Prediction of Critical Heat Flux and Position in Narrow Rectangular Channels Using Deep Feed-Forward Neural Networks Coupling with Empirical Correlations
International Journal of Heat and Mass Transfer(2024)
摘要
The critical heat flux (CHF) is a crucial thermo-hydraulic parameter or phenomenon in boiling heat transfer in many industry applications. For a long narrow rectangular channel, a fundamental heat transfer unit in the nuclear industry, the CHF is hard to measure and predict owing to the large aspect ratio (>1000) in geometry. Current technologies can only predict the value of CHF. Still, they cannot predict where the CHF occurs (the positions occurring dry out in narrow rectangular channels) in the large aspect ratio narrow rectangular channel. In this study, we establish a nonlinear relationship between CHF values and their specific occurrence locations under various operating conditions (various inlet mass flow rate, inlet temperature, wall heat flux density) using deep feed-forward neural networks (DFNN) coupled with CHF empirical correlations. Compared with the traditional empirical correlation method and the neural networks (NN) method, the new proposed method can predict both the value of CHF and the specific location where the CHF occurs. By inducing the empirical correlations into the neural networks, the prediction error decreases from 10% to 5% compared to the traditional NN methods. This work aims to provide a predictable and controllable strategy for CHF in narrow rectangular channels.
更多查看译文
关键词
narrow rectangular channels,critical heat flux,critical heat flux occurring position,deep feed-forward neural networks
AI 理解论文
溯源树
样例
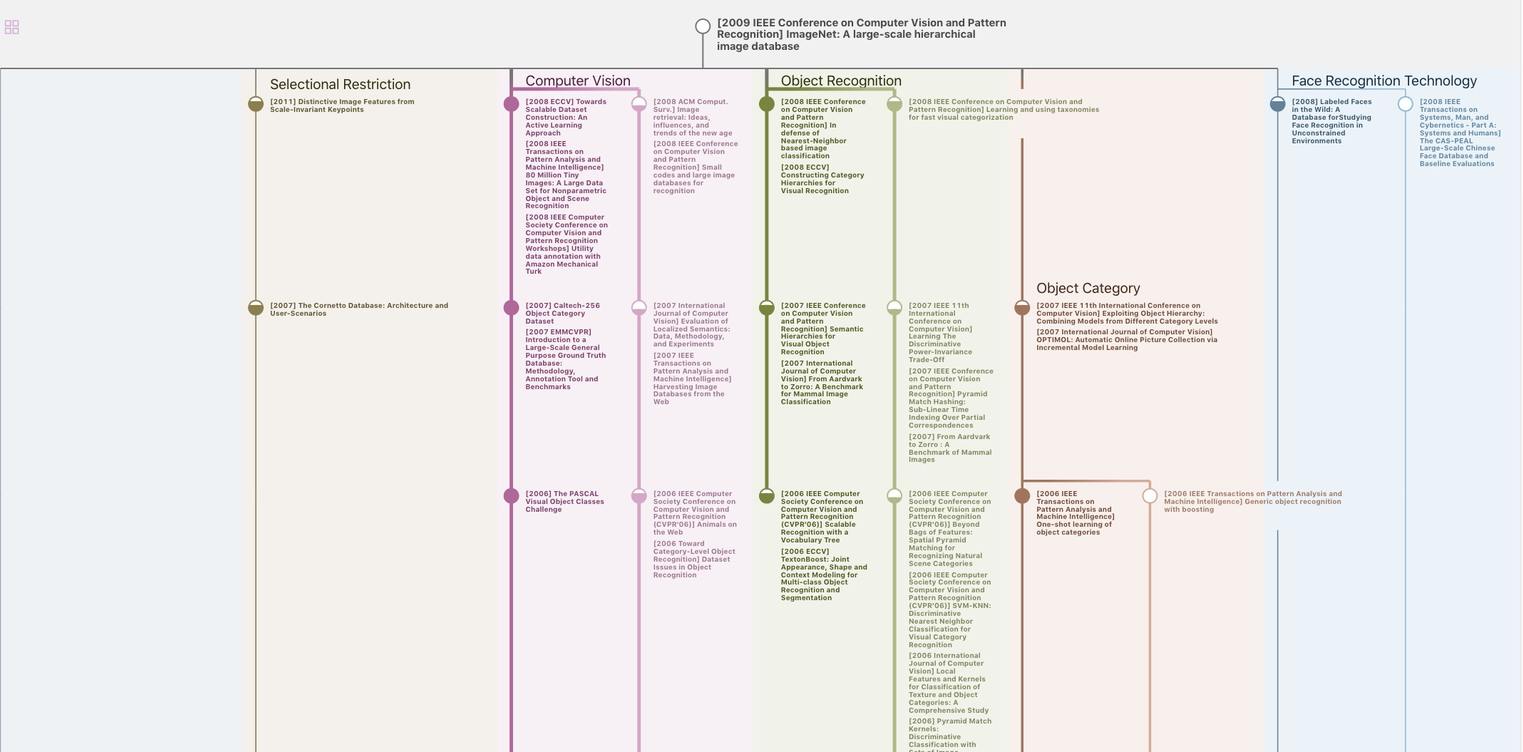
生成溯源树,研究论文发展脉络
Chat Paper
正在生成论文摘要