Utilizing nanotechnology and advanced machine learning for early detection of gastric cancer surgery
ENVIRONMENTAL RESEARCH(2024)
摘要
Nanotechnology has emerged as a promising frontier in revolutionizing the early diagnosis and surgical management of gastric cancers. The primary factors influencing curative efficacy in GIC patients are drug inefficacy and high surgical and pharmacological therapy recurrence rates. Due to its unique optical features, good biocompatibility, surface effects, and small size effects, nanotechnology is a developing and advanced area of study for detecting and treating cancer. Considering the limitations of GIC MRI and endoscopy and the complexity of gastric surgery, the early diagnosis and prompt treatment of gastric illnesses by nanotechnology has been a promising development. Nanoparticles directly target tumor cells, allowing their detection and removal. It also can be engineered to carry specific payloads, such as drugs or contrast agents, and enhance the efficacy and precision of cancer treatment. In this research, the boosting technique of machine learning was utilized to capture nonlinear interactions between a large number of input variables and outputs by using XGBoost and RNN-CNN as a classification method. The research sample included 350 patients, comprising 200 males and 150 females. The patients' mean +/- SD was 50.34 +/- 13.04 with a mean age of 50.34 +/- 13.04. Highrisk behaviors (P = 0.070), age at diagnosis (P = 0.034), distant metastasis (P = 0.004), and tumor stage (P = 0.014) were shown to have a statistically significant link with GC patient survival. AUC was 93.54%, Accuracy 93.54%, F1-score 93.57%, Precision 93.65%, and Recall 93.87% when analyzing stomach pictures. Integrating nanotechnology with advanced machine learning techniques holds promise for improving the diagnosis and treatment of gastric cancer, providing new avenues for precision medicine and better patient outcomes.
更多查看译文
关键词
Gastric cancer (GIC),Nanotechnology,Machine learning (ML),Convolutional neural networks (CNN),Recurrent neural network (RNN),eXtreme gradient boosting (XGBoost)
AI 理解论文
溯源树
样例
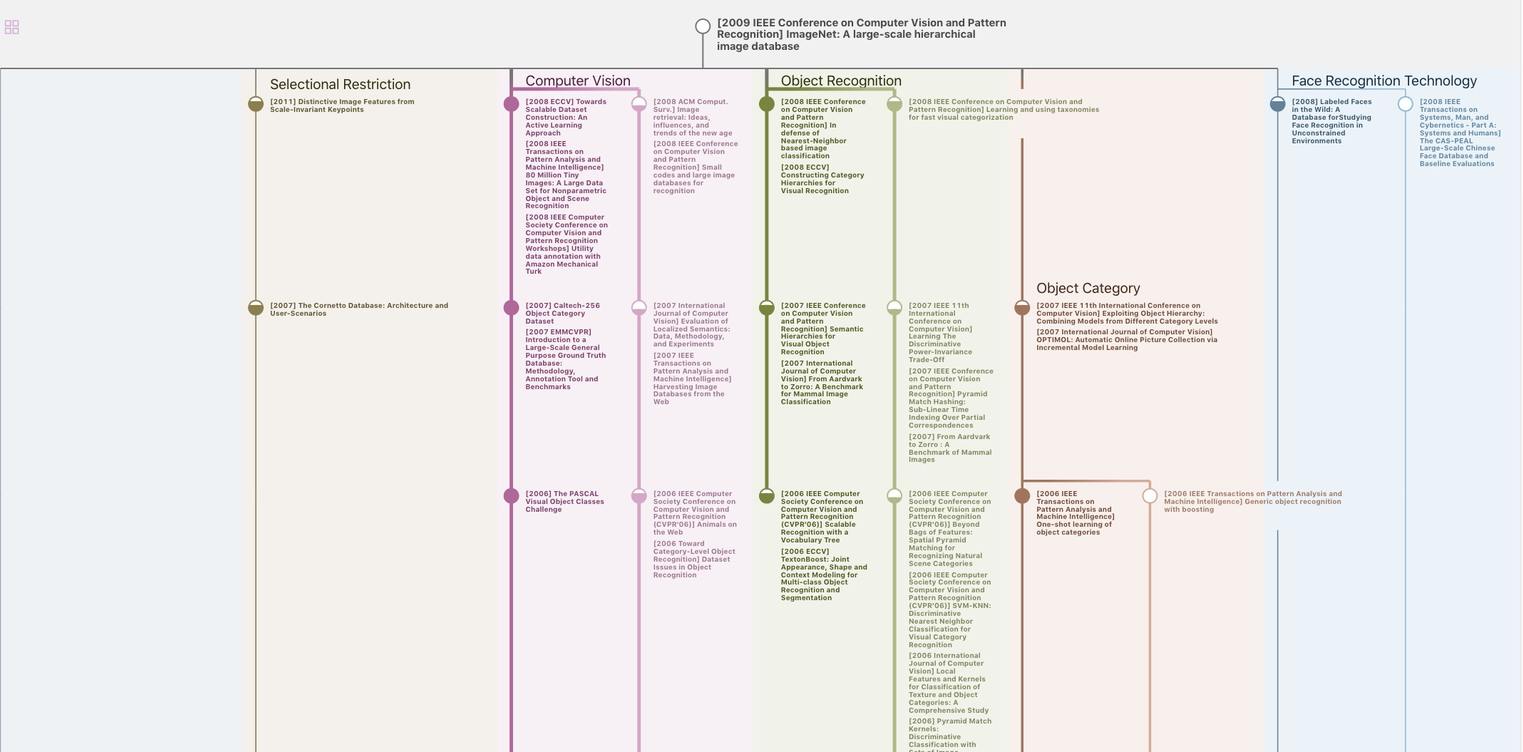
生成溯源树,研究论文发展脉络
Chat Paper
正在生成论文摘要