TL-AttSharpNet: Automated Lung Image Segmentation using Transfer Learning with Depthwise Convolution and Attention
2023 IEEE 2nd National Biomedical Engineering Conference (NBEC)(2023)
摘要
The global community has recently been impacted by the COVID-19 pandemic, regarded as the biggest public health emergency the world has ever seen. Covid-19 has been shown to provoke pneumonia, among other diseases characterized by lung inflammation. Imaging exams such as chest X-rays (CXR) are often used because they are cheap, fast, widespread, and use less radiation. Even though typical CXR images may help early screening of suspected lung disease cases, the images of various viral pneumonias, covid-19, tuberculosis, lung cancer and others are similar and often overlap with other infectious and inflammatory lung diseases. Recently, improvements in deep learning have allowed researchers to develop segmentation models that can automatically segment lung images for easy diagnosis. Segmenting lung images often helps differentiate lung tissue from other structures in the chest, leading to improved detection. However, designing an effective lung segmentation model is a challenging problem since the regions of interest are often confused with the lung tissue. To contribute, this research proposes TL-AttSharpNet, a U-net-like architecture incorporating the pre-trained weights of VGG16 with depthwise convolutions and an attention mechanism for lung image segmentation. Experiments showed that the proposed architecture achieved a 0.9786 dice score and 0.9503 Jaccard, outperforming baseline models and the existing lung image segmentation architectures.
更多查看译文
关键词
Lung Segmentation,Deep Learning,Attention,Transfer Learning,Medical Imaging
AI 理解论文
溯源树
样例
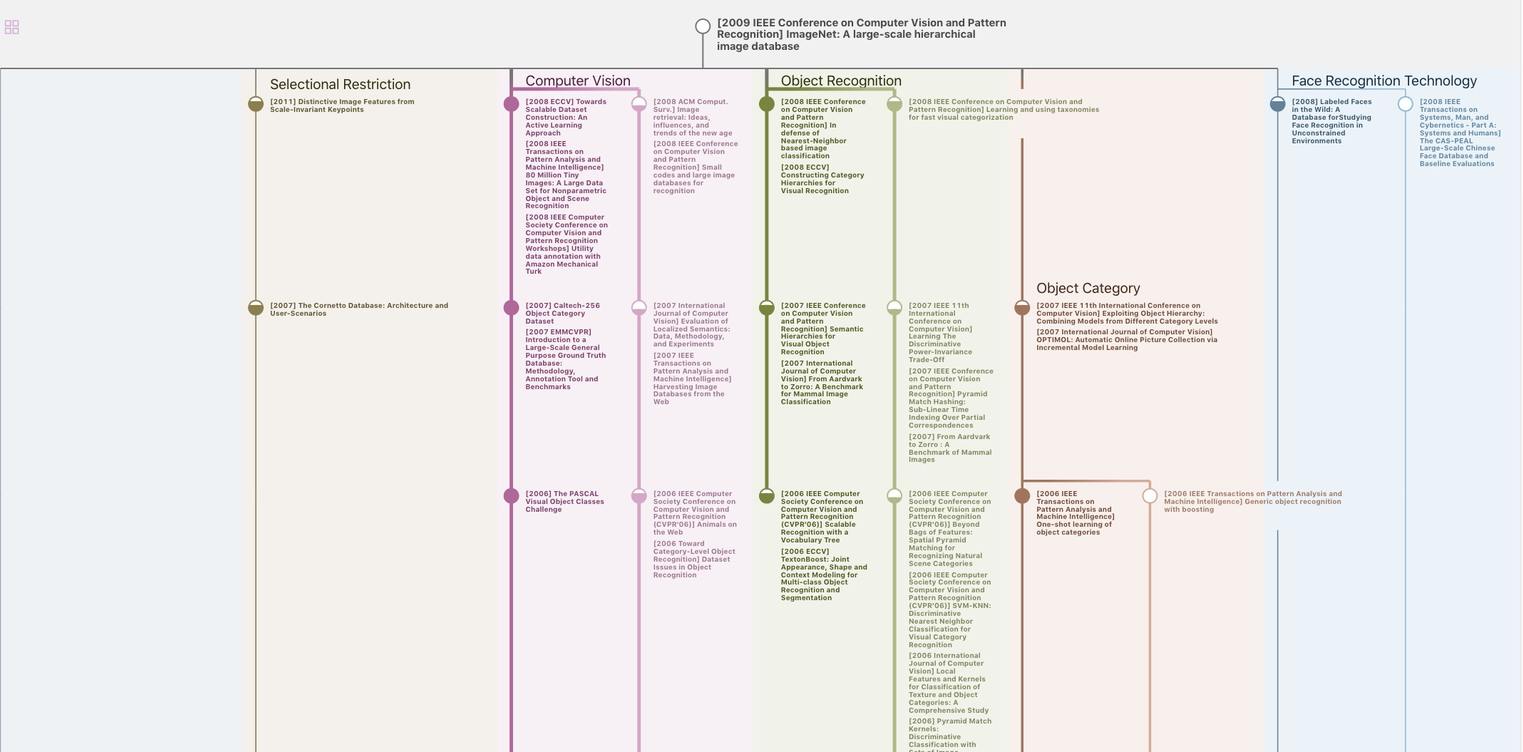
生成溯源树,研究论文发展脉络
Chat Paper
正在生成论文摘要