Structurally-Constrained Unsupervised Deep Learning for Seismic High-Resolution Reconstruction
IEEE TRANSACTIONS ON GEOSCIENCE AND REMOTE SENSING(2024)
摘要
Seismic high-resolution reconstruction is a key step in retrieving high-resolution components to characterize subsurface geological structures. Recently, deep learning (DL) has emerged as an effective technique in seismic resolution improvement; however, most DL methods with deep networks are based on supervised learning, which is purely data-driven and requires a large amount of high-quality labels generated from well-data. Generally, the well-data are expensive to collect through field surveys. In this article, we propose an automatic reflectivity inversion framework for improving the resolution of the seismic data on the basis of an unsupervised DL approach. We leverage a network to adaptively learn features from poststack seismic data by integrating the physical constraint provided by the Robinson convolution model and the prior knowledge encoded in sparse reflectivity model, and then use these learned features to reconstruct the high-resolution data. Moreover, we incorporate a lateral structural constraint as a specific prior into the training process of the neural network to enhance its ability to recover more structural details and improve the stability of the results. Experimental results on both synthetic and field datasets validate the performance of our proposed method. Compared with the conventional method, the proposed method can yield more accurate and laterally more consistent high-resolution results.
更多查看译文
关键词
Deep learning (DL),seismic resolution enhancement,spatial continuity,unsupervised learning
AI 理解论文
溯源树
样例
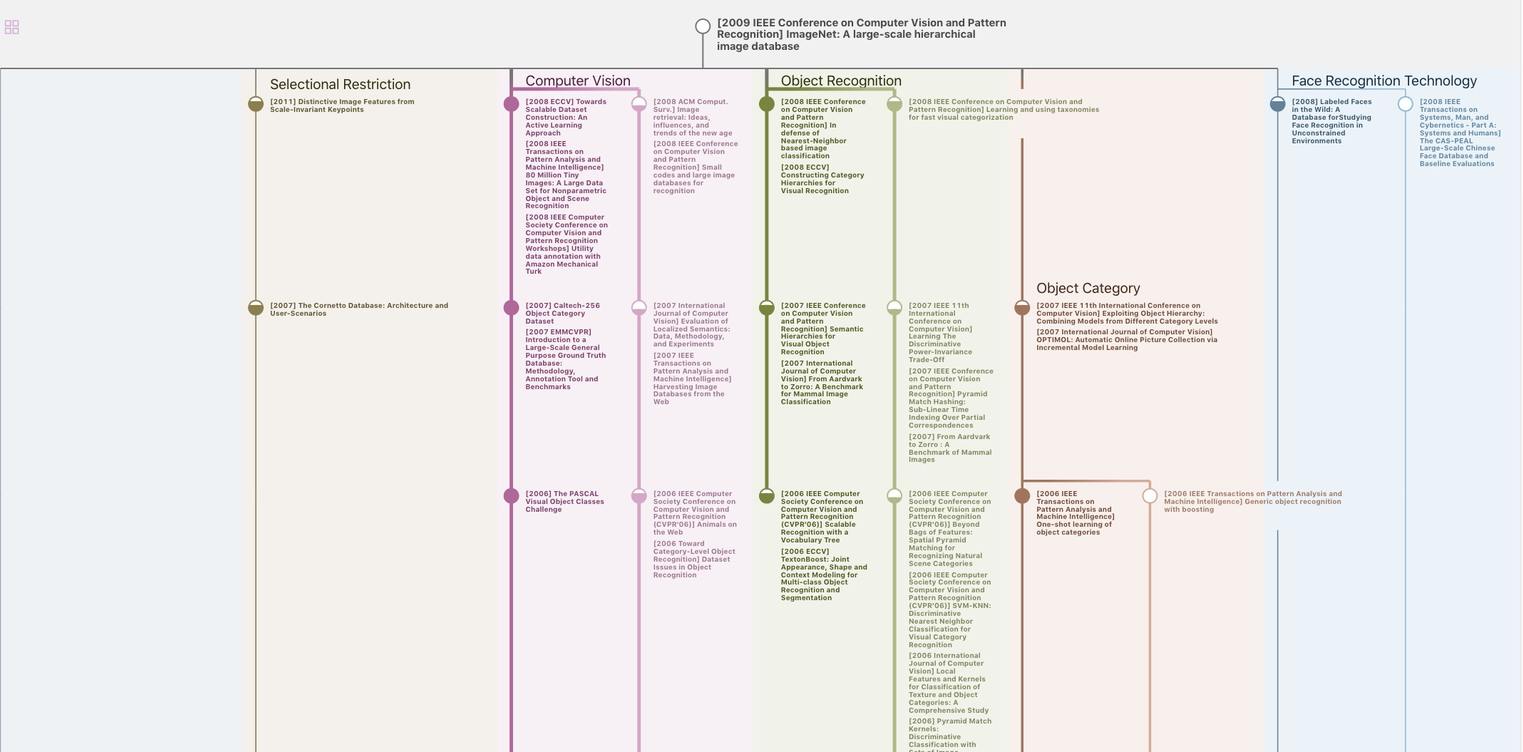
生成溯源树,研究论文发展脉络
Chat Paper
正在生成论文摘要