Population-Based Artificial Intelligence Assessment of Relationship Between the Risk Factors for Diabetic Retinopathy in Indian Population
OPHTHALMIC EPIDEMIOLOGY(2023)
摘要
PurposeRisk factors (RFs), like 'body mass index (BMI),' 'age,' and 'gender' correlate with Diabetic Retinopathy (DR) diagnosis and have been widely studied. This study examines how these three secondary RFs independently affect the predictive capacity of primary RFs.MethodsThe dataset consisted of four population-based studies on the prevalence of DR and associated RFs in India between 2001 and 2010. An Autoencoder was employed to categorize RFs as primary or secondary. This study evaluated six primary RFs coupled independently with each secondary RF on five machine-learning models.ResultsThe secondary RF 'gender' gave a maximum increase in Area under the curve (AUC) score to predict DR when combined separately with 'insulin treatment,' 'fasting plasma glucose,' 'hypertension history,' and 'glycosylated hemoglobin' with a maximum increase in AUC for the Naive Bayes model from 0.573 to 0.646, for the Support Vector Machines (SVM) model from 0.644 to 0.691, for the SVM model from 0.487 to 0.607, and for the Decision Tree model from 0.8 to 0.848, respectively. The secondary RFs 'age' and 'BMI' gave a maximum increase in AUC score to predict DR when combined separately with 'diabetes mellitus duration' and 'systolic blood pressure,' with a maximum increase in AUC for the SVM model from 0.389 to 0.621, and for the Decision Tree model from 0.617 to 0.713, respectively.ConclusionThe risk factor 'gender' was the best secondary RF in predicting DR compared to 'age' and 'BMI,' increasing the predictive power of four primary RFs.
更多查看译文
关键词
Diabetic retinopathy,autoencoder,feature selection,primary,risk factor,secondary
AI 理解论文
溯源树
样例
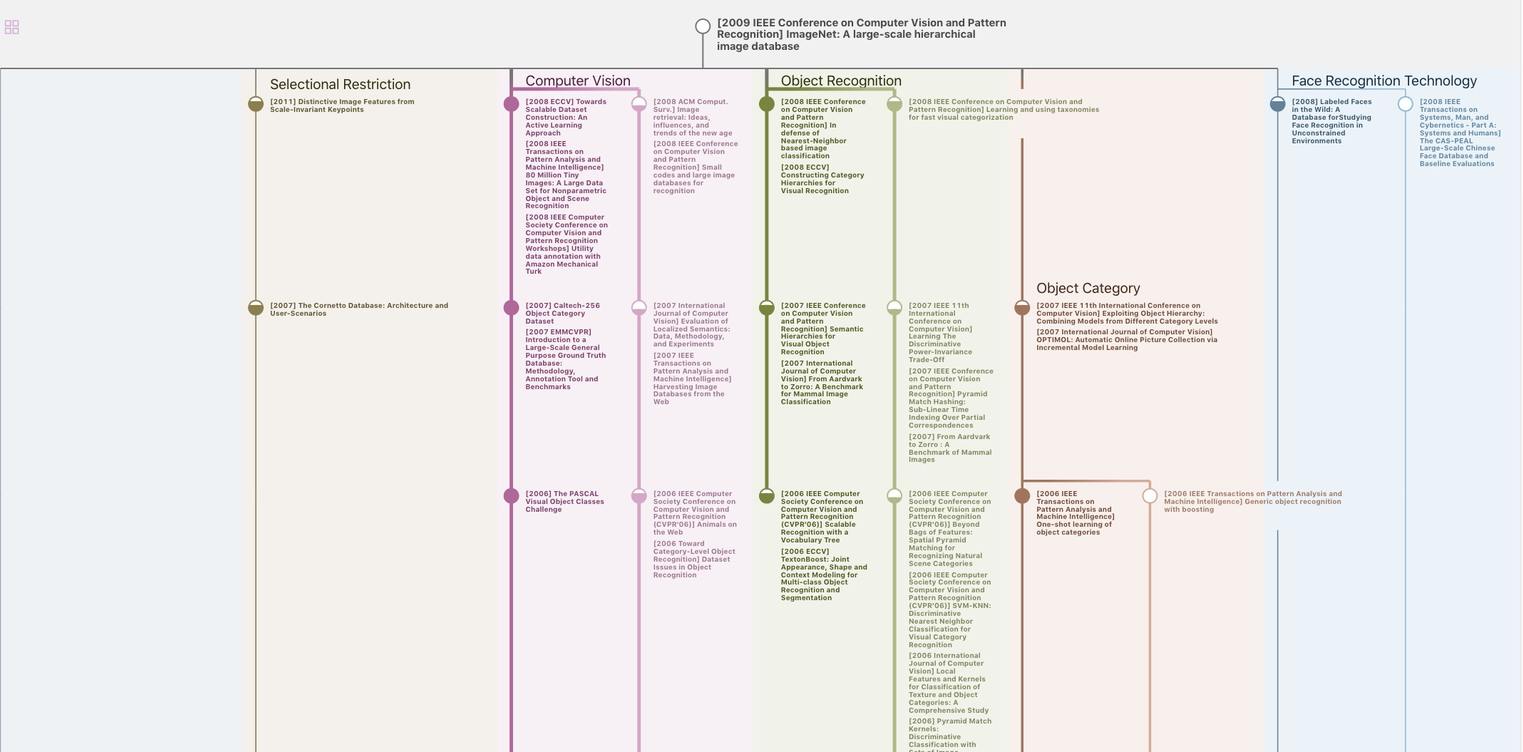
生成溯源树,研究论文发展脉络
Chat Paper
正在生成论文摘要