A Novel Label Selection Algorithm Based on Principal Component Analysis and Sparse Approximation Solution for Multi-label Classification
2023 IEEE 35TH INTERNATIONAL CONFERENCE ON TOOLS WITH ARTIFICIAL INTELLIGENCE, ICTAI(2023)
摘要
In multi-label classification, an instance may be associated with multiple labels simultaneously and thus the class labels are correlated one another. As various applications emerge, besides large instance size and high feature dimensionality, the dimensionality of label space also grows quickly, which would increase computational costs and even deteriorate classification performance. To this end, dimensionality reduction strategy is applied to label space via exploiting label correlation information, which covers label embedding and label selection techniques. Recently a lot of label embedding work has been conducted, but less attention has been paid to label selection techniques due to its difficulty. In this case, it is still an open problem how to design more effective label selection techniques for multi-label classification. Column subset selection problem (CSSP) originally is a mathematical issue in matrix theory to select a small portion of columns from a large-scale matrix for more interpretable data summarization. Therefore, such a CSSP naturally becomes an attractive mathematical representation for label selection, which is NP-hard and generally is solved via greedy strategy. In this paper, we build a two-stage label selection algorithm. At first, we apply principal component analysis (PCA) to reduce the dimensionality of the label matrix to obtain a low dimensional real matrix as the right side term in linear systems. Then, we use sparse approximation (SA) solution for linear systems to choose several informative columns from the label matrix as approximations of the low dimensional real matrix, which ultimately obtains the sub-optimal label subset of original label matrix. This new label selection method based on PCA and SA is referred to as PCASA simply. Our proposed method is validated experimentally to work well on six benchmark data sets with more than 100 labels.
更多查看译文
关键词
multi-label classification,principal component analysis,sparse approximation solution,label selection
AI 理解论文
溯源树
样例
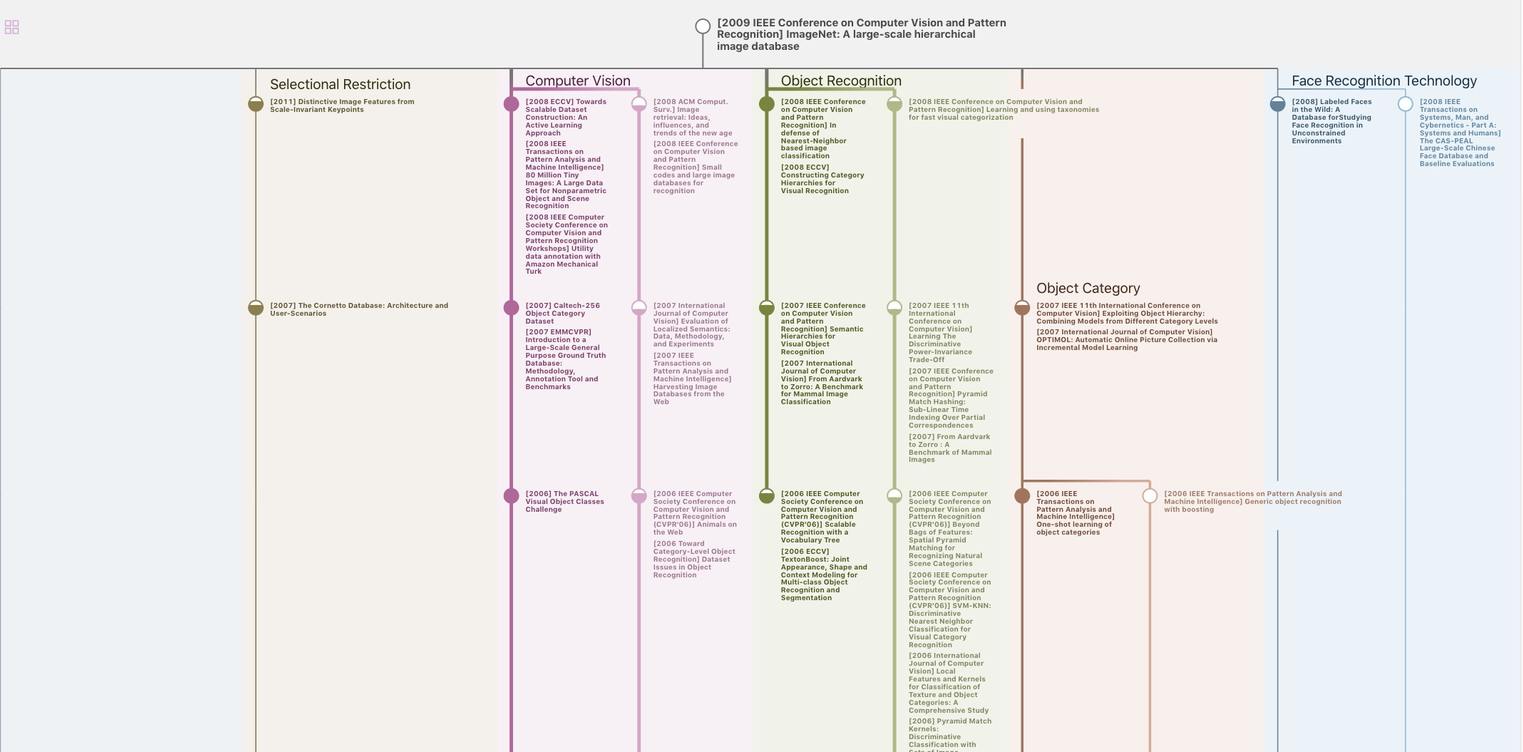
生成溯源树,研究论文发展脉络
Chat Paper
正在生成论文摘要