Quasi-Probabilistic Readout Correction of Mid-Circuit Measurements for Adaptive Feedback via Measurement Randomized Compiling
arxiv(2023)
摘要
Quantum measurements are a fundamental component of quantum computing.
However, on modern-day quantum computers, measurements can be more error prone
than quantum gates, and are susceptible to non-unital errors as well as
non-local correlations due to measurement crosstalk. While readout errors can
be mitigated in post-processing, it is inefficient in the number of qubits due
to a combinatorially-large number of possible states that need to be
characterized. In this work, we show that measurement errors can be tailored
into a simple stochastic error model using randomized compiling, enabling the
efficient mitigation of readout errors via quasi-probability distributions
reconstructed from the measurement of a single preparation state in an
exponentially large confusion matrix. We demonstrate the scalability and power
of this approach by correcting readout errors without the need for any matrix
inversion on a large number of different preparation states applied to a
register of a eight superconducting transmon qubits. Moreover, we show that
this method can be extended to measurement in the single-shot limit using
quasi-probabilistic error cancellation, and demonstrate the correction of
mid-circuit measurement errors on an ancilla qubit used to detect and actively
correct bit-flip errors on an entangled memory qubit. Our approach paves the
way for performing an assumption-free correction of readout errors on large
numbers of qubits, and offers a strategy for correcting readout errors in
adaptive circuits in which the results of mid-circuit measurements are used to
perform conditional operations on non-local qubits in real time.
更多查看译文
AI 理解论文
溯源树
样例
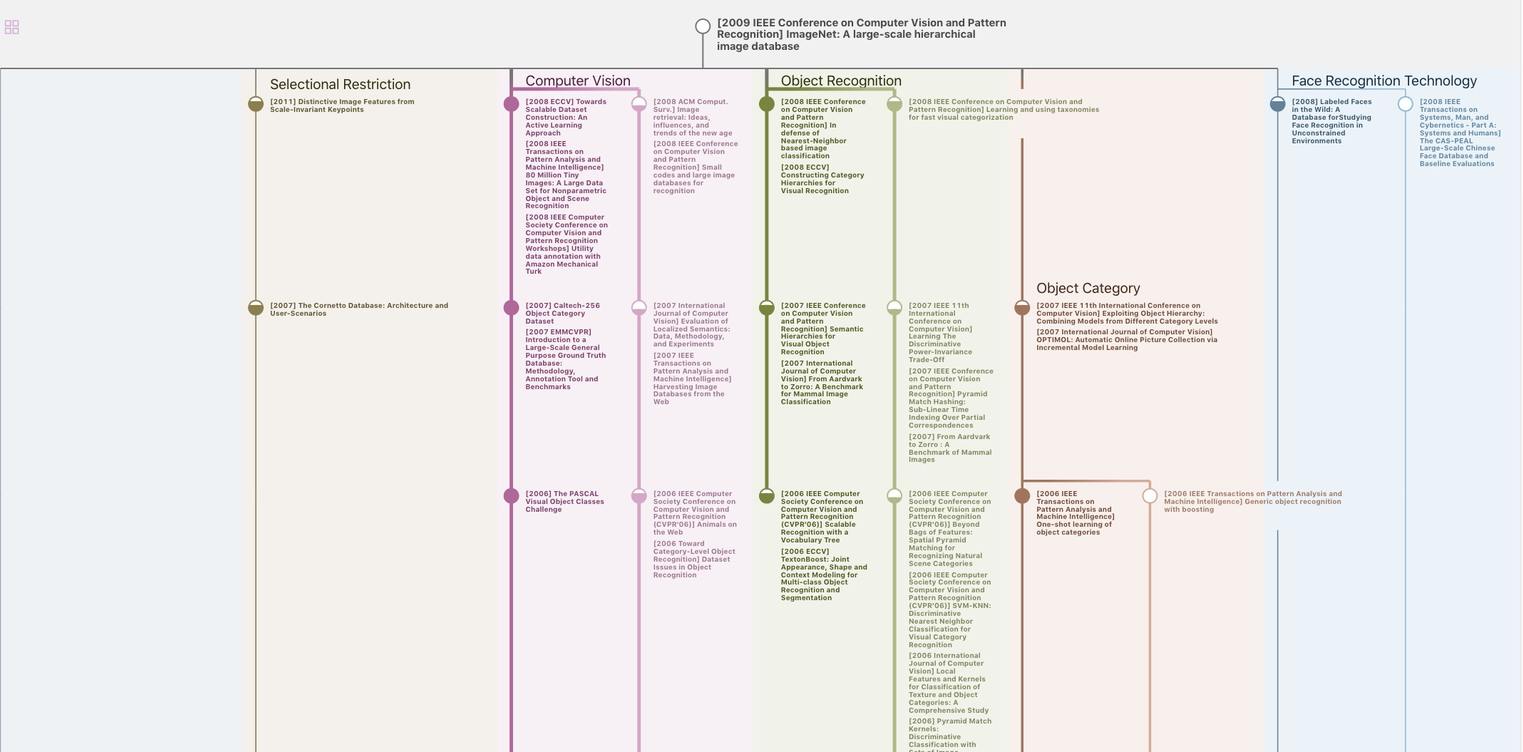
生成溯源树,研究论文发展脉络
Chat Paper
正在生成论文摘要