Uncovering associations between pre-existing conditions and COVID-19 Severity: A polygenic risk score approach across three large biobanks
PLOS GENETICS(2023)
摘要
ObjectiveTo overcome the limitations associated with the collection and curation of COVID-19 outcome data in biobanks, this study proposes the use of polygenic risk scores (PRS) as reliable proxies of COVID-19 severity across three large biobanks: the Michigan Genomics Initiative (MGI), UK Biobank (UKB), and NIH All of Us. The goal is to identify associations between pre-existing conditions and COVID-19 severity.MethodsDrawing on a sample of more than 500,000 individuals from the three biobanks, we conducted a phenome-wide association study (PheWAS) to identify associations between a PRS for COVID-19 severity, derived from a genome-wide association study on COVID-19 hospitalization, and clinical pre-existing, pre-pandemic phenotypes. We performed cohort-specific PRS PheWAS and a subsequent fixed-effects meta-analysis.ResultsThe current study uncovered 23 pre-existing conditions significantly associated with the COVID-19 severity PRS in cohort-specific analyses, of which 21 were observed in the UKB cohort and two in the MGI cohort. The meta-analysis yielded 27 significant phenotypes predominantly related to obesity, metabolic disorders, and cardiovascular conditions. After adjusting for body mass index, several clinical phenotypes, such as hypercholesterolemia and gastrointestinal disorders, remained associated with an increased risk of hospitalization following COVID-19 infection.ConclusionBy employing PRS as a proxy for COVID-19 severity, we corroborated known risk factors and identified novel associations between pre-existing clinical phenotypes and COVID-19 severity. Our study highlights the potential value of using PRS when actual outcome data may be limited or inadequate for robust analyses. In our study, we addressed a pressing issue arising from the COVID-19 pandemic, namely identifying who is vulnerable to severe complications that require hospitalization. Some pre-existing health conditions can increase risk for hospitalization, but identifying these conditions is challenging when directly relying on imperfect health records. Instead, we used genetic information to predict COVID-19 severity. By combining the risk effects of multiple genetic variants, as estimated by an external study, into a single score, we aimed to capture the predisposition to severe illness from COVID-19.We analyzed genetic data from over half a million individuals in three large biobanks and discovered connections between this genetic risk score and specific pre-existing conditions, including obesity and heart diseases. Our approach provides a way to overcome challenges in data availability and quality and offers valuable insights, even when actual COVID-19 outcomes were inconsistently or not documented. Our work lays a foundation for a better understanding of individuals at risk and emphasizes how genetics can inform public health decisions and personalized care. Ultimately, our approach showcases how to obtain key information on managing risks for not just COVID-19 but also other infectious diseases.
更多查看译文
AI 理解论文
溯源树
样例
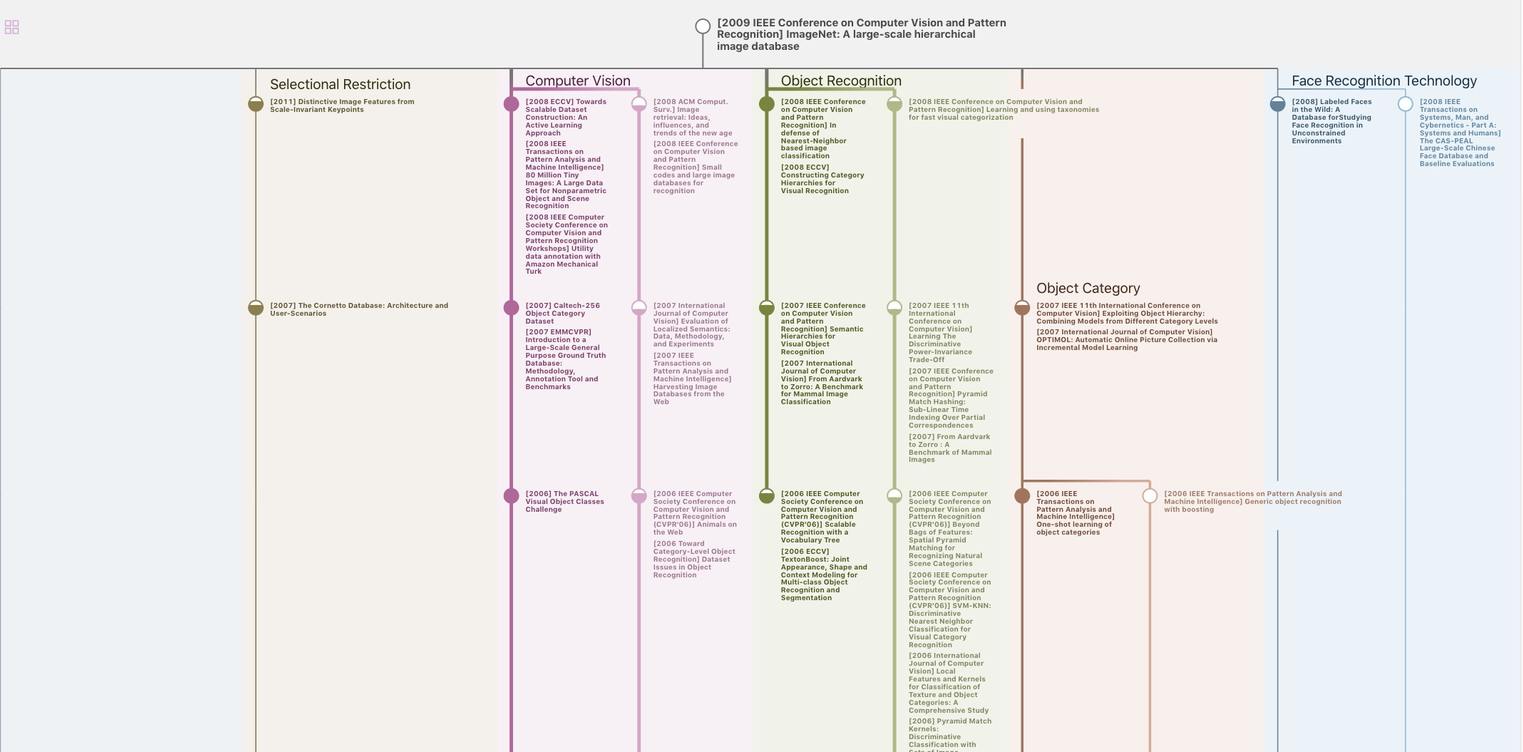
生成溯源树,研究论文发展脉络
Chat Paper
正在生成论文摘要