Efficient Remote Sensing Image Super-Resolution Via Lightweight Diffusion Models.
IEEE geoscience and remote sensing letters(2024)
摘要
With the emergence of diffusion models, the image generation has experienced a significant advancement. In super-resolution tasks, diffusion models surpass generative adversarial network (GAN)-based methods in generating more realistic samples. However, these models come with significant costs: denoising networks rely on large U-Net, making them computationally intensive for high-resolution (HR) images, and the extensive sampling steps in diffusion models lead to prolonged inference time. This complexity limits their application in remote sensing, due to the high demand for high-resolution images in such scenarios. To address this, we propose a lightweight diffusion model (LWTDM), which simplifies the denoising network and efficiently incorporates conditional information using a cross-attention-based encoder-decoder architecture. Furthermore, LWTDM serves as the pioneering model that incorporates the accelerated sampling technique from denoising diffusion implicit models (DDIMs). This integration involves the meticulous selection of sampling steps, ensuring the quality of the generated images. The experiments confirm that LWTDM strikes a favorable balance between precision and perceptual quality, while its faster inference speed makes it suitable for diverse remote sensing scenarios with specific requirements. The source code is available at: https://github.com/Suanmd/LWTDM.
更多查看译文
关键词
Cross-attention mechanism,lightweight diffusion models (LWTDM),remote sensing super-resolution,satellite imagery
AI 理解论文
溯源树
样例
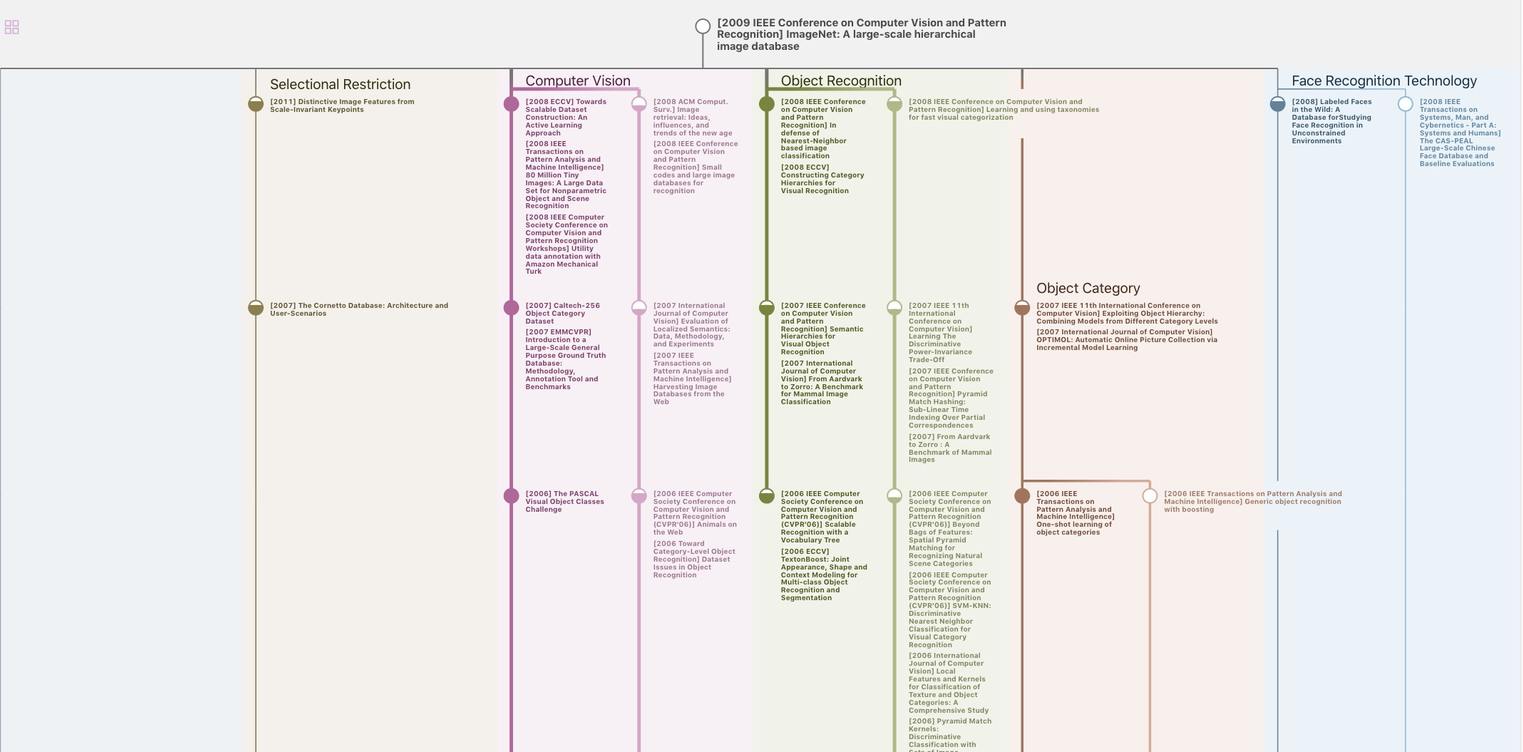
生成溯源树,研究论文发展脉络
Chat Paper
正在生成论文摘要