SOFT: Self-supervised sparse Optical Flow Transformer for video stabilization via quaternion
ENGINEERING APPLICATIONS OF ARTIFICIAL INTELLIGENCE(2024)
摘要
Video stabilization is crucial for video representation learning, which suffers from the challenges such as the perception of unstable vision, the stripping and cognition of target motion features in complex scenes, the correction of the jittery camera systems trails. In this paper, we propose a Self-supervised sparse Optical Flow Transformer (SOFT) model, consisting of a self-supervised contrastive learning transformer network, a sparse optical flow perception network and a multimodal cognitive fusion network. The SOFT model takes advantage of optical flow to estimate motion. The sparse optical flow perception network perceiving partially sparse optical flow containing motion features. This serves as the input to the self-supervised contrastive learning transformer network for generating sparse optical flow features, which are fed into the multimodal cognitive fusion network together with the real and virtual camera pose for video frame warping. Experimental comparisons with state-of-the-art models on 4 metrics demonstrate the effectiveness of the SOFT model. It achieves the best performance with an average Stability of 0.869 and average Distortion of 0.993 across 6 categories videos, which shows that the SOFT model can effectively perceive the motion in the video and smooth the jitter track of videos.
更多查看译文
关键词
Video stabilization,Transformer,Self-supervised contrastive learning,Sparse optical flow
AI 理解论文
溯源树
样例
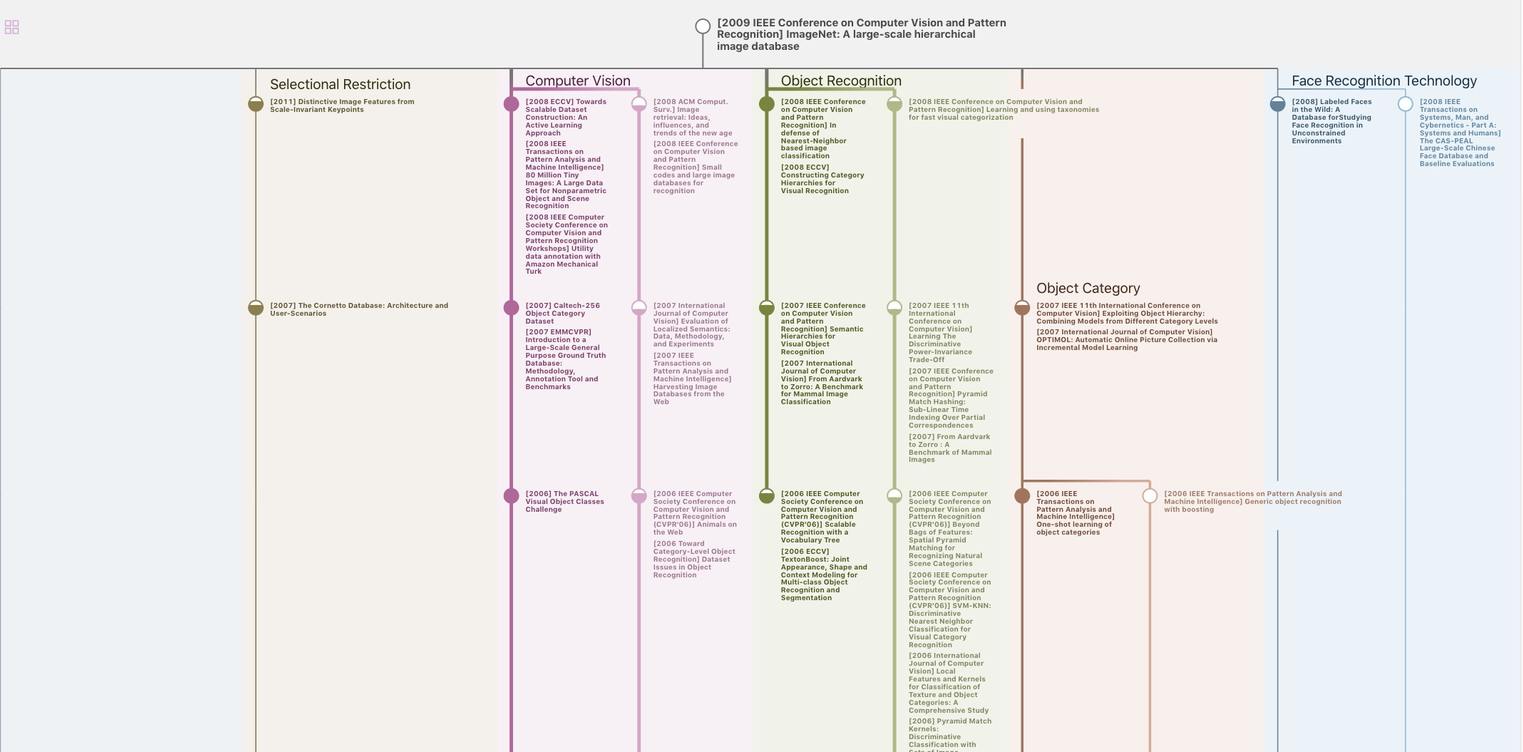
生成溯源树,研究论文发展脉络
Chat Paper
正在生成论文摘要