Local navigation-like functions for safe robot navigation in bounded domains with unknown convex obstacles
AUTOMATICA(2024)
摘要
In this paper, we propose a controller that stabilizes a holonomic robot with single-integrator dynamics to a target position in a bounded domain, while preventing collisions with convex obstacles. We assume that the robot can measure its own position and heading in a global coordinate frame, as well as its relative position vector to the closest point on each obstacle in its sensing range. The robot has no information about the locations and shapes of the obstacles. We define regions around the boundaries of the obstacles and the domain within which the robot can sense these boundaries, and we associate each region with a virtual potential field that we call a local navigation-like function (NLF), which is only a function of the robot's position and its distance from the corresponding boundary. We also define an NLF for the remaining free space of the domain, and we identify the critical points of the NLFs. Then, we propose a switching control law that drives the robot along the negative gradient of the NLF for the obstacle that is currently closest, or the NLF for the remaining free space if no obstacle is detected. We derive a conservative upper bound on the tunable parameter of the NLFs that guarantees the absence of locally stable equilibrium points, which can trap the robot, if the obstacles' boundaries satisfy a minimum curvature condition. We also analyze the convergence and collision avoidance properties of the switching control law and, using a Lyapunov argument, prove that the robot safely navigates around the obstacles and converges asymptotically to the target position. We validate our analytical results for domains with different obstacle configurations by implementing the controller in both numerical simulations and physical experiments with a nonholonomic mobile robot.(c) 2023 Elsevier Ltd. All rights reserved.
更多查看译文
关键词
Robot navigation,Obstacle avoidance,Virtual potential field,Switching control
AI 理解论文
溯源树
样例
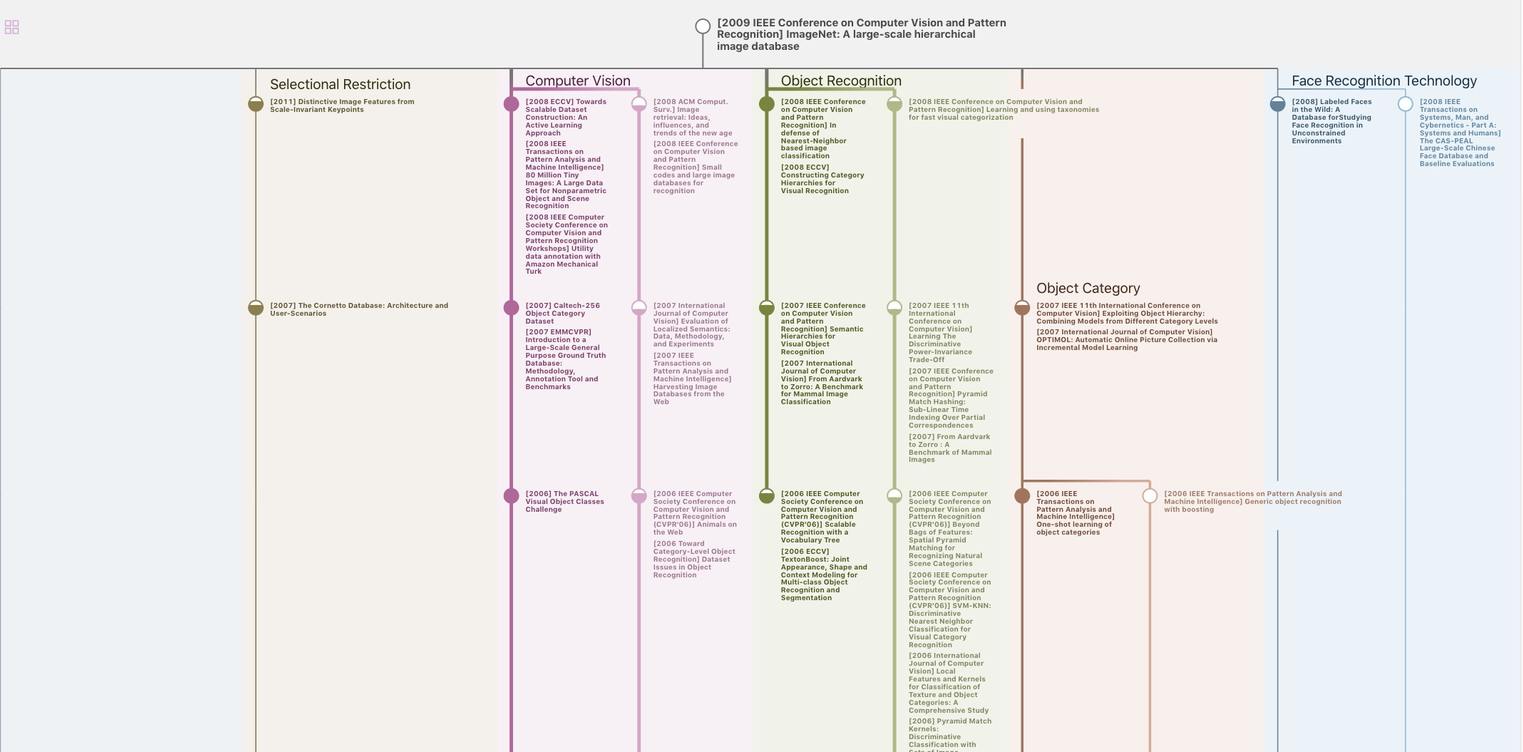
生成溯源树,研究论文发展脉络
Chat Paper
正在生成论文摘要