RUW-Net: A Dual Codec Network for Road Extraction From Remote Sensing Images
IEEE JOURNAL OF SELECTED TOPICS IN APPLIED EARTH OBSERVATIONS AND REMOTE SENSING(2024)
摘要
Road information plays an increasingly important role in applications, such as map updating, urban planning, and intelligent supervision. However, roads in remote sensing images may be shaded by trees and buildings or interfered with by farmland. These intrinsic image features can cause road extraction results to suffer from breakage and misidentification problems. To address these problems, this article improves on D-LinkNet and proposes a dual codec structure network, namely RUW-Net. Specifically, we use ReSidual U-blocks instead of ordinary residual blocks to extract more global contextual information during the encoding stage. Moreover, we propose a decoder-encoder combination (DEC) module to build a dual codec structure. The DEC module links the decoder of the first U-block and the encoder of the following U-block to narrow the semantic gap in the encoding and decoding process. The RUW-Net model can extract more multiscale contextual features and effectively use them to enhance the semantic information of road entities. Therefore, the RUW-Net model can obtain more accurate extraction results. We conducted a series of experiments on public datasets, such as DeepGlobe, including comparative, robustness, and ablation experiments. The results show that the proposed model alleviates the road extraction breakage and misidentification problems. Compared with other representative methods, the RUW-Net performs better in terms of completeness and accuracy of road extraction results; overall, its extraction results are also the best. The RUW-Net model provides a new idea for road extraction from remote sensing images.
更多查看译文
关键词
Multiscale feature,remote sensing (RS) image,road extraction,semantic segmentation
AI 理解论文
溯源树
样例
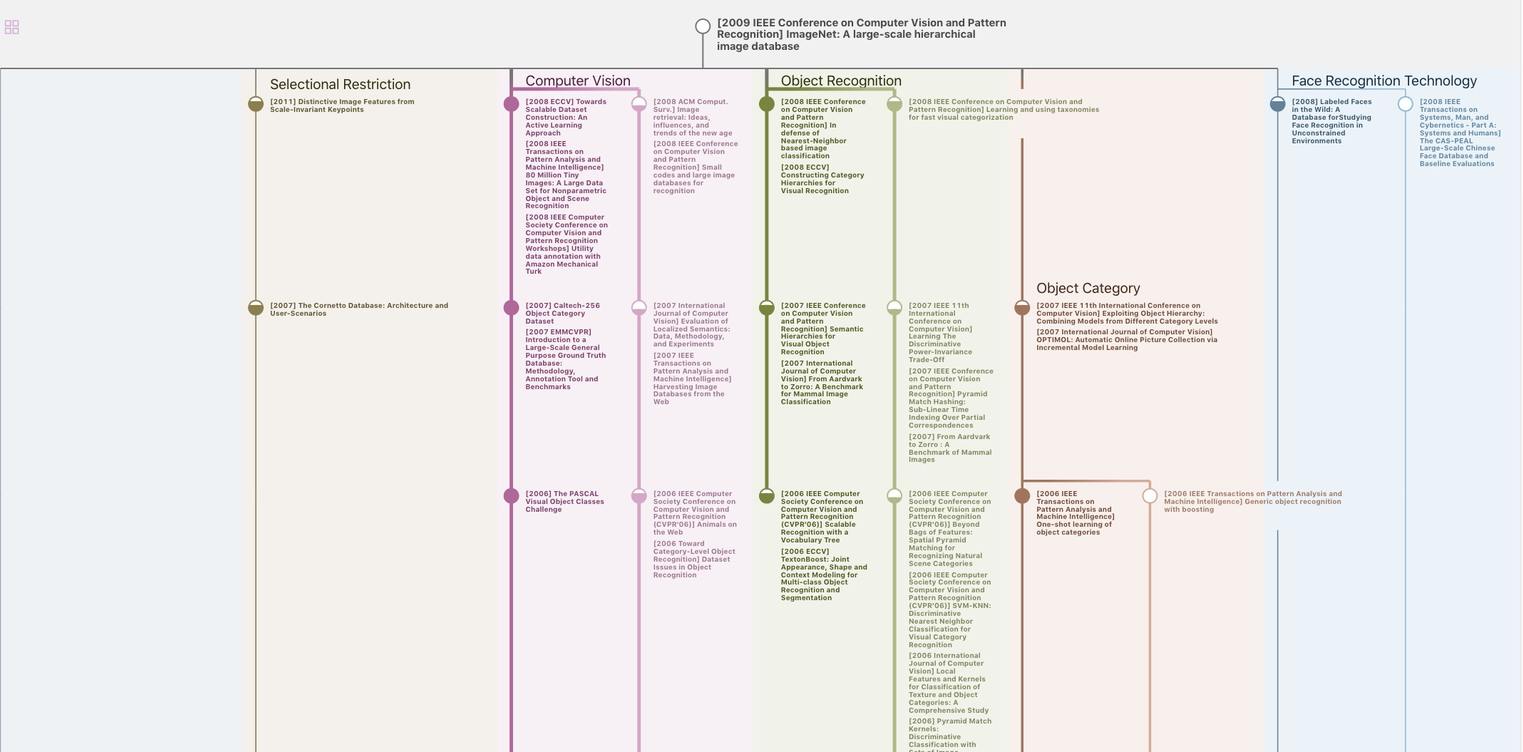
生成溯源树,研究论文发展脉络
Chat Paper
正在生成论文摘要