Restricted Latent Class Models for Nominal Response Data: Identifiability and Estimation
Psychometrika(2023)
摘要
Restricted latent class models (RLCMs) provide an important framework for diagnosing and classifying respondents on a collection of multivariate binary responses. Recent research made significant advances in theory for establishing identifiability conditions for RLCMs with binary and polytomous response data. Multiclass data, which are unordered nominal response data, are also widely collected in the social sciences and psychometrics via forced-choice inventories and multiple choice tests. We establish new identifiability conditions for parameters of RLCMs for multiclass data and discuss the implications for substantive applications. The new identifiability conditions are applicable to a wealth of RLCMs for polytomous and nominal response data. We propose a Bayesian framework for inferring model parameters, assess parameter recovery in a Monte Carlo simulation study, and present an application of the model to a real dataset.
更多查看译文
关键词
restricted latent class models,nominal response data,cognitive diagnosis model,identifiability,Bayesian
AI 理解论文
溯源树
样例
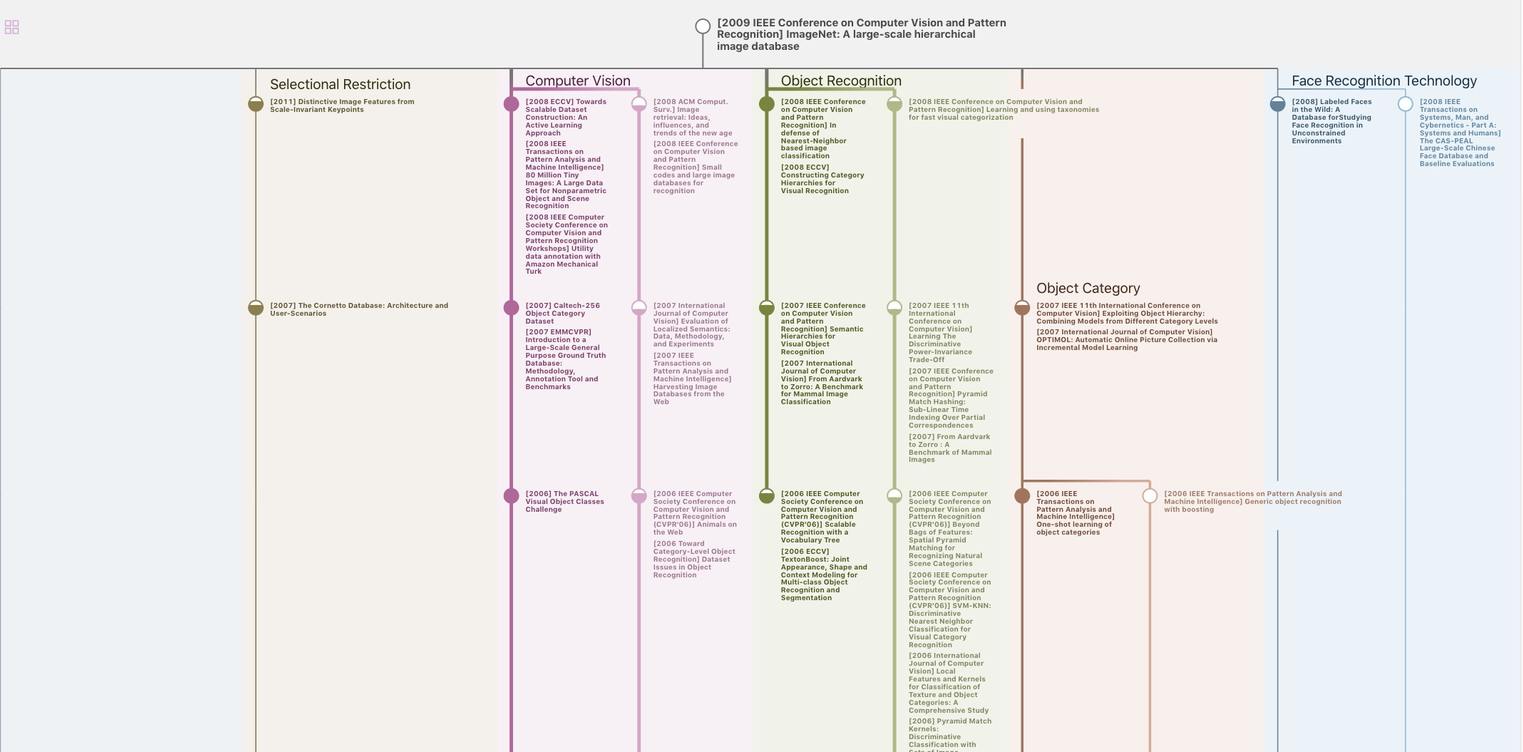
生成溯源树,研究论文发展脉络
Chat Paper
正在生成论文摘要