Data-driven approaches for sustainable agri-food: coping with sustainability and interpretability
Journal of Ambient Intelligence and Humanized Computing(2023)
摘要
Motivated by the increasing interest in machine learning algorithms for data-driven applications in agri-food addressing sustainability issues and by the ongoing discussion on the interpretability and sustainability of such algorithms, we compare congruently the performance of some state-of-the-art techniques and a new version (here proposed for the first time) of Co-Active Neuro-Fuzzy Inference System, equipped with fractional regularization (CANFIS-T for short). To this end, we consider two case studies retrieved from the literature and dealing with two approaches for sustainability development, i.e. ex-ante Life Cycle Assessment and Supply Chain Operations Reference in the agri-food context. Such approaches are set in a data-driven framework and completed by the above-mentioned machine learning techniques. The state-of-the-art techniques from the relevant literature are the ensemble ANFIS, Radial Basis Function Network and Decision Tree. The techniques are compared from the computational, interpretability and energy standpoints. From a formal perspective, we prove what negatively affects the accuracy of ensemble ANFIS. On the basis of the performed experiments, we notice that except for the ensemble ANFIS, all the approaches can be regarded as sustainable, with energy savings over 99%, while only CANFIS-T keeps both good accuracy and interpretability (with up to 4 rules) when the number of input and output variables gets large.
更多查看译文
关键词
Fuzzy sets,CANFIS,LCA,SCOR,Energy
AI 理解论文
溯源树
样例
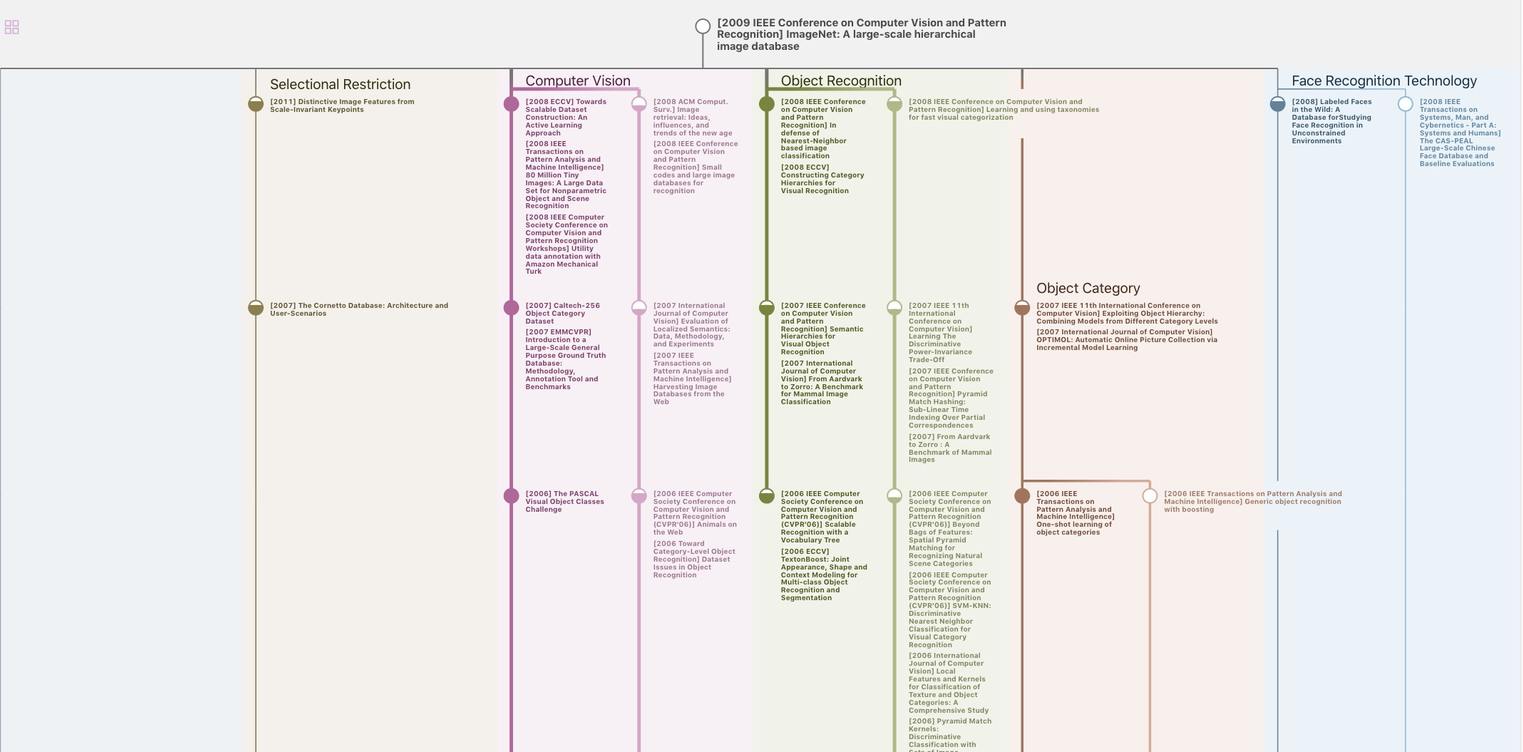
生成溯源树,研究论文发展脉络
Chat Paper
正在生成论文摘要