Evaluating CH4/N2 Separation Performances of Hundreds of Thousands of Real and Hypothetical MOFs by Harnessing Molecular Modeling and Machine Learning.
ACS applied materials & interfaces(2023)
摘要
Considering the large abundance and diversity of metal-organic frameworks (MOFs), evaluating the gas adsorption and separation performance of the entire MOF material space using solely experimental techniques or brute-force computer simulations is impractical. In this study, we integrated high-throughput molecular simulations with machine learning (ML) to explore the potential of both synthesized, the real MOFs, and computer-generated, the hypothetical MOFs (hypoMOFs), for adsorption-based CH4/N-2 separation. CH4/N-2 mixture adsorption data obtained from molecular simulations were used to train the ML models that could accurately predict gas uptakes of 4612 real MOFs. These models were then transferred to two distinct databases consisting of 98 601 hypoMOFs and 587 anion-pillared hypoMOFs to examine their CH4/N-2 mixture separation performances using various adsorbent evaluation metrics. The top adsorbents were identified for vacuum swing adsorption (VSA) and pressure swing adsorption (PSA) conditions and examined in detail to gain molecular insights into their structural and chemical properties. Results revealed that the hypoMOFs offered high CH4 selectivities, up to 14.8 and 13.6, and high working capacities, up to 3.1 and 5.8 mol/kg, at VSA and PSA conditions, respectively, and many of the hypoMOFs could outperform the real MOFs. Our approach offers a rapid and accurate assessment of the mixture adsorption and separation properties of MOFs without the need for computationally demanding simulations. Our results for the best adsorbents will be useful in accelerating the experimental efforts for the design of novel MOFs that can achieve high-performance CH4/N-2 separation.
更多查看译文
关键词
metal-organic framework,molecular simulation,machine learning,adsorption,gas separation
AI 理解论文
溯源树
样例
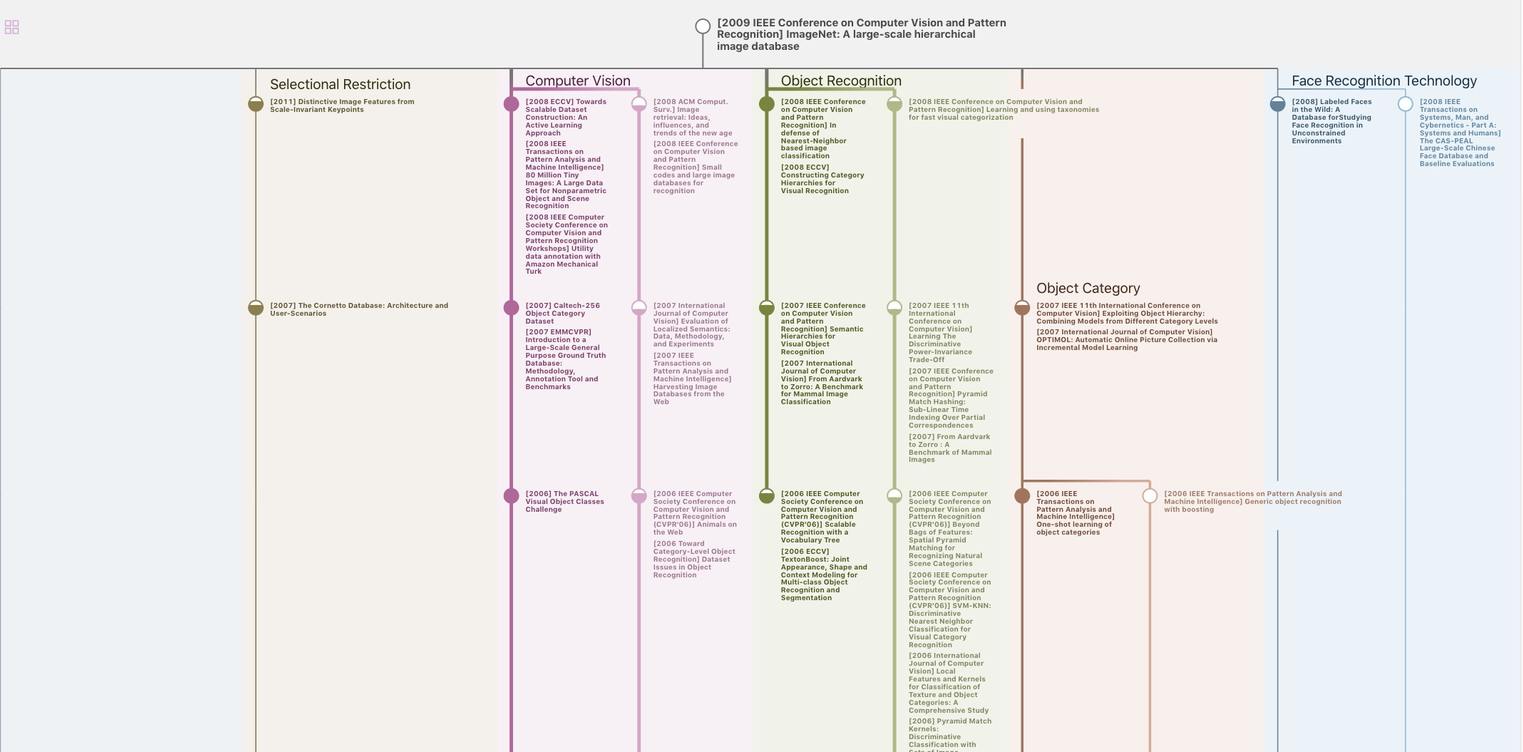
生成溯源树,研究论文发展脉络
Chat Paper
正在生成论文摘要