Preformer: Simple and Efficient Design for Precipitation Nowcasting With Transformers
IEEE GEOSCIENCE AND REMOTE SENSING LETTERS(2024)
摘要
The primary objective of precipitation nowcasting is to predict precipitation patterns several hours in advance. Recent studies have emphasized the potential of deep learning methods for this task. To harness the correlations among various meteorological elements, existing frameworks project multiple meteorological elements into a latent space and then utilize convolutional-recurrent networks for future precipitation prediction. Although effective, the escalating model complexity may impede practical applications. This letter develops the Preformer, a streamlined Transformer framework for precipitation nowcasting that efficiently captures global spatiotemporal dependencies among multiple meteorological elements. The Preformer implements an encoder-translator-decoder architecture, where the encoder integrates spatial features of multiple elements, the translator models spatiotemporal dynamics, and the decoder combines spatiotemporal information to forecast future precipitation. Without introducing complex structures or strategies, the Preformer achieves state-of-the-art performance even with the least parameters.
更多查看译文
关键词
Precipitation,Transformers,Spatiotemporal phenomena,Decoding,Humidity,Correlation,Computer architecture,Data mining,precipitation nowcasting,transformer
AI 理解论文
溯源树
样例
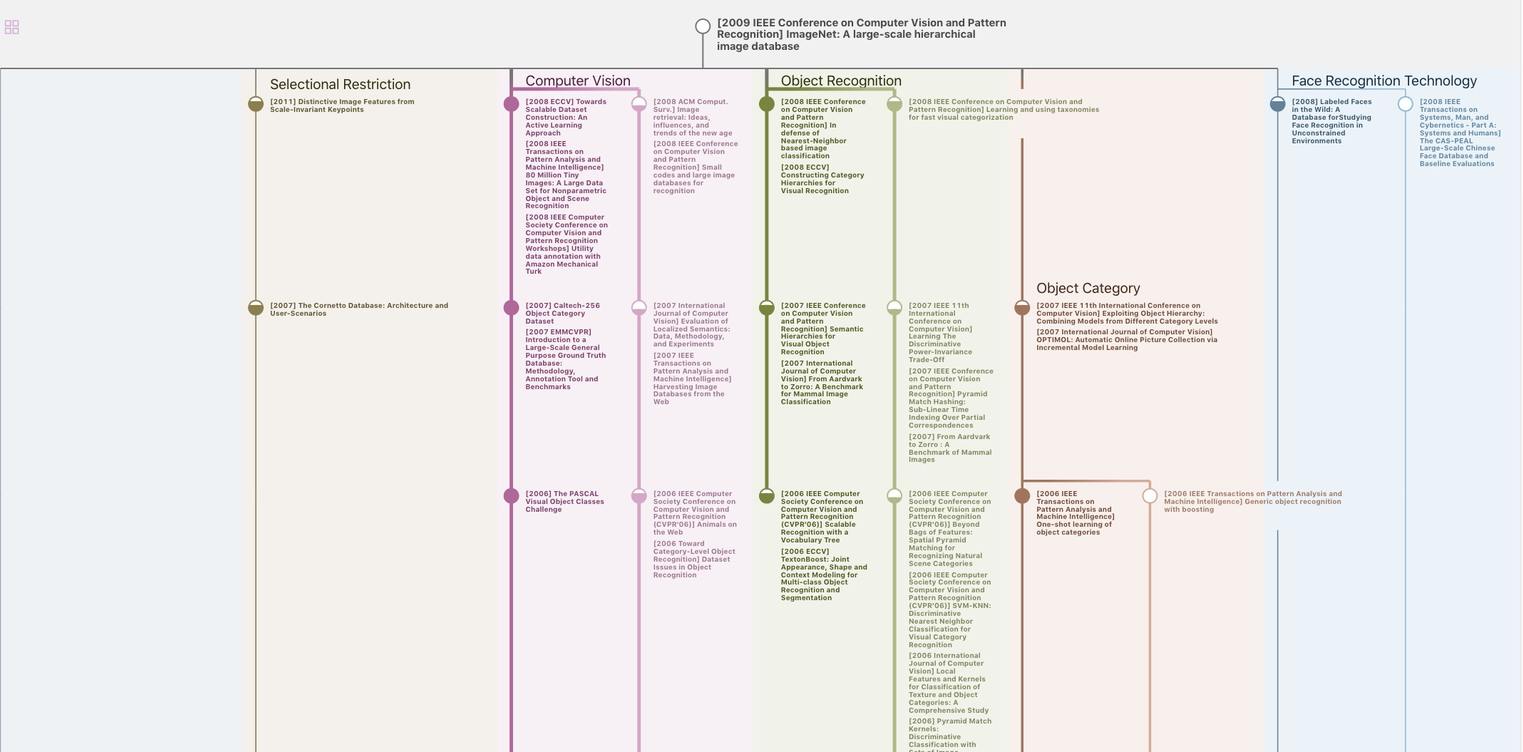
生成溯源树,研究论文发展脉络
Chat Paper
正在生成论文摘要