Predictive quality assurance for ring gantry linear accelerator using deep learning and statistical process control
2023 18th International Joint Symposium on Artificial Intelligence and Natural Language Processing (iSAI-NLP)(2023)
摘要
The Machine Performance Check (MPC) has become the standard daily procedure for QA in linear accelerators. Predictive QA has emerged as a proactive maintenance approach, aiming to address faults before they breach clinical tolerances. This study focuses on developing a predictive QA system based on MPC, utilizing deep learning techniques. A total of 154 daily MPC data points were collected. The initial 65.4% of data (100 MPC datasets) were used to train the predictive model using a combination of CNN and Long Short-Term Memory (LSTM). Warning thresholds for all MPC test parameters were generated using statistical process control. The remaining 34.6% of the data were used to evaluate the accuracy of the model. Various hyperparameters, including the window size, were optimized to enhance the effectiveness of the model. Based on the testing dataset, CNN-LSTM with a window size of 3 demonstrated the highest accuracy. The average MAE, MSE, and RMSE values were 0.0315 +/- 0.0541, 0.0014 +/- 0.0128, and 0.0374 +/- 0.0631, respectively. To proactively detect machine errors, the predictive MPC-based QA system compared next-day and trend prediction outputs for each MPC test parameter against the warning thresholds. MPC-based QA with deep learning techniques, medical physicists can engage in preventative maintenance, anticipating LINAC errors and reducing unscheduled LINAC downtime.
更多查看译文
关键词
Predictive Quality Assurance,Deep Learning,Ring gantry Linear Accelerator,Statistical Process Control,and Time Series Prediction
AI 理解论文
溯源树
样例
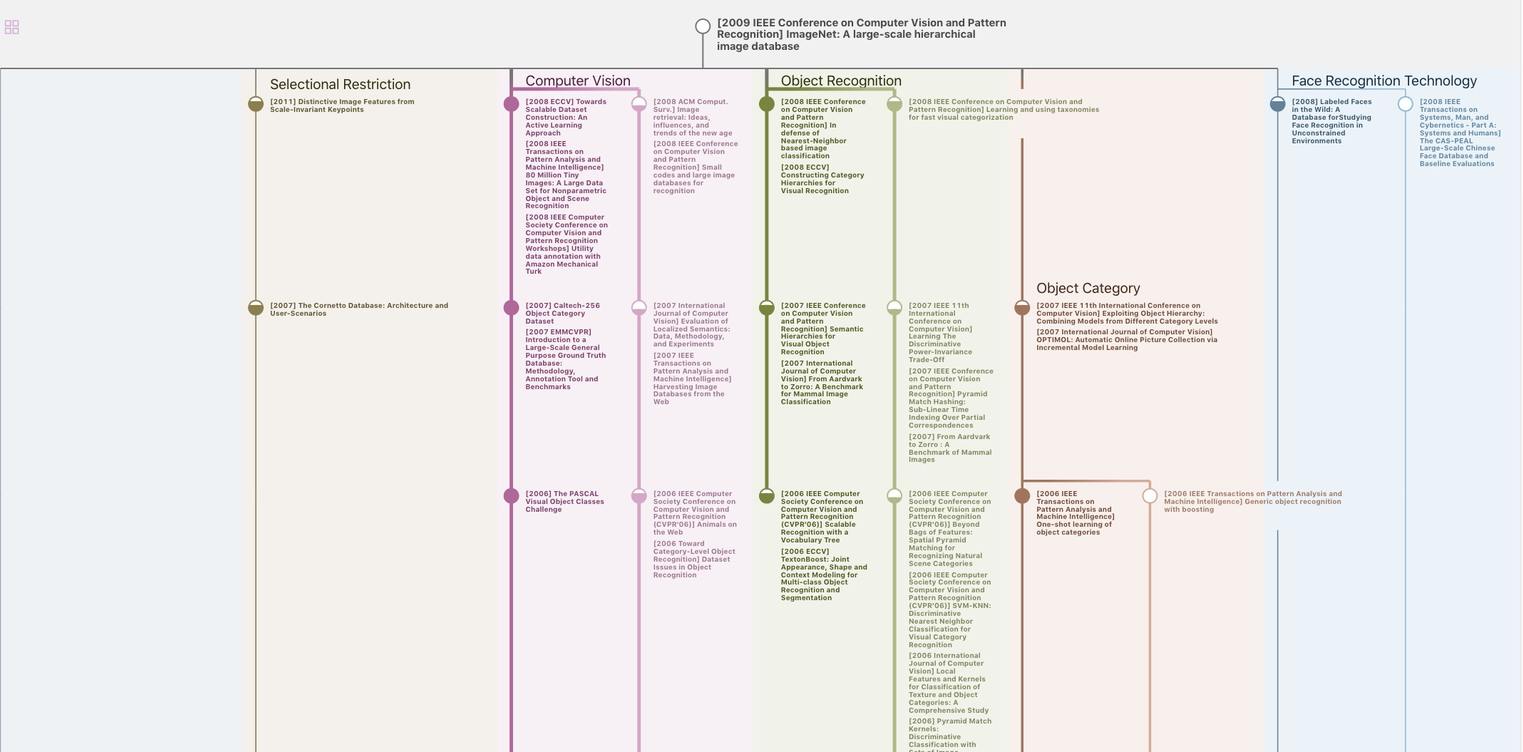
生成溯源树,研究论文发展脉络
Chat Paper
正在生成论文摘要