Adaptive Guided Convolution Generated With Spatial Relationships for Point Clouds Analysis
IEEE TRANSACTIONS ON GEOSCIENCE AND REMOTE SENSING(2024)
摘要
Representing valuable semantics in irregular 3-D point clouds with spatial-variant relationships is challenging. Current shared/weighted point convolution methods have limited efficiency and struggle to represent distinctive weight matrices precisely. In thisarticle, we present guided convolution (GuidedConv) operation, a plug-and-play point convolution operator for 3-D point cloud analysis. Compared to existing point convolution methods, GuidedConv implements a guided-share method for the weight matrices, which dynamically assigns corresponding weight matrices to points depending on their local spatial relationships without increasing the kernels in a brute-force way or equipping sophisticated auxiliary networks. The key of GuidedConv is to dynamically assigns weight matrices generated by kernel base to the points according to a guided mask, which is learned adaptively from the spatial relationships of 3-D point clouds in a data-driven manner. Guided masks establish a one-of-a-kind correspondence between the weight matrices and the points. In this way, GuidedConv possesses powerful capabilities to characterize different semantics and is more efficient and precise than current point convolution operators. Extensive qualitative and quantitative evaluations clearly show integrating GuidedConv into several relatively typical networks without modifying configurations to achieve on-par or better performances than state-of-the-art point cloud classification and segmentation methods on several benchmark datasets. Thorough ablation studies and visualizations demonstrate the flexibility and effectiveness of GuidedConv.
更多查看译文
关键词
3-D computer vision,point cloud classification,point cloud segmentation,point convolution
AI 理解论文
溯源树
样例
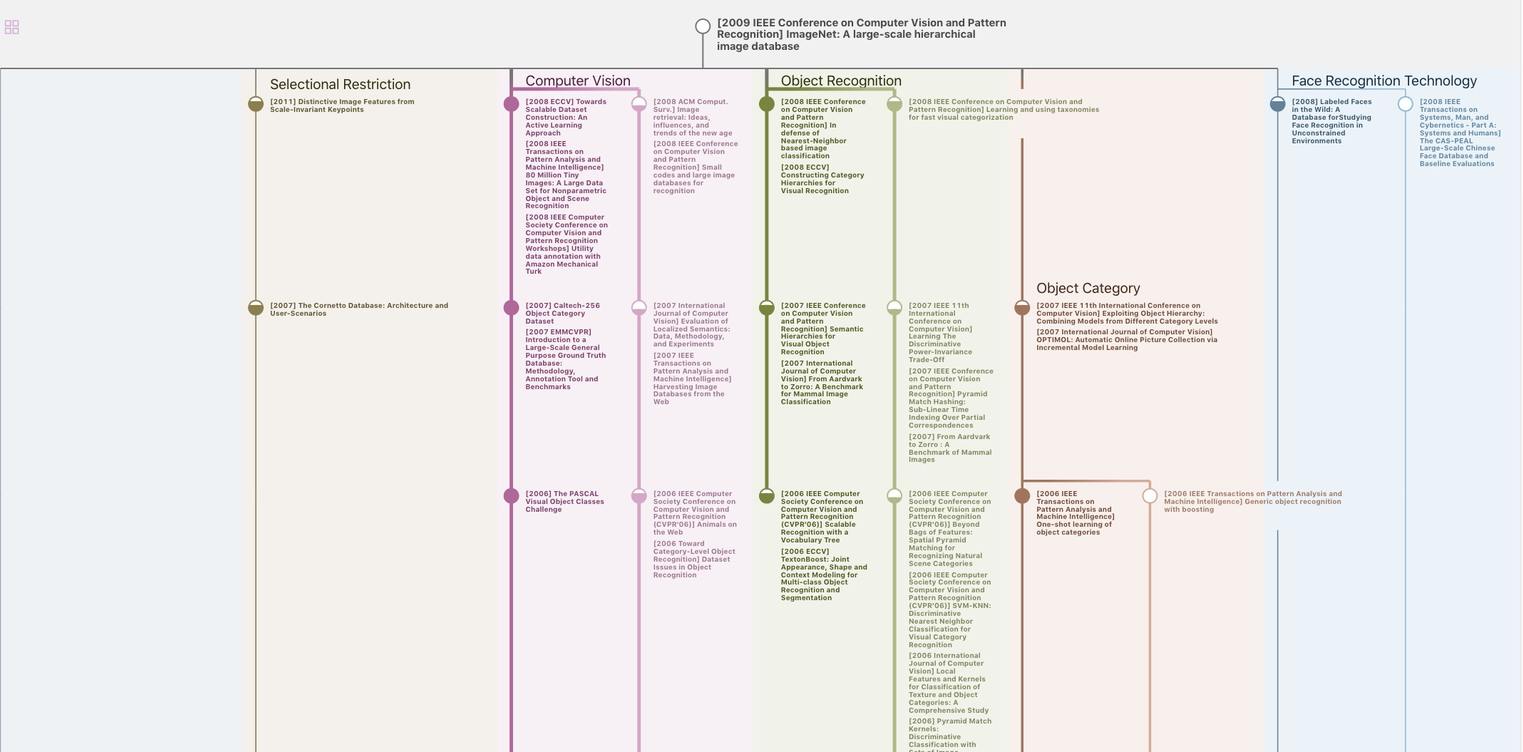
生成溯源树,研究论文发展脉络
Chat Paper
正在生成论文摘要