MGCRL: Multi-view graph convolution and multi-agent reinforcement learning for dialogue state tracking
Neural Computing and Applications(2024)
摘要
Dialogue state tracking (DST) is a significant part of prevalent task-oriented dialogue systems, which monitor the user’s goals based on current and previous dialogues for effective dialogue management. However, most existing approaches train DST on a single domain, ignoring the information across domains, and thus relevant slots in each domain must learn to cooperate. This paper deals this challenge by introducing a multi-view graph convolution and multi-agent reinforcement learning (MGCRL) method to help each domain-specific slot to learn to cooperate. Specifically, first, a multi-view graph is presented to provide more related information to transfer structured features among domain-specific slots across various domains. Compared with a single-view graph, multi-view has better complementarity, incorporating additional graphs for learning multiple types of association, thus improving the cross-domain information-sharing capability of DST models. On this basis, a multi-agent reinforcement learning method is presented to train DST models. Compared to current reinforcement learning methods used in DST models, the proposed multi-agent reinforcement learning utilizing multi-view graph convolutional networks allows each agent to learn to cooperate in multi-agent environments. Experimental results on the widely-used datasets MultiWOZ (Multi-Domain Wizard-of-Oz) 2.0/2.1 demonstrate that the proposed MGCRL method achieves a higher joint goal accuracy than the existing state-of-the-art DST models.
更多查看译文
关键词
Dialogue state tracking,Dialogue management,Graph convolution,Reinforcement learning
AI 理解论文
溯源树
样例
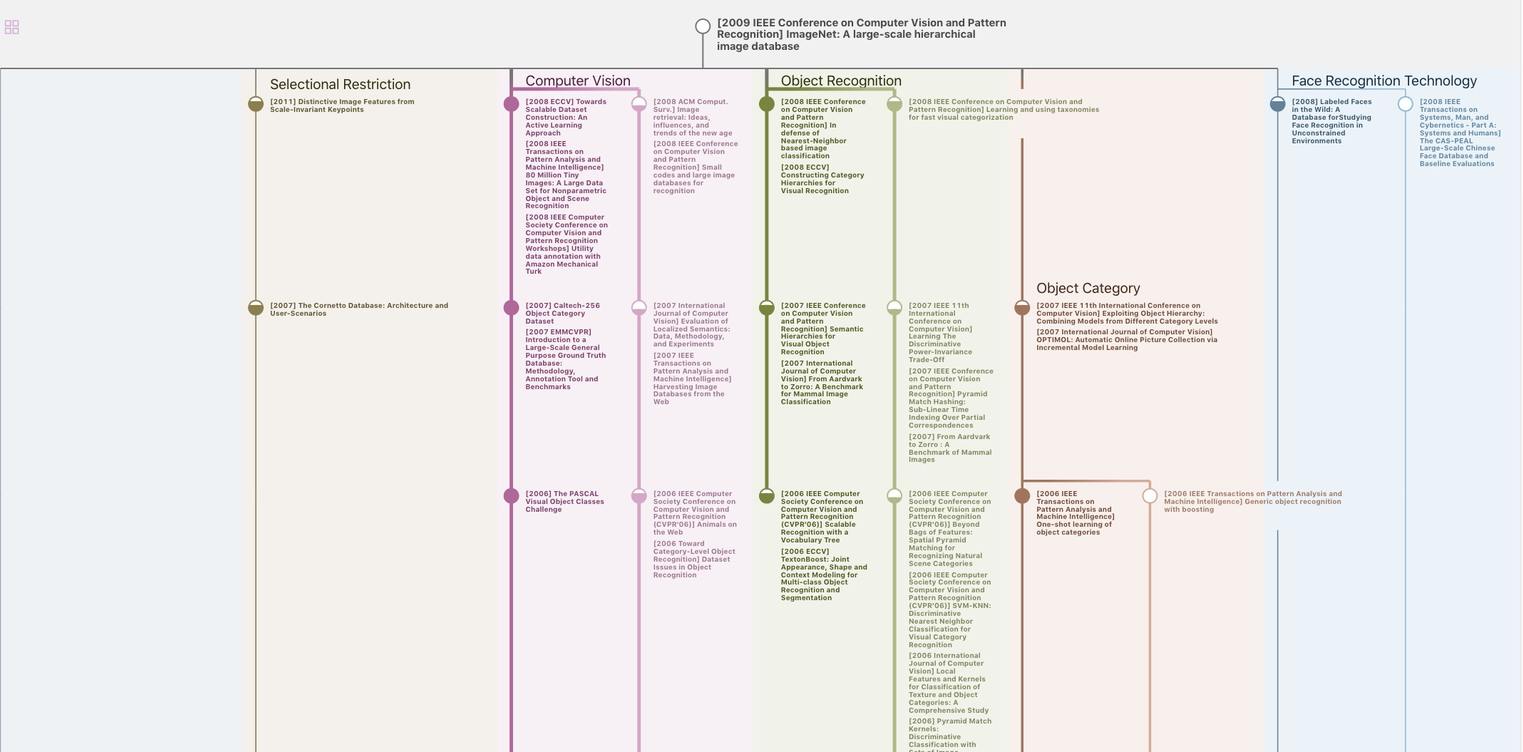
生成溯源树,研究论文发展脉络
Chat Paper
正在生成论文摘要