Cross-Modal Feature Fusion and Interaction Strategy for CNN-Transformer-Based Object Detection in Visual and Infrared Remote Sensing Imagery
IEEE GEOSCIENCE AND REMOTE SENSING LETTERS(2024)
摘要
Due to the complementarity of visible and infrared images, it has become more favorable to fuse these two modalities to improve the object detection accuracy in the remote sensing area. However, there are still some problems to be solved. Most of the existing algorithms focus too much on the local information and ignore long-range information when performing feature extraction on different modalities. Besides, coarse weighted fusion strategies do not fully utilize the information from different modalities, and the fusion structure ignores the importance of intermodal information exchange. To tackle these problems, a cross-modal feature fusion and interaction strategy for the convolutional neural network (CNN)-transformer-based object detection in visual and infrared remote sensing imagery is proposed. We adopt a parallel structure to extract the features of different modalities, separately. In visual and infrared modality, the convolutional layers and transformer encoders are cascaded to fully extract both local and long-range information. The cross-modal feature fusion and interaction module (CFFIM) adopts the attention mechanisms to jointly fuse different modal features at the same scale to improve the diversity of fused features, and the feature interaction enables the sharing of visible and infrared information. Experiments on the VEDAI dataset have demonstrated the effectiveness of the proposed scheme compared to other state-of-the-art algorithms.
更多查看译文
关键词
Feature extraction,Object detection,Transformers,Visualization,Convolution,Sun,Remote sensing,Feature fusion,object detection,vision transformer,visual and infrared remote sensing imagery
AI 理解论文
溯源树
样例
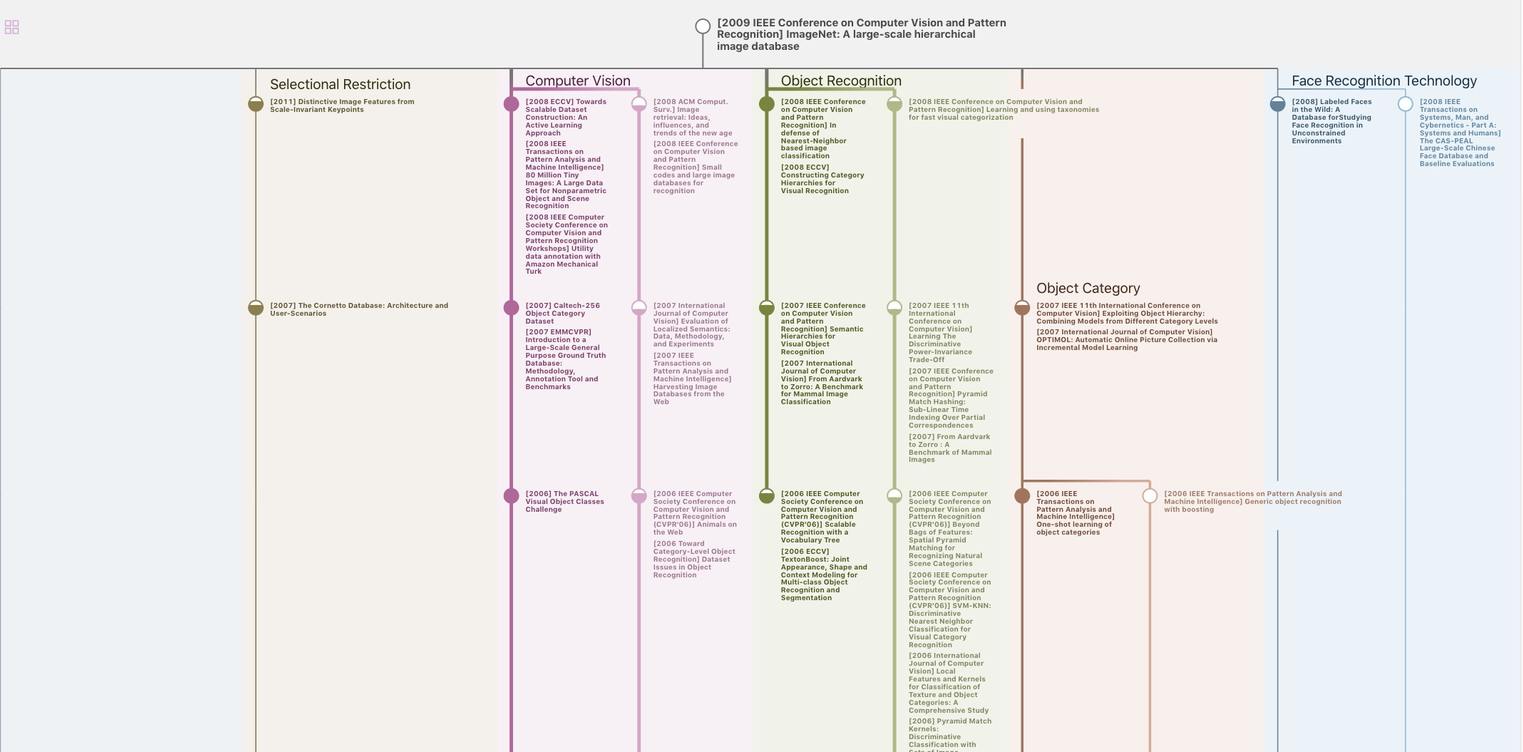
生成溯源树,研究论文发展脉络
Chat Paper
正在生成论文摘要