Early Risk Prediction of Depression Based on Social Media Posts in Arabic
2023 IEEE 35TH INTERNATIONAL CONFERENCE ON TOOLS WITH ARTIFICIAL INTELLIGENCE, ICTAI(2023)
摘要
Depression is a prevalent global health issue, impacting various aspects of individuals' lives, including home and social interactions. In the Arabic environment, the stigma surrounding mental disorders and the limited awareness in the psychiatry domain has made the early diagnosis of depression a challenging task. However, social media platforms have enabled individuals to express their thoughts and personal experiences, making these platforms a valuable resource for mental health monitoring. In this paper, we propose an approach to predict the early signs of depression utilizing posts expressed in Arabic on the Twitter platform. The proposed methodology integrates knowledge extracted using an LLM-based transformer, the UMLS medical knowledge resource, and machine learning prediction algorithms. To the best of our knowledge, this is the first research study that maps LLM-based translated texts to external medical knowledge resources to improve the accuracy of the prediction model. The proposed model consists of four phases. Firstly, NLP-based data preprocessing pipeline is employed to ensure the input dataset is in a suitable format for analysis. Secondly, the ChatGPT transformer is utilized to translate Arabic tweets into English, enabling further processing and analysis in English. Thirdly, relevant medical concepts are extracted from the translated text using the quickUMLS tool and UMLS metathesaurus, aiding in identifying important terms related to mental health. Fourthly, TF-IDF and Bag of Words (BOW) algorithms are used to assign weights to the extracted features, highlighting the significance of concepts. Finally, classification algorithms, including Support Vector Machine (SVM), Logistic Regression (LR), Random Forest (RF), Naive Bayes (NB), and Stochastic Gradient Descent (SGD), are trained using the extracted concepts. Among these classifiers, Random Forest with Bag of Words demonstrated the best performance, achieving an accuracy of 80.24%.
更多查看译文
关键词
Social Media,Depression,Prediction,UMLS,QuickUMLS,Machine Learning,Feature Extraction,TF-IDF
AI 理解论文
溯源树
样例
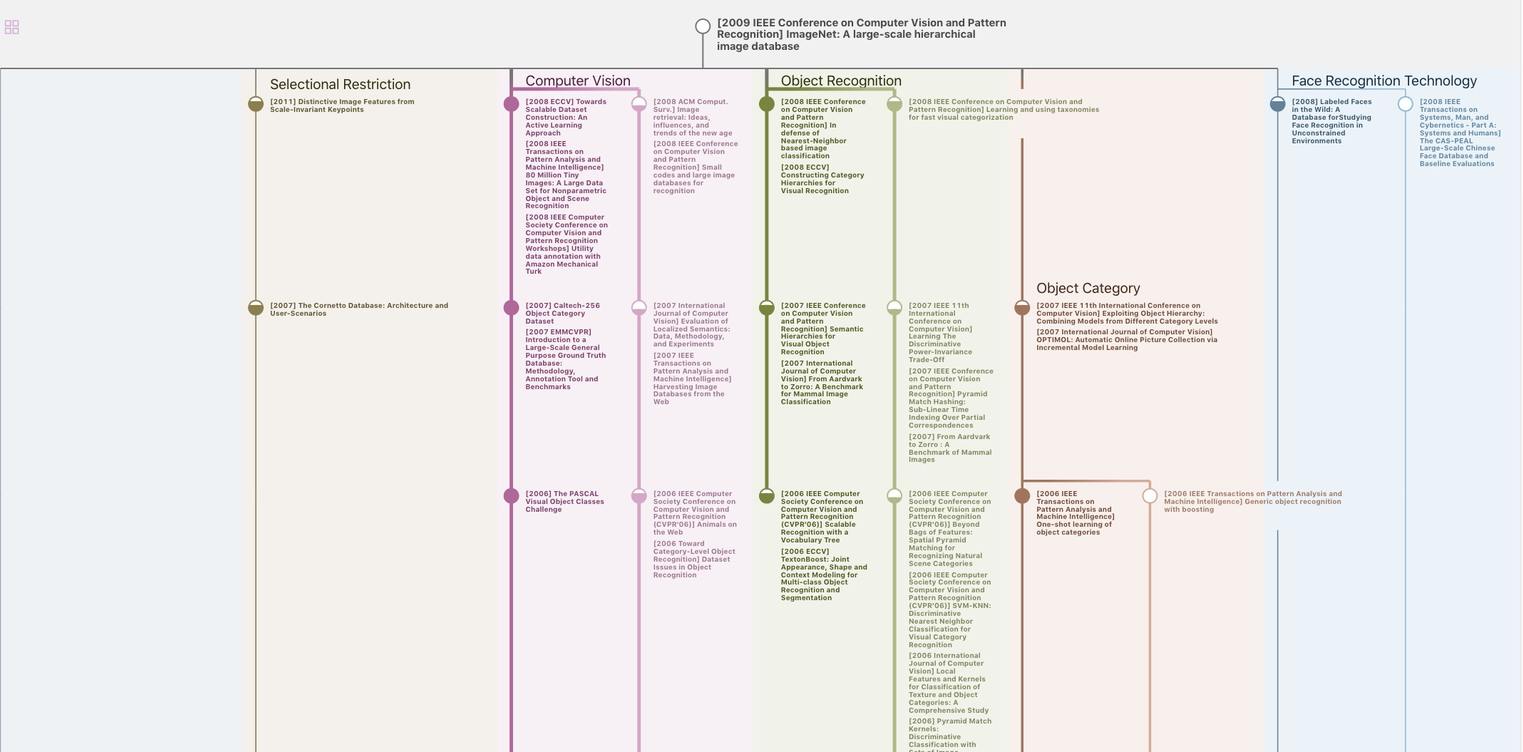
生成溯源树,研究论文发展脉络
Chat Paper
正在生成论文摘要