Meta-Learning with Versatile Loss Geometries for Fast Adaptation Using Mirror Descent
CoRR(2023)
摘要
Utilizing task-invariant prior knowledge extracted from related tasks,
meta-learning is a principled framework that empowers learning a new task
especially when data records are limited. A fundamental challenge in
meta-learning is how to quickly "adapt" the extracted prior in order to train a
task-specific model within a few optimization steps. Existing approaches deal
with this challenge using a preconditioner that enhances convergence of the
per-task training process. Though effective in representing locally a quadratic
training loss, these simple linear preconditioners can hardly capture complex
loss geometries. The present contribution addresses this limitation by learning
a nonlinear mirror map, which induces a versatile distance metric to enable
capturing and optimizing a wide range of loss geometries, hence facilitating
the per-task training. Numerical tests on few-shot learning datasets
demonstrate the superior expressiveness and convergence of the advocated
approach.
更多查看译文
关键词
Meta-learning,bilevel optimization,mirror descent,loss geometries
AI 理解论文
溯源树
样例
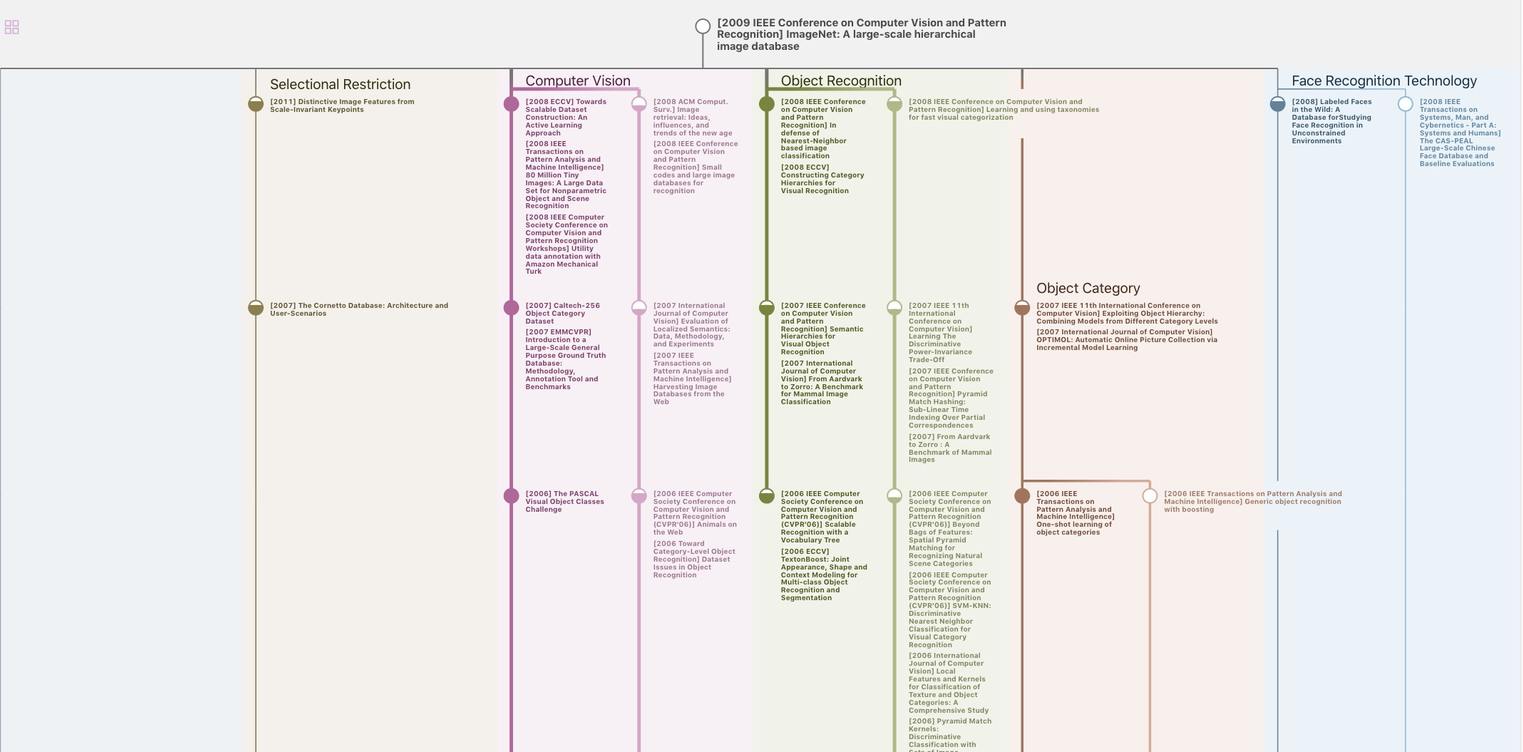
生成溯源树,研究论文发展脉络
Chat Paper
正在生成论文摘要