Enhancing Person Re-Identification Performance Through In Vivo Learning
IEEE TRANSACTIONS ON IMAGE PROCESSING(2024)
摘要
This research investigates the potential of in vivo learning to enhance visual representation learning for image-based person re-identification (re-ID). Compared to traditional self-supervised learning (which require external data), the introduced in vivo learning utilizes supervisory labels generated from pedestrian images to improve re-ID accuracy without relying on external data sources. Three carefully designed in vivo learning tasks, leveraging statistical regularities within images, are proposed without the need for laborious manual annotations. These tasks enable feature extractors to learn more comprehensive and discriminative person representations by jointly modeling various aspects of human biological structure information, contributing to enhanced re-ID performance. Notably, the method seamlessly integrates with existing re-ID frameworks, requiring minimal modifications and no additional data beyond the existing training set. Extensive experiments on diverse datasets, including Market1501, CUHK03-NP, Celeb-reID, Celeb-reid-light, PRCC, and LTCC, demonstrate substantial enhancements in rank-1 precision compared to state-of-the-art methods.
更多查看译文
关键词
Person re-identification,in vivo learning,boosting performance
AI 理解论文
溯源树
样例
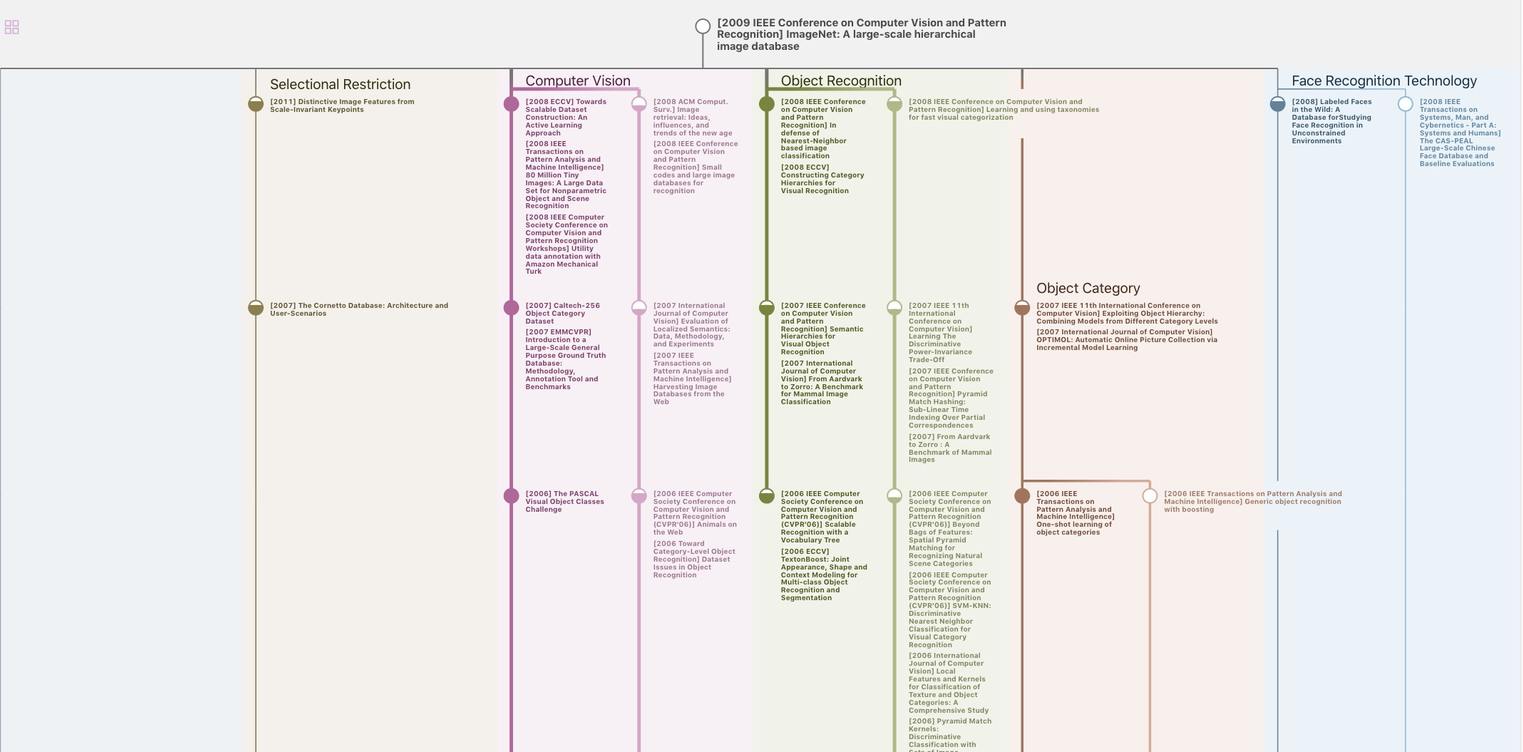
生成溯源树,研究论文发展脉络
Chat Paper
正在生成论文摘要