An interpretable deep learning strategy for effective thermal conductivity prediction of porous materials
INTERNATIONAL JOURNAL OF HEAT AND MASS TRANSFER(2024)
摘要
The effective thermal conductivity of porous materials plays a pivotal role in advancing novel thermal insulation materials. Existing theoretical prediction methods are computationally demanding with restricted applicability, and deep learning methods lack interpretability. More importantly, current approaches fail to explore the material structures themselves and leverage those insights to improve the accuracy of thermal conductivity prediction. Herein, we for the first time propose an interpretable deep learning approach with feedback for thermal conductivity prediction. A 3D convolutional neural network is trained on material structures-thermal conductivity data, and heat maps are generated from weighted feature maps to elucidate the prediction processes. Through image processing, incongruous regions are eliminated, resulting in porous structures with improved reasonability. Consequently, more accurate effective thermal conductivities can be obtained. This work may offer a novel predictive approach that refines both the material structure and the predictive model, thereby achieving more precise thermal conductivity predictions for porous materials.
更多查看译文
关键词
Porous materials,Effective thermal conductivity,Interpretable deep learning,Feedback
AI 理解论文
溯源树
样例
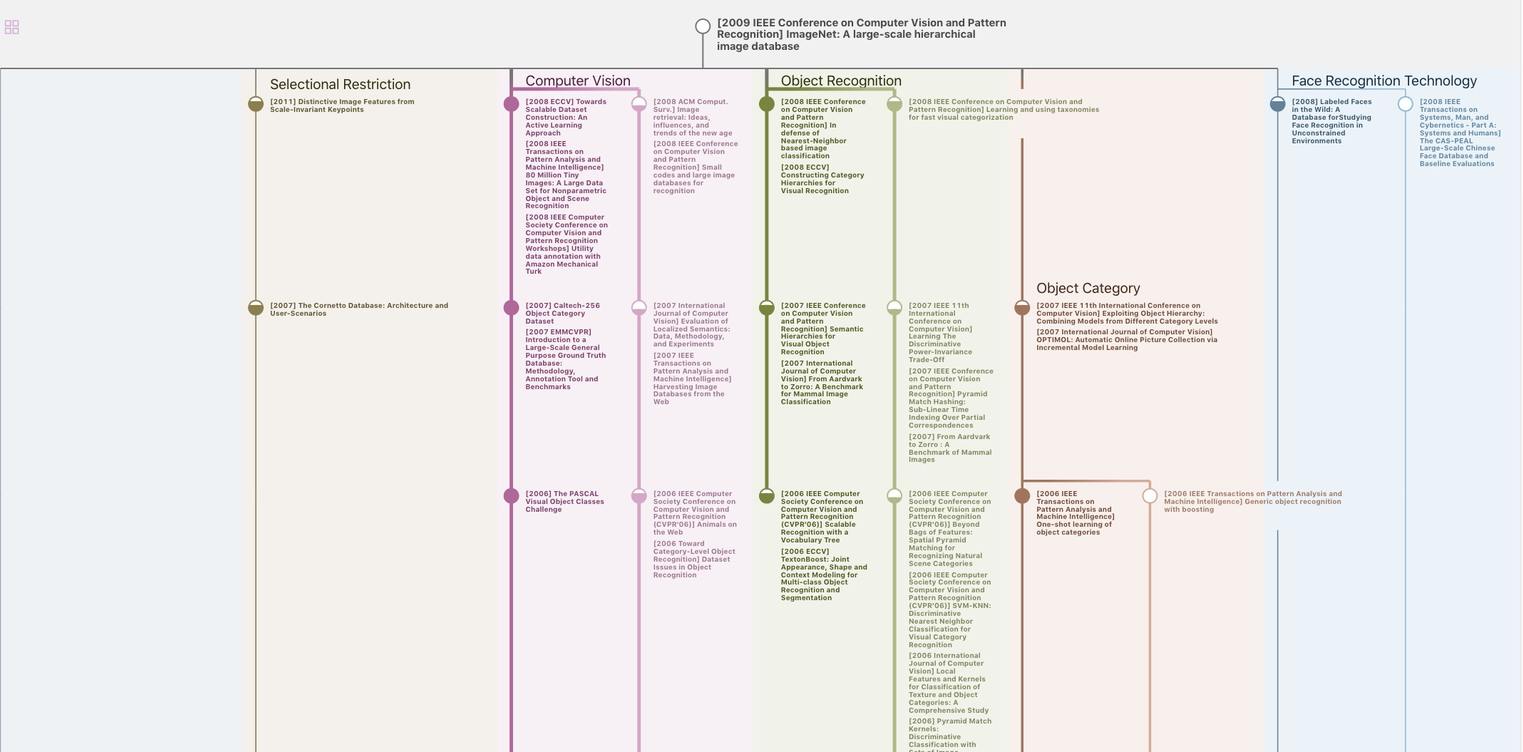
生成溯源树,研究论文发展脉络
Chat Paper
正在生成论文摘要