A robust model training strategy using hard negative mining in a weakly labeled dataset for lymphatic invasion in gastric cancer
JOURNAL OF PATHOLOGY CLINICAL RESEARCH(2024)
摘要
Gastric cancer is a significant public health concern, emphasizing the need for accurate evaluation of lymphatic invasion (LI) for determining prognosis and treatment options. However, this task is time-consuming, labor-intensive, and prone to intra- and interobserver variability. Furthermore, the scarcity of annotated data presents a challenge, particularly in the field of digital pathology. Therefore, there is a demand for an accurate and objective method to detect LI using a small dataset, benefiting pathologists. In this study, we trained convolutional neural networks to classify LI using a four-step training process: (1) weak model training, (2) identification of false positives, (3) hard negative mining in a weakly labeled dataset, and (4) strong model training. To overcome the lack of annotated datasets, we applied a hard negative mining approach in a weakly labeled dataset, which contained only final diagnostic information, resembling the typical data found in hospital databases, and improved classification performance. Ablation studies were performed to simulate the lack of datasets and severely unbalanced datasets, further confirming the effectiveness of our proposed approach. Notably, our results demonstrated that, despite the small number of annotated datasets, efficient training was achievable, with the potential to extend to other image classification approaches used in medicine.
更多查看译文
关键词
artificial intelligence,computational pathology,gastric cancer,lymphatic invasion,hard negative mining
AI 理解论文
溯源树
样例
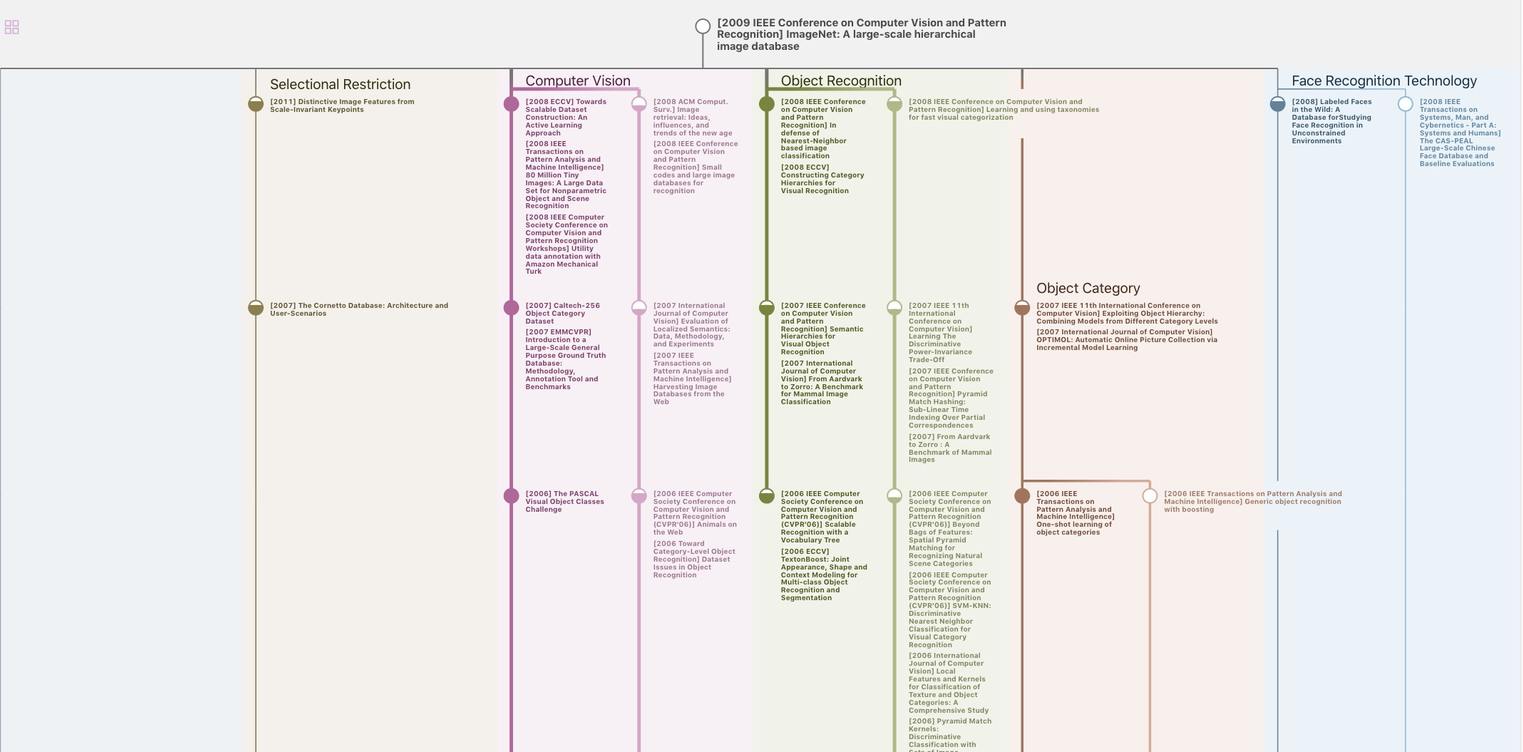
生成溯源树,研究论文发展脉络
Chat Paper
正在生成论文摘要