Combining Long and Short Memory in Time Series Models: the Role of Asymptotic Correlations of the MLEs
ECONOMETRICS AND STATISTICS(2024)
摘要
A major practical problem in the application of the long memory ARF IMA model has been distinguishing between the long memory and short memory components and subsequent estimation of the model. The asymptotic correlations between the Maximum Likelihood Estimators (MLE ) of the long memory parameter, d, and the short memory parameters within an ARF IMA estimation are derived. The correlation in an ARF IMA(1, d, 0) model can be as large as -0. 95. However, MLE still works well in these high correlation cases; even for non stationary situations. Similarly, QMLE also performs well in simulations where the innovations have t or skewed t densities. MLE also performs very well for ARF IMA (3 , d, 0) models when there is a moderate level of persistence in the short memory part. However, MLE can perform extremely poorly when there is a combination of long memory and substantial persistence in the short memory component. Some of these points are illustrated in the analysis of some Realized Volatility time series which contain long memory. The correlation in "gap ARF IMA" models is comparatively low which indicates that MLE is quite stable in this case. Some suggestions and recommendations for applied work are provided.(c) 2022 EcoSta Econometrics and Statistics. Published by Elsevier B.V. All rights reserved.
更多查看译文
关键词
Long memory,ARFIMA,Strong persistence in short memory,Correlations between MLEs of parameters
AI 理解论文
溯源树
样例
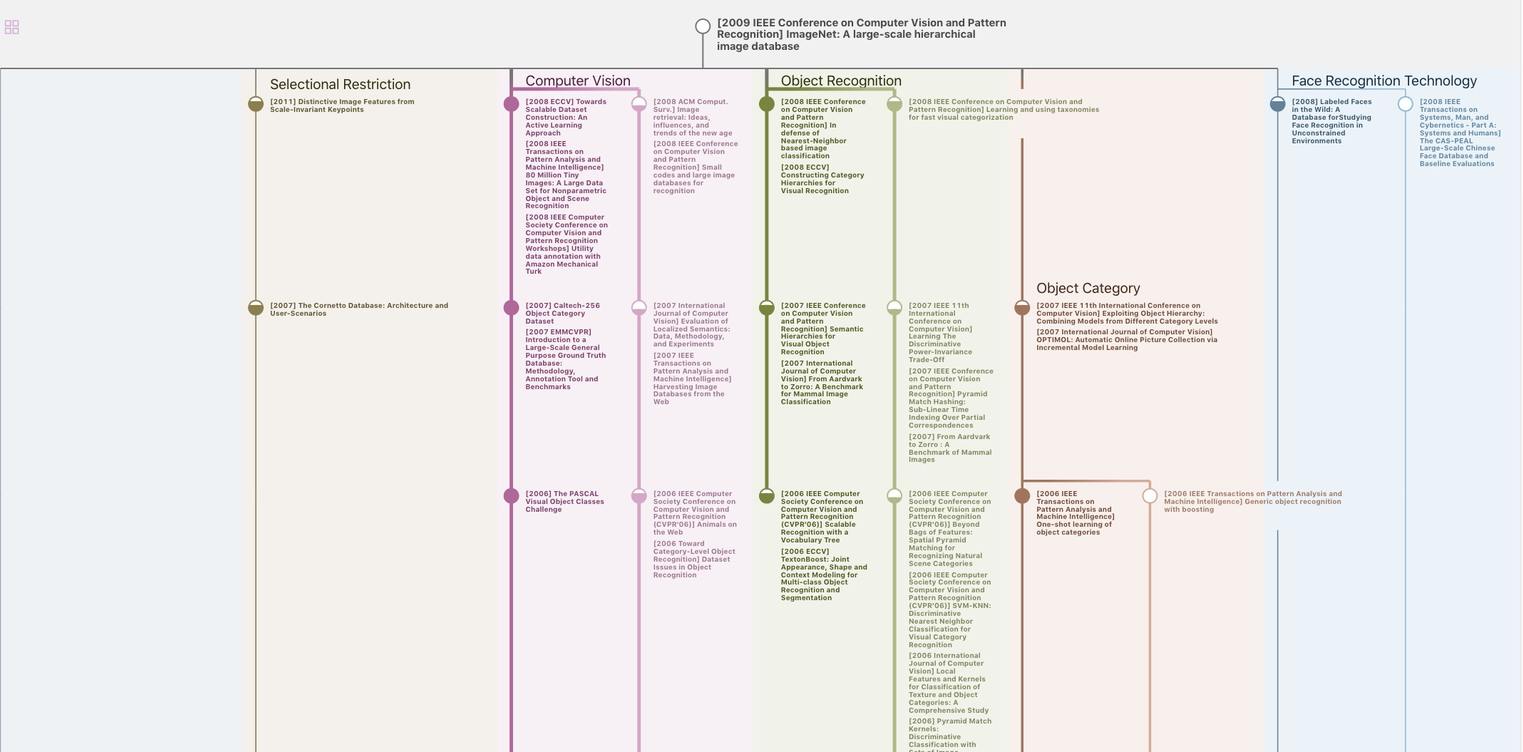
生成溯源树,研究论文发展脉络
Chat Paper
正在生成论文摘要