Performance of Large Intelligent Surfaces in Multiuser Millimeter Wave MIMO-NOMA Systems
IEEE ACCESS(2023)
摘要
Large Intelligent Surfaces (LIS) are considered a candidate technology for 6G networks due to its simple deployment and relatively low cost. Recently, Millimeter Wave (mmWave) communication has attracted much attention due to its huge spectrum resources (30-300 GHz), which supports gigabits per second data rates. However, it suffers from severe path loss compared to path attenuation at low frequency bands. Massive MIMO compensates this path loss by adding more antennas in a small area. Non-Orthogonal Multiple Access (NOMA) is one of the multiple access techniques that increases the data rate of the system. In this paper, we integrate LIS with multi-user MIMO-NOMA in the mmWave band to enhance the overall system performance. Hybrid precoding is performed by having a zero-forcing digital precoder at the base station and an analog precoding at the LIS. Users are grouped into clusters to minimize multi-user interference. Joint optimization of power allocation of NOMA users, LIS phases, and gains is proposed to maximize the energy efficiency of the system. Closed form expressions for the optimum phases and gains of LIS are obtained using the Quadratic Constraint Quadratic Problem (QCQP). Due to the non-convexity of the formulated problem, alternating optimization is developed to solve the problem. Subsequently, the optimization parameters are decoupled through applying fractional programming. The problem is divided into sub problems solved alternatively. The performance of the system is evaluated in terms of energy efficiency and power consumption. Numerical results show the superior performance of LIS at the expense of higher power consumption.
更多查看译文
关键词
NOMA,Millimeter wave communication,Precoding,Energy efficiency,Optimization,Spectral efficiency,Power demand,Large intelligent surface (LIS),millimeter wave (mmWave) communication,MIMO,passive RIS,active RIS,spectral efficiency,energy efficiency,joint optimization
AI 理解论文
溯源树
样例
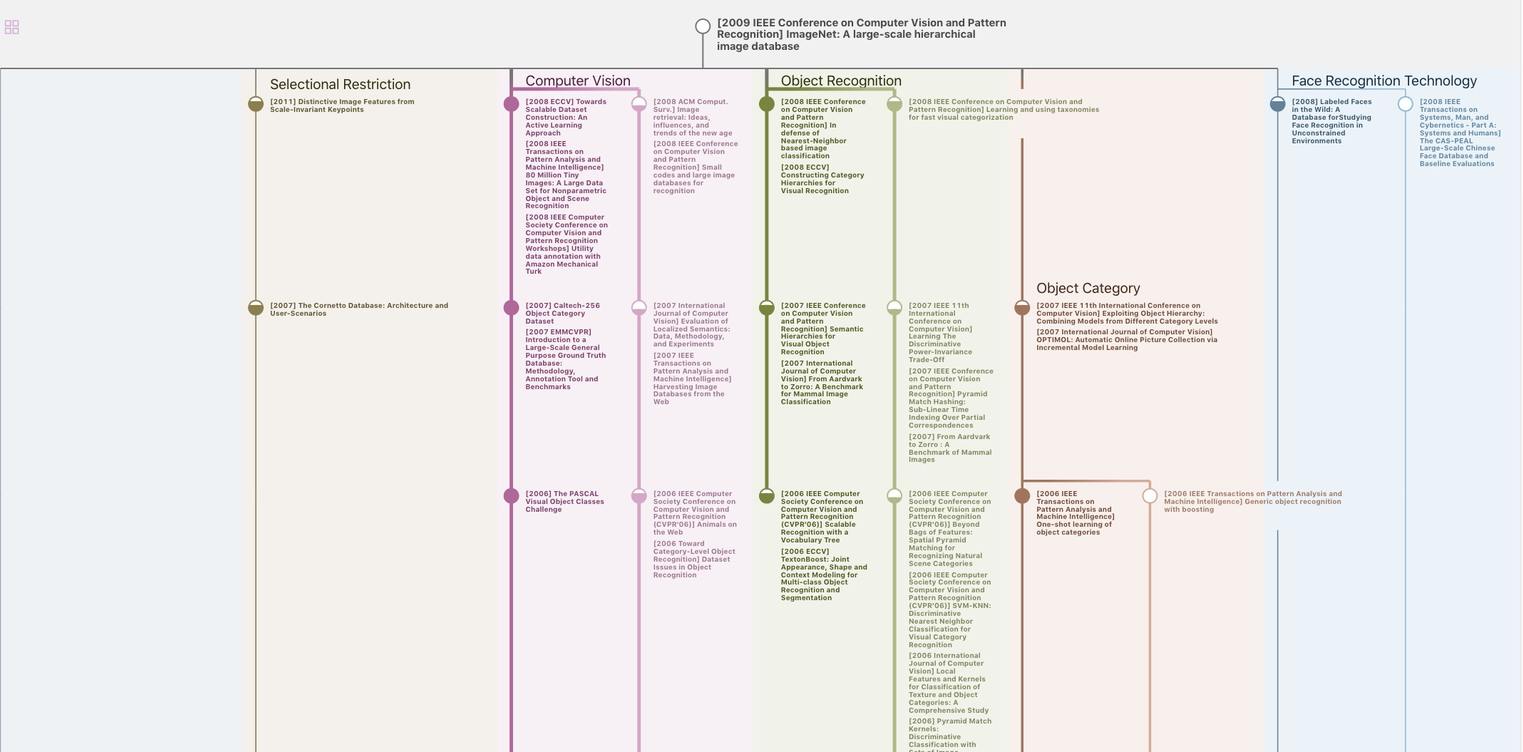
生成溯源树,研究论文发展脉络
Chat Paper
正在生成论文摘要