Cnnformer: Transformer-Based Semantic Information Enhancement Framework for Behavior Recognition
IEEE ACCESS(2023)
摘要
Behavior recognition is a vital task in computer vision. While, semantic information extraction is still insufficient in behavior recognition models. In this paper, we propose an improved behavior recognition model, which is called Cnnformer, to alleviate the problem of inadequate semantic information extraction. Cnnformer is transformer-based semantic information enhancement model for behavior recognition. In Cnnformer, a new attention mechanism is designed and introduced into the encoder module. This attention mechanism uses dilated convolution to capture static context information, trigger mining dynamic context information, and obtain the final fused dynamic and static context information. In addition, four layers of convolution are added in front of the encoder module, which has a strong induction bias to extract the superficial feature representation (such as color, geometry, texture, etc.). Finally, Cnnformer combines the convolution module and the attention module into the encoder module to simultaneously learn both local and global features, so as to enhance visual representation. Experimental results show that Cnnformer has higher performance in behavior recognition, and the accuracy of Top-1 is 3.4% higher than that of the basic model in the Kinetics-400 dataset.
更多查看译文
关键词
Behavior recognition,transformer,convolutional neural networks,semantic information,dilated convolution
AI 理解论文
溯源树
样例
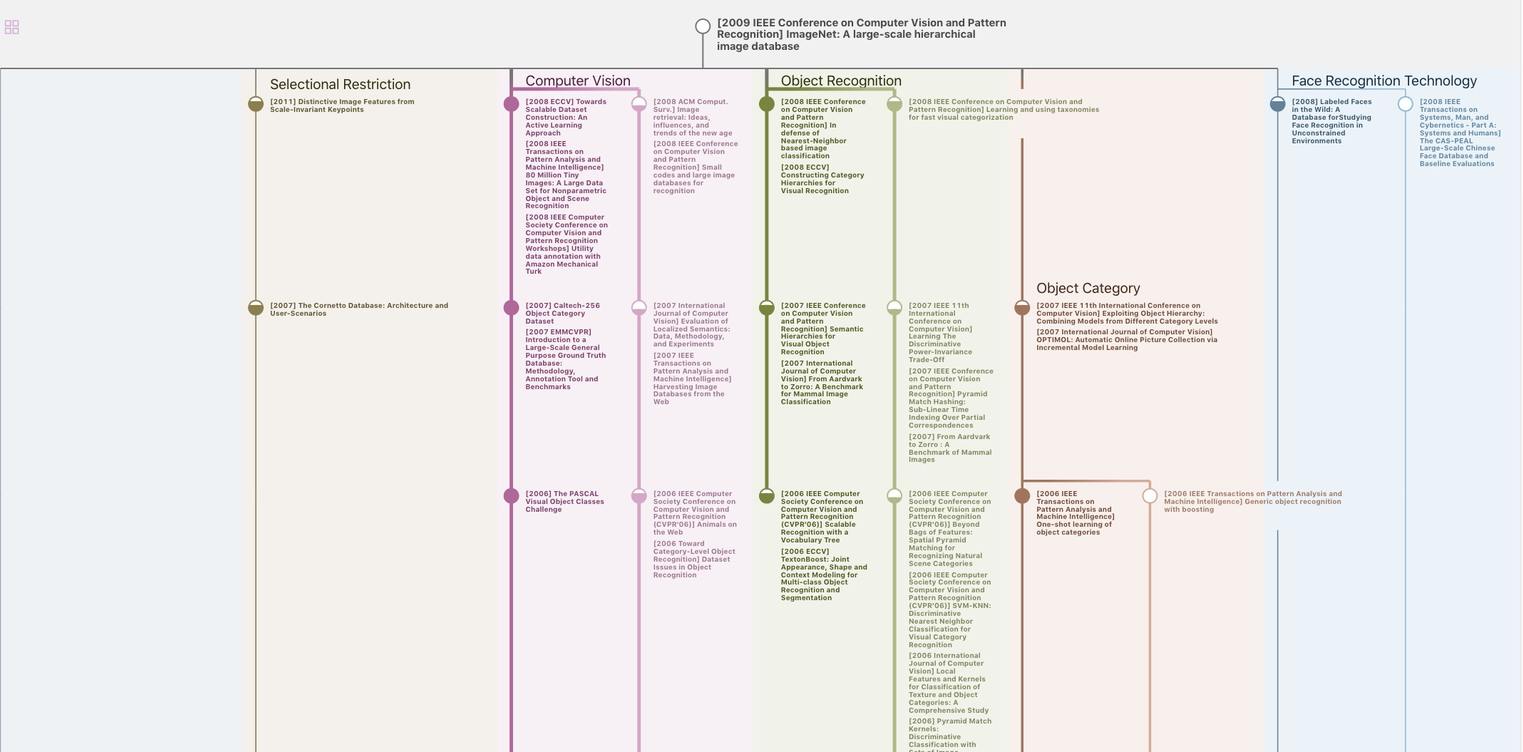
生成溯源树,研究论文发展脉络
Chat Paper
正在生成论文摘要