Generic dual-phase classification models through deep learning semantic segmentation method and image gray-level optimization
SCRIPTA MATERIALIA(2024)
摘要
Two generic deep learning models for automatic classification of dual-phase microstructures were constructed through the semantic segmentation method of DeepLab v3+, based on a small data including 6 SEM images of the U-2Nb alloys with dual-phase microstructure and the corresponding ground truth. One model is suitable for directly classifying images with different gray-level ranges and has a good average classifying accuracy. Although the other model is only suitable for a specific gray-level range, but it cost less for training and can realize a higher classifying accuracy than the former model combined with optimal gray-level adjustment for images. The two DL models were applied to classify dual-phase microstructures of different morphologies and materials, including the isothermal cooling and continuous cooling microstructures of U-2Nb alloys, and the dual-phase steel microstructure, the results of which manifest that both the two models have high classification accuracy and excellent general applicability.
更多查看译文
关键词
Dual-phase,Microstructure classification,Semantic segmentation,Deep learning,Gray-level range
AI 理解论文
溯源树
样例
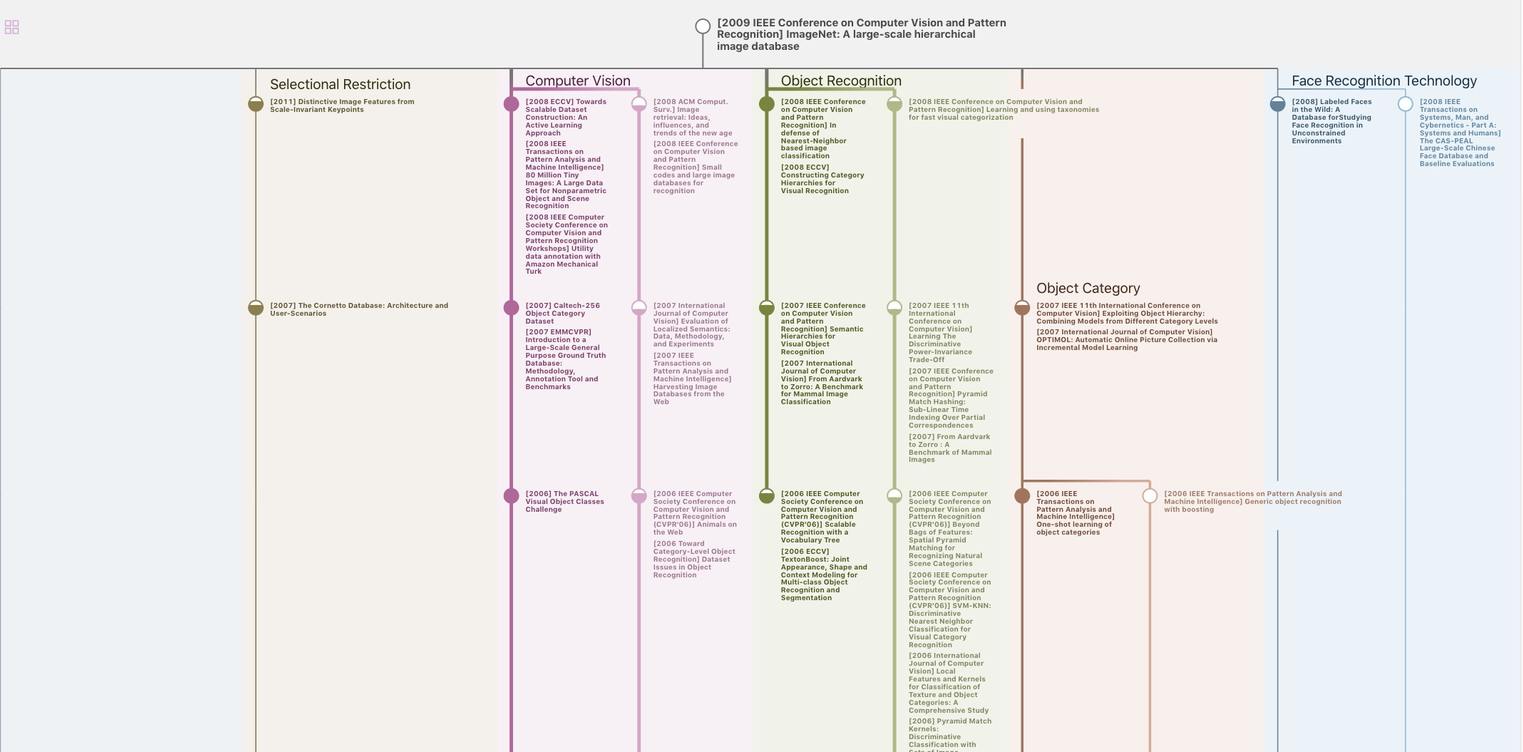
生成溯源树,研究论文发展脉络
Chat Paper
正在生成论文摘要