Random walk with restart on hypergraphs: fast computation and an application to anomaly detection
Data Mining and Knowledge Discovery(2023)
摘要
Random walk with restart (RWR) is a widely-used measure of node similarity in graphs, and it has proved useful for ranking, community detection, link prediction, anomaly detection, etc. Since RWR is typically required to be computed separately for a larger number of query nodes or even for all nodes, fast computation of it is indispensable. However, for hypergraphs, the fast computation of RWR has been unexplored, despite its great potential. In this paper, we propose ARCHER , a fast computation framework for RWR on hypergraphs. Specifically, we first formally define RWR on hypergraphs, and then we propose two computation methods that compose ARCHER . Since the two methods are complementary (i.e., offering relative advantages on different hypergraphs), we also develop a method for automatic selection between them, which takes a very short time compared to the total running time. Through our extensive experiments on 18 real-world hypergraphs, we demonstrate (a) the speed and space efficiency of ARCHER , (b) the complementary nature of the two computation methods composing ARCHER , (c) the accuracy of its automatic selection method, and (d) its successful application to anomaly detection on hypergraphs.
更多查看译文
关键词
Hypergraph,Random walk with restart,Fast computation,Anomaly detection
AI 理解论文
溯源树
样例
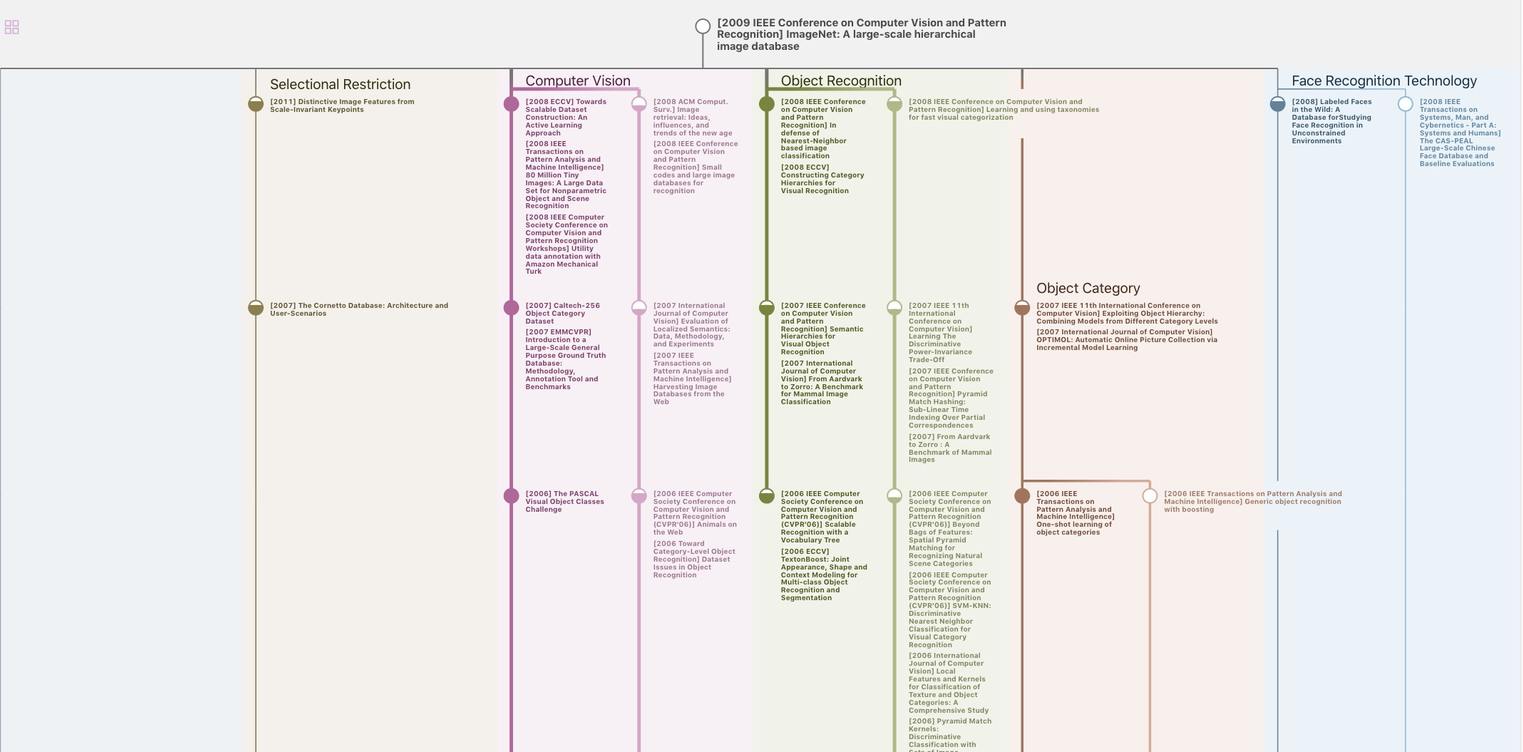
生成溯源树,研究论文发展脉络
Chat Paper
正在生成论文摘要