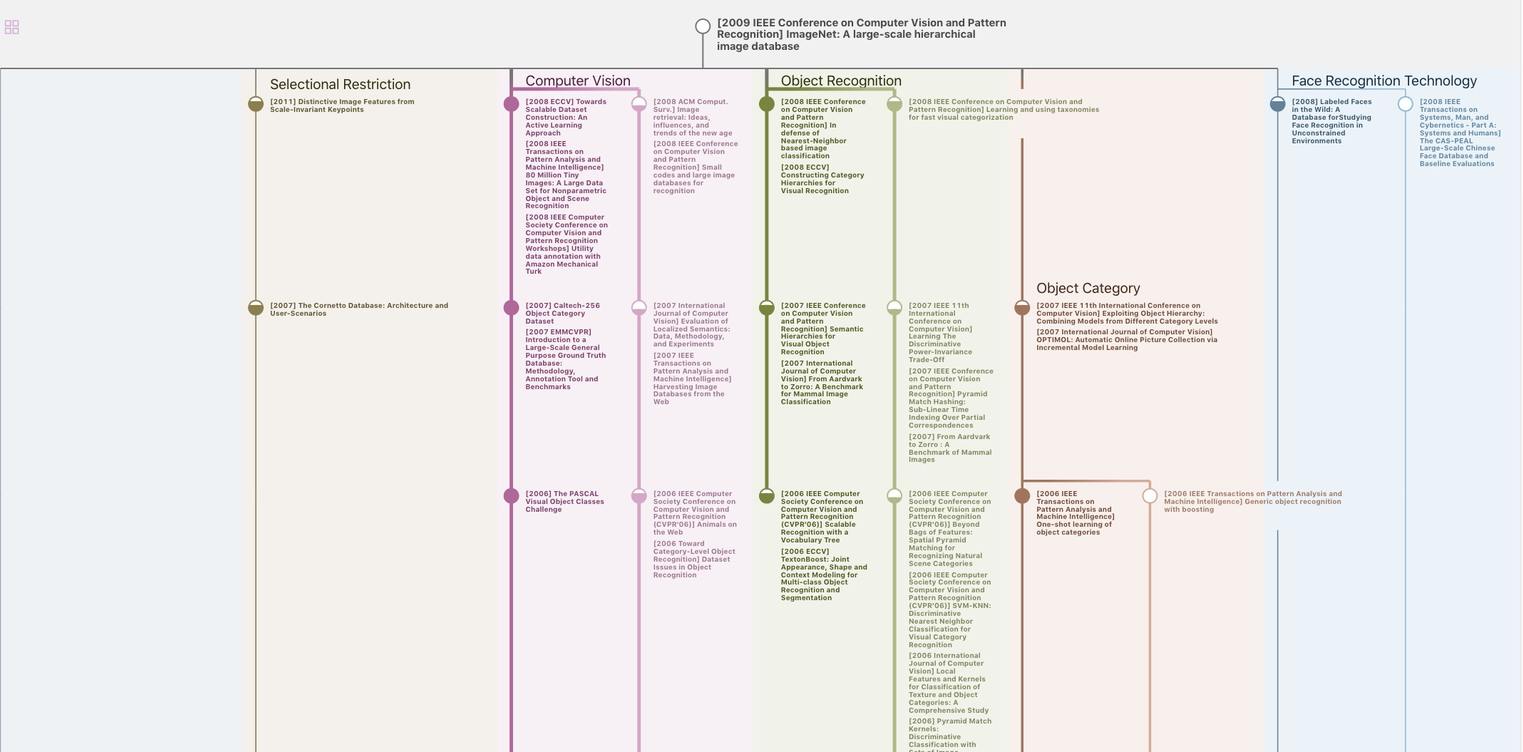
生成溯源树,研究论文发展脉络
Chat Paper
正在生成论文摘要