Fedsoda: Federated Cross-Assessment and Dynamic Aggregation for Histopathology Segmentation
IEEE International Conference on Acoustics, Speech, and Signal Processing(2024)
摘要
Federated learning (FL) for histopathology image segmentation involving multiple medical sites plays a crucial role in advancing the field of accurate disease diagnosis and treatment. However, it is still a task of great challenges due to the sample imbalance across clients and large data heterogeneity from disparate organs, variable segmentation tasks, and diverse distribution. Thus, we propose a novel FL approach for histopathology nuclei and tissue segmentation, FedSODA, via synthetic-driven cross-assessment operation (SO) and dynamic stratified-layer aggregation (DA). Our SO constructs a cross-assessment strategy to connect clients and mitigate the representation bias under sample imbalance. Our DA utilizes layer-wise interaction and dynamic aggregation to diminish heterogeneity and enhance generalization. The effectiveness of our FedSODA has been evaluated on the most extensive histopathology image segmentation dataset from 7 independent datasets. The code is available at https://github.com/yuanzhang7/FedSODA.
更多查看译文
关键词
Histopathology image segmentation,Federated learning,Medical image,Dynamic aggregation
AI 理解论文
溯源树
样例
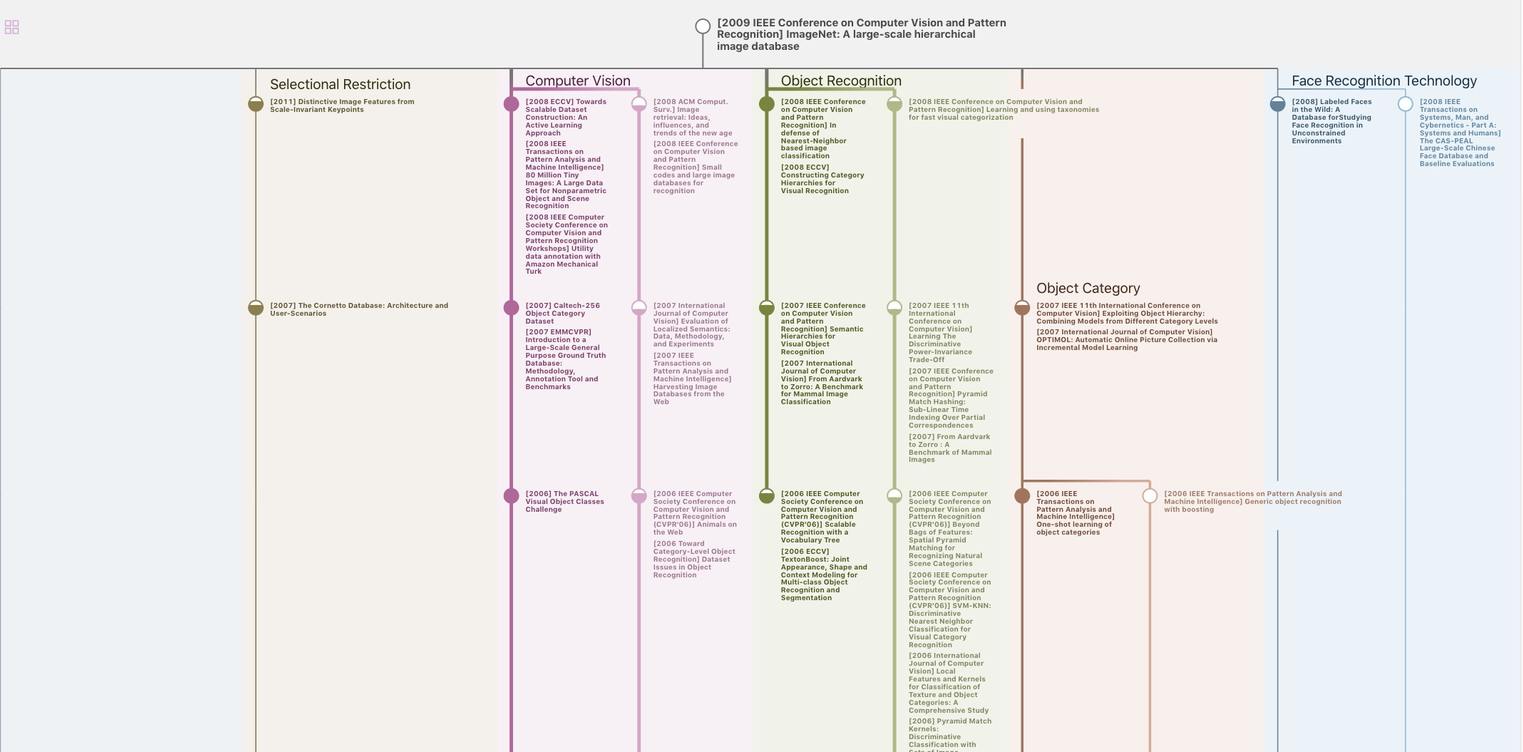
生成溯源树,研究论文发展脉络
Chat Paper
正在生成论文摘要