Clustering of Global Magnetospheric Observations
arxiv(2023)
摘要
The use of supervised methods in space science have demonstrated powerful
capability in classification tasks, but unsupervised methods have been less
utilized for the clustering of spacecraft observations. We use a combination of
unsupervised methods, being principal component analysis, self-organizing maps,
and hierarchical agglomerative clustering, to make predictions on if THEMIS and
MMS observations occurred in the magnetosphere, magnetosheath, or the solar
wind. The resulting predictions are validated visually by analyzing the
distribution of predictions and studying individual time series. Particular
nodes in the self organizing map are studied to see what data they represent.
The capability of deeper hierarchical analysis using this model is briefly
explored. Finally, the changes in region prediction can be used to infer
magnetopause and bow shock crossings, which can act as an additional method of
validation, and are saved for their utility in solar wind validation,
understanding magnetopause processes, and the potential to develop a bow shock
model.
更多查看译文
AI 理解论文
溯源树
样例
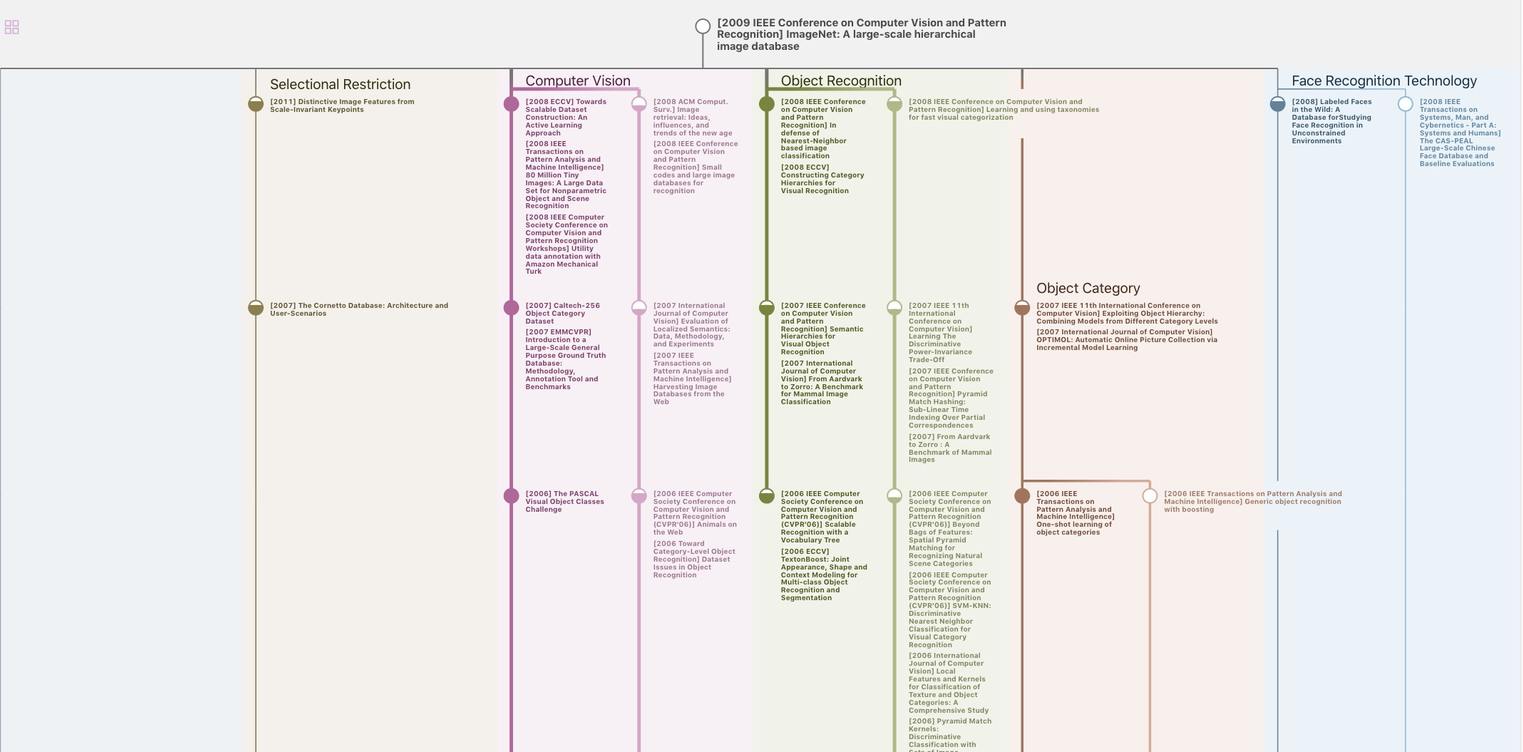
生成溯源树,研究论文发展脉络
Chat Paper
正在生成论文摘要