Classification and regression in prescriptive analytics: Development of hybrid models and an example of ship inspection by port state control
COMPUTERS & OPERATIONS RESEARCH(2024)
摘要
In prescriptive analytics, unknown quantities are involved in practical decision-making problems, and these unknown quantities need to be predicted using auxiliary data. A classic approach is to develop machine learning (ML) models to generate point estimates, which are then input to the decision making problem in a deterministic manner to prescribe the optimal decision. However, the limited quantity and inevitable errors in the auxiliary data lead to inaccurate predictions and thus sub-optimal decisions. One viable approach to addressing the above issue is to consider the uncertainties in data by inputting the conditional distributions of the unknown quantities on the auxiliary data to the optimization problem on hand, and the distributions are predicted by regression ML models. Meanwhile, it is observed that the quantitative target in some problems are discrete, and these properties are analogous to categorical targets in classification problems. Considering the fact that describing and estimating the distribution of categorical variables are much easier than quantitative variables, this study innovatively develops random forest (RF) models with regression and classification features to generate the distribution of quantitative targets that are discrete. Especially, nodes splitting criteria in the RF models is in a regression manner, while the outputs of individual decision trees and the whole RF model is in a classification manner. Numerical experiments using real port state control (PSC) inspection records and settings at the Hong Kong port are conducted to validate and compare the above prescriptive analytics approaches. The superiority of applying the newly proposed RF model into the development of prescriptive analytics approaches is also demonstrated.
更多查看译文
关键词
Data-driven decision making,Prescriptive analytics,Machine learning,Ordinal classification
AI 理解论文
溯源树
样例
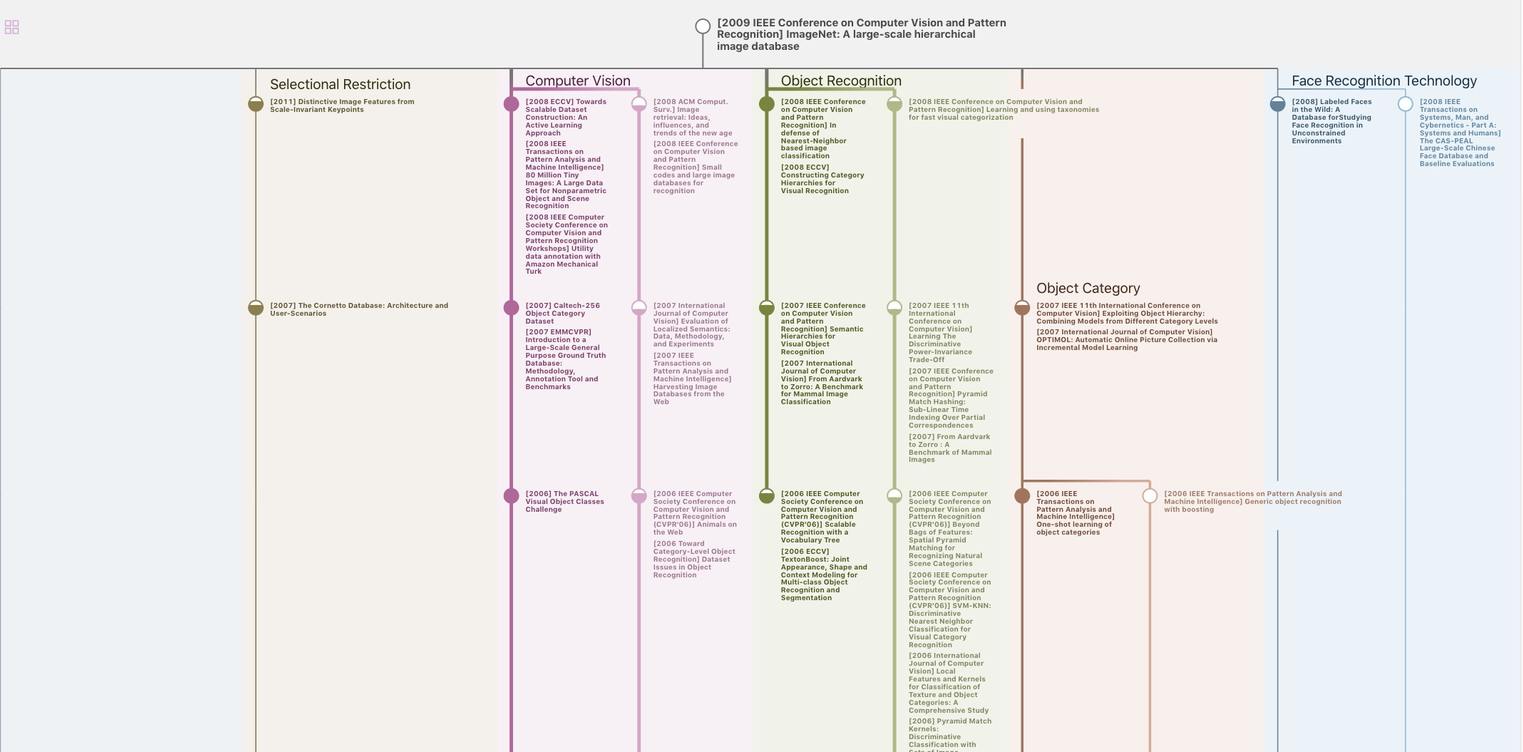
生成溯源树,研究论文发展脉络
Chat Paper
正在生成论文摘要