Landslide hazard spatiotemporal prediction based on data-driven models: Estimating where, when and how large landslide may be
INTERNATIONAL JOURNAL OF APPLIED EARTH OBSERVATION AND GEOINFORMATION(2024)
摘要
The geoscientific community primarily focuses on predicting where landslides are likely to occur through datadriven susceptibility models. Recently, few researchers have turned to statistical estimation of landslide planimetric area within a given terrain unit and exploration of the spatiotemporal distribution of landslide occurrence. However, these data-driven approaches cannot fulfill the commonly accepted definition of landslide hazard and cannot predict the location, time/frequency, and magnitude of landslide occurrence simultaneously. This study proposes a unified and data-driven framework for landslide hazard spatiotemporal modeling, enabling dynamic and probabilistic estimation of landslide occurrence within a given slope unit at a specific time period and for a specific landslide magnitude. This framework not only involves static and dynamic factors in modelling, but also considers spatial and temporal interactions to explore the spatiotemporal variation effects of landslide hazard. We test this framework on the main island of Taiwan with a multi-temporal landslide inventory from 2004 to 2018. Specifically, this framework assumes that the occurrence and size of landslides spatiotemporally follows a binomial and a Log-Gaussian distribution, respectively, and then uses generalized additive models to achieve the estimation of landslide hazard probability. Finally, the performance is validated by a spatiotemporal leave-oneout cross-validation scheme. We believe that this framework will lay the foundation for the community to estimate landslide hazard in a unified and probabilistic data-driven prototype. We envision it could lead to studies of dynamic hazard responses to climate change.
更多查看译文
关键词
Spatiotemporal landslide hazard prediction,Exceedance probability,Slope unit,Spatiotemporal cross-validation
AI 理解论文
溯源树
样例
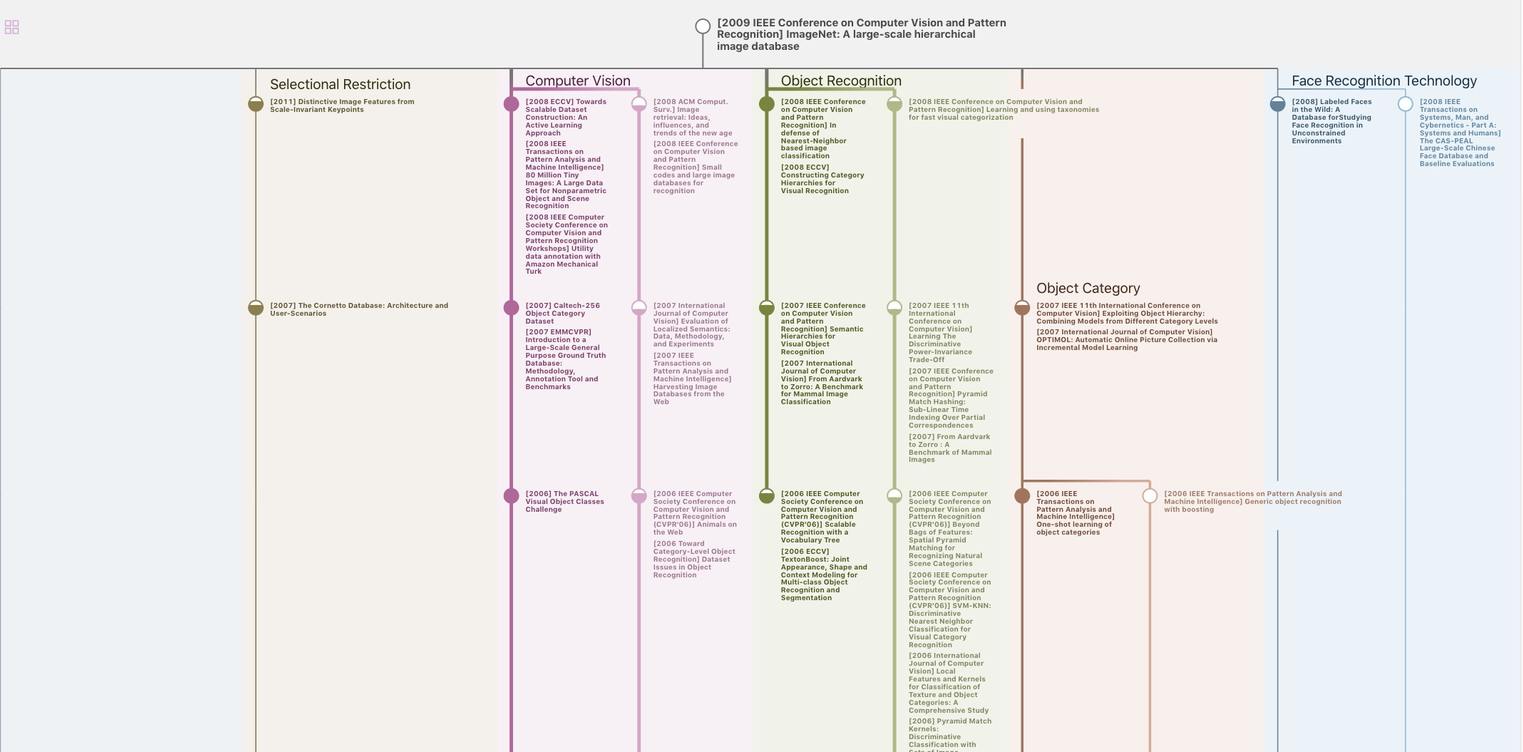
生成溯源树,研究论文发展脉络
Chat Paper
正在生成论文摘要